Improving Differentiable Neural Computers Through Memory Masking, De-allocation, and Link Distribution Sharpness Control
ICLR(2022)
摘要
The Differentiable Neural Computer (DNC) can learn algorithmic and question answering tasks. An analysis of its internal activation patterns reveals three problems: Most importantly, the lack of key-value separation makes the address distribution resulting from content-based look-up noisy and flat, since the value influences the score calculation, although only the key should. Second, DNC's de-allocation of memory results in aliasing, which is a problem for content-based look-up. Thirdly, chaining memory reads with the temporal linkage matrix exponentially degrades the quality of the address distribution. Our proposed fixes of these problems yield improved performance on arithmetic tasks, and also improve the mean error rate on the bAbI question answering dataset by 43%.
更多查看译文
关键词
differentiable neural computers,memory masking,link distribution sharpness control,de-allocation
AI 理解论文
溯源树
样例
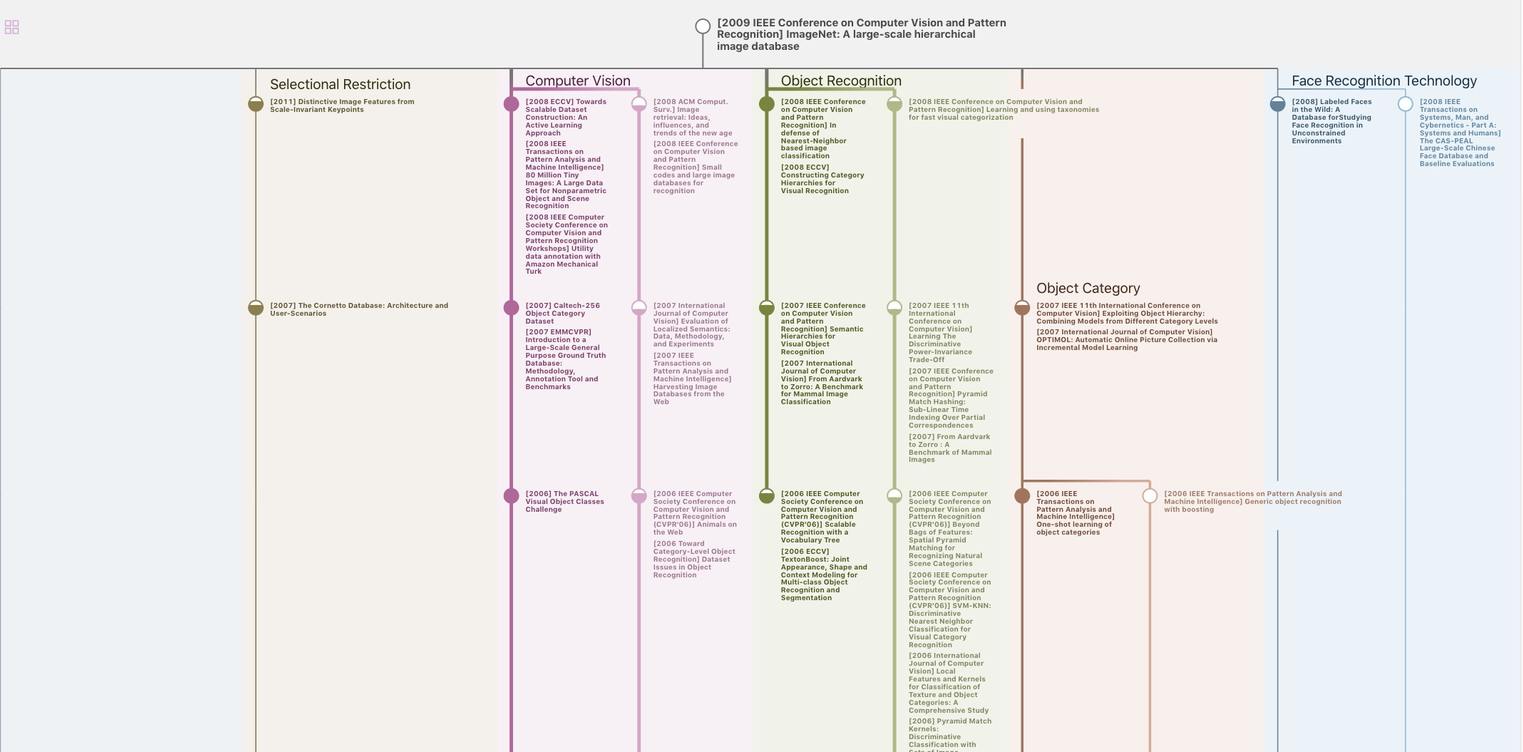
生成溯源树,研究论文发展脉络
Chat Paper
正在生成论文摘要