Fast Adaptive Stacking of Ensembles Adaptation for Supporting Active Learning. a Real Case Application.
ICNC-FSKD(2018)
摘要
Algorithms for mining stream data have received a lot of attention in the last decade. In general, several of these algorithms require labeled instances for training because they are designed for supervised learning. However, in many real activities, labelled process involves overspending of resources. Due to this, active learning and other learning paradigms have proposed reducing the cost of labeling instances without a significant loss of model performance. In this paper we adapt Fast Adaptive Stacking of Ensembles (FASE), an ensemble method for learning from non-stationary data streams, to the active learning paradigm. This new version, called FASE-AL, was assessed by classifying the Joinville roads into paved and unpaved roads. The assessment data is emitted by a device placed in the public transport buses. FASE-AL obtained better percentage of correctly classified instances compared with other classical algorithms. The results are shown on a map which can be used by the public transport companies in the preparation of buses maintenance plan and route planning decisions, for instance.
更多查看译文
关键词
Active learning,classification,ensemble,data stream,concept drift
AI 理解论文
溯源树
样例
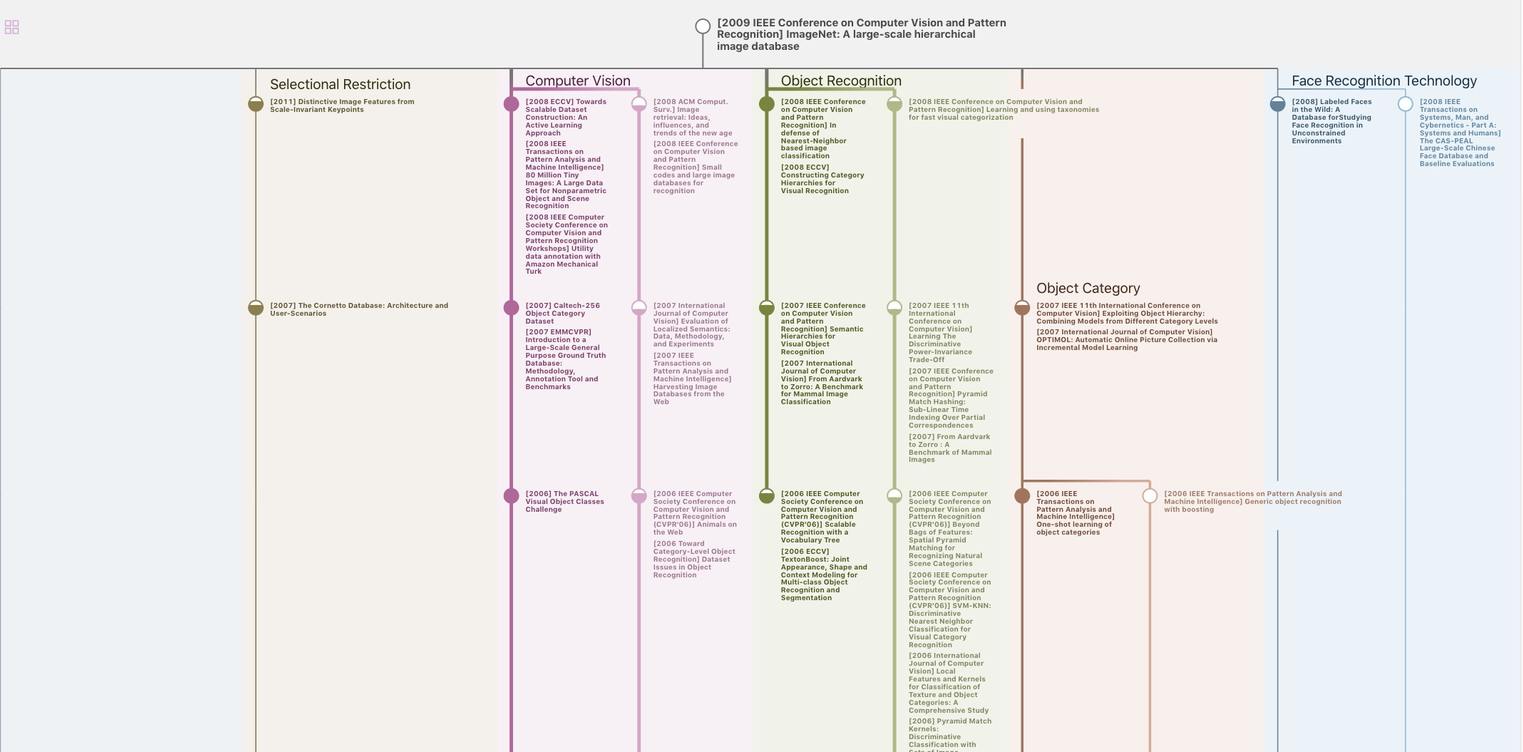
生成溯源树,研究论文发展脉络
Chat Paper
正在生成论文摘要