Hessian-Regularized Multitask Dictionary Learning for Remote Sensing Image Recognition
IEEE Geoscience and Remote Sensing Letters(2019)
摘要
Learning effective image representations is a vital issue for remote sensing (RS) image recognition tasks. Although numerous algorithms have been proposed, it is still challenging due to the limited labeled data. One representative work is the Laplacian-regularized multitask dictionary learning (LR-MTDL) that employs graph Laplacian regularization terms to fully utilize both the labeled and unlabeled information. However, it probably conduces to poor extrapolating power because Laplacian regularization biases the solution toward a constant function. In this letter, we propose a Hessian-regularized multitask dictionary learning to learn a source-data set-shared but target-data set-biased representation for RS image recognition. Particularly, Hessian can properly exploit the intrinsic local geometry of the data manifold and finally leverage the performance. Extensive experiments on four RS image data sets validate the effectiveness of the proposed method by comparing with baseline algorithms including single-task dictionary learning and LR-MTDL.
更多查看译文
关键词
Task analysis,Machine learning,Feature extraction,Laplace equations,Manifolds,Dictionaries,Image recognition
AI 理解论文
溯源树
样例
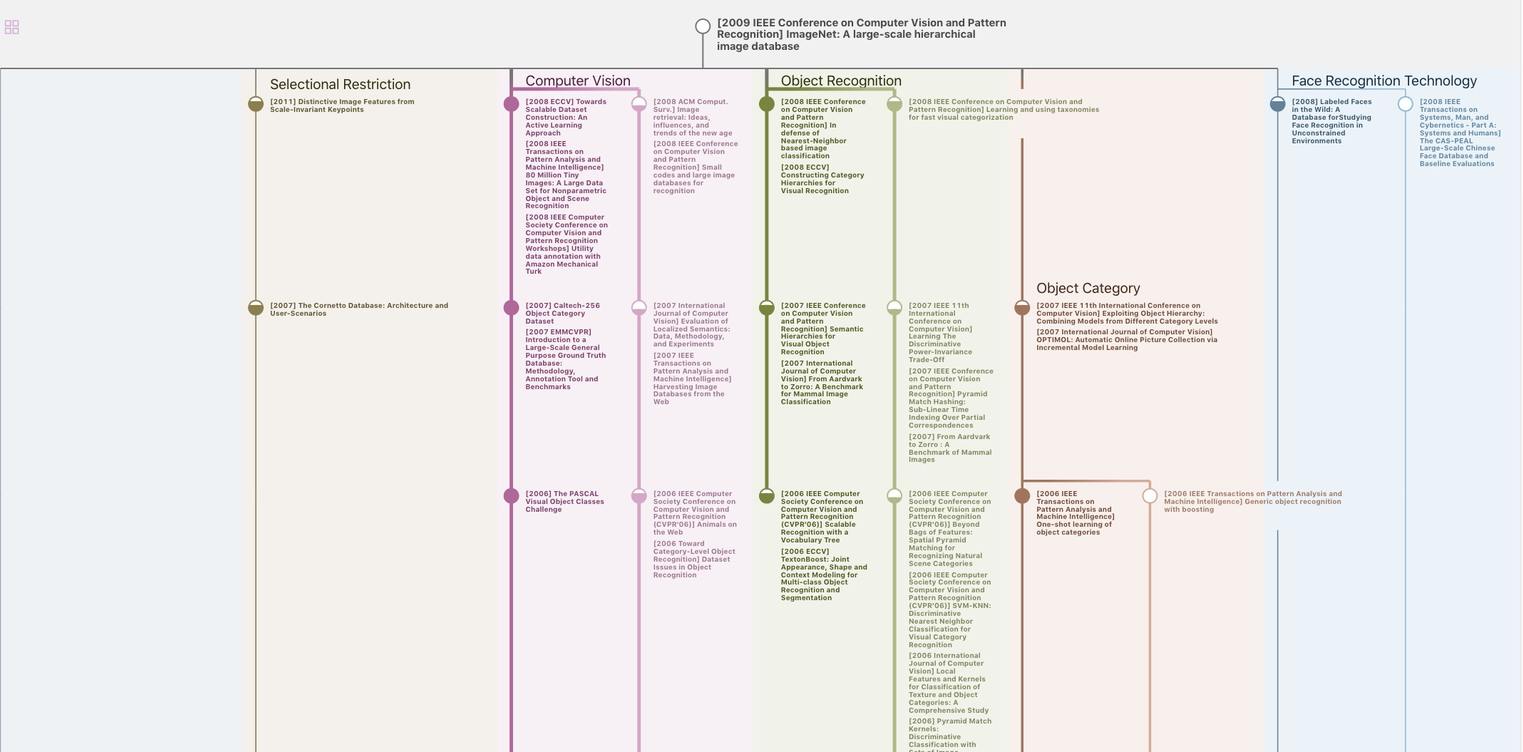
生成溯源树,研究论文发展脉络
Chat Paper
正在生成论文摘要