Controller exploitation-exploration reinforcement learning architecture for computing near-optimal policies
Soft Computing(2018)
摘要
This paper suggests a new controller exploitation-exploration (CEE) reinforcement learning (RL) architecture that attains a near-optimal policy. The proposed architecture consists of three modules: controller, fast-tracked learning and the actor-critic. The strategies are represented by a probability distribution c_ik . The controller employs a combination (balance) of the exploration or exploitation using the Kullback–Leibler divergence deciding if the new strategies are better than currently employed immediate strategy. The exploitation uses a fast-tracked learning algorithm, which employs a fix strategy and priori knowledge. The method is (only) asked to find estimated values of the transition matrices and utilities. The exploration employs an actor-critic architecture. The actor is responsible for the computation of the strategies using a policy gradient method. The critic determines the acceptance of the proposed strategies. We show the convergence of the proposed algorithms for implementing the architecture. An application example related to inventory shows the effectiveness of the proposed architecture.
更多查看译文
关键词
Reinforcement learning,Architecture,Average cost,Markov chains,Optimization
AI 理解论文
溯源树
样例
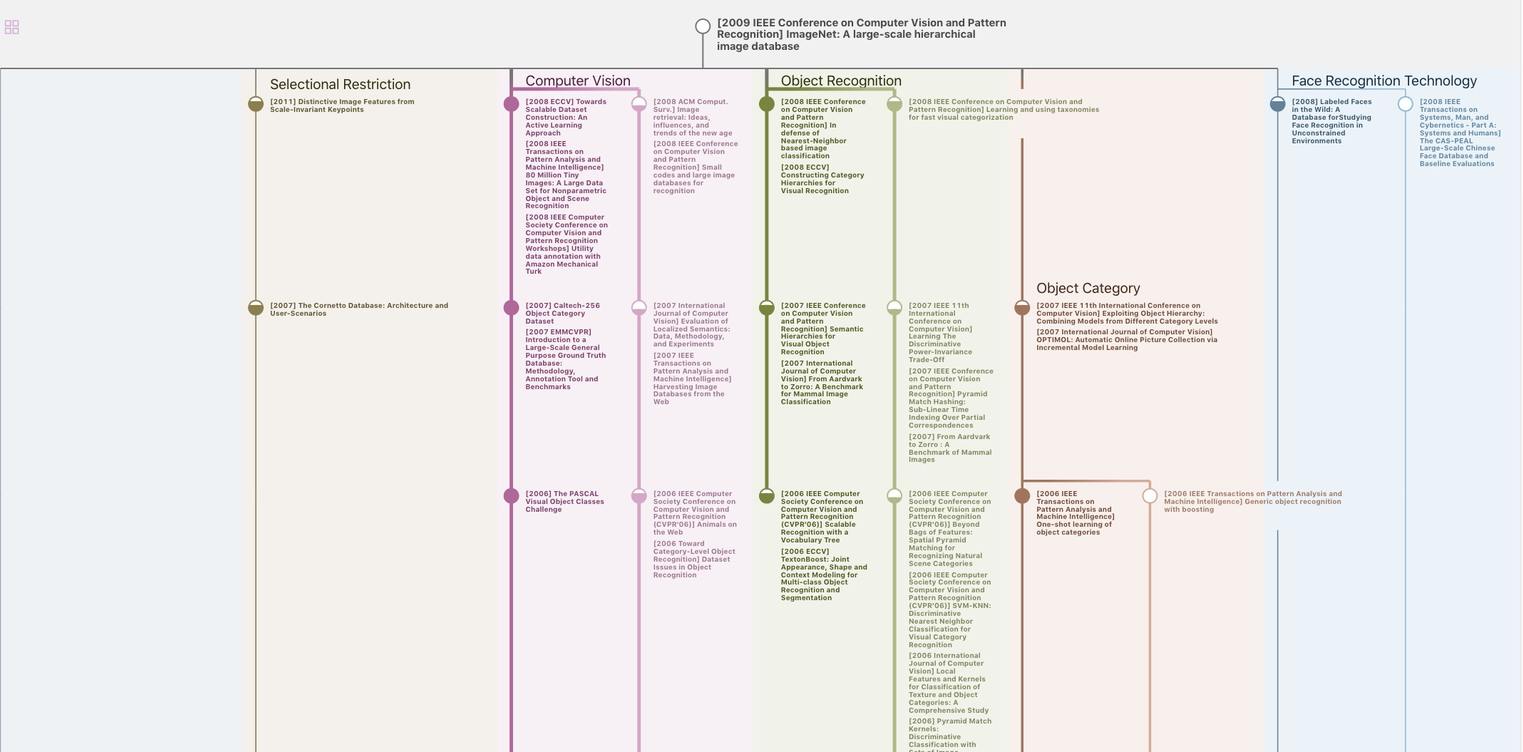
生成溯源树,研究论文发展脉络
Chat Paper
正在生成论文摘要