Self-Supervised Feature Learning With CRF Embedding for Hyperspectral Image Classification
IEEE Transactions on Geoscience and Remote Sensing(2019)
摘要
The challenges in hyperspectral image (HSI) classification lie in the existence of noisy spectral information and lack of contextual information among pixels. Considering the three different levels in HSIs, i.e., subpixel, pixel, and superpixel, offer complementary information, we develop a novel HSI feature learning network (HSINet) to learn consistent features by self-supervision for HSI classification. HSINet contains a three-layer deep neural network and a multifeature convolutional neural network. It automatically extracts the features such as spatial, spectral, color, and boundary as well as context information. To boost the performance of self-supervised feature learning with the likelihood maximization, the conditional random field (CRF) framework is embedded into HSINet. The potential terms of unary, pairwise, and higher order in CRF are constructed by the corresponding subpixel, pixel, and superpixel. Furthermore, the feedback information derived from these terms are also fused into the different-level feature learning process, which makes the HSINet-CRF be a trainable end-to-end deep learning model with the back-propagation algorithm. Comprehensive evaluations are performed on three widely used HSI data sets and our method outperforms the state-of-the-art methods.
更多查看译文
关键词
Feature extraction,Shape,Semantics,Image segmentation,Convolutional neural networks,Hyperspectral sensors
AI 理解论文
溯源树
样例
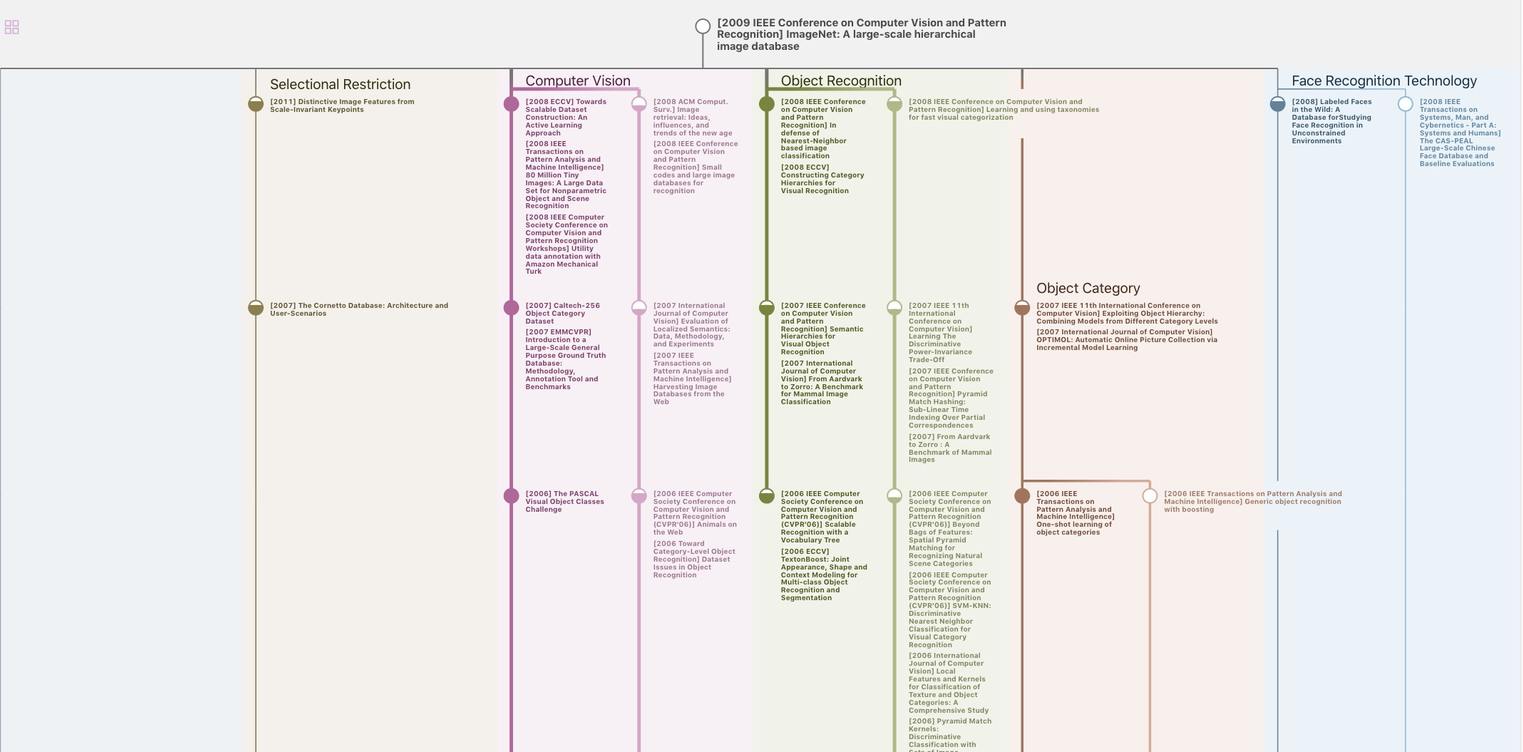
生成溯源树,研究论文发展脉络
Chat Paper
正在生成论文摘要