Distributed Deep Learning-based Offloading for Mobile Edge Computing Networks
Mobile Networks and Applications(2018)
摘要
This paper studies mobile edge computing (MEC) networks where multiple wireless devices (WDs) choose to offload their computation tasks to an edge server. To conserve energy and maintain quality of service for WDs, the optimization of joint offloading decision and bandwidth allocation is formulated as a mixed integer programming problem. However, the problem is computationally limited by the curse of dimensionality, which cannot be solved by general optimization tools in an effective and efficient way, especially for large-scale WDs. In this paper, we propose a distributed deep learning-based offloading (DDLO) algorithm for MEC networks, where multiple parallel DNNs are used to generate offloading decisions. We adopt a shared replay memory to store newly generated offloading decisions which are further to train and improve all DNNs. Extensive numerical results show that the proposed DDLO algorithm can generate near-optimal offloading decisions in less than one second.
更多查看译文
关键词
Mobile edge computing,Offloading,Deep learning,Distributed learning
AI 理解论文
溯源树
样例
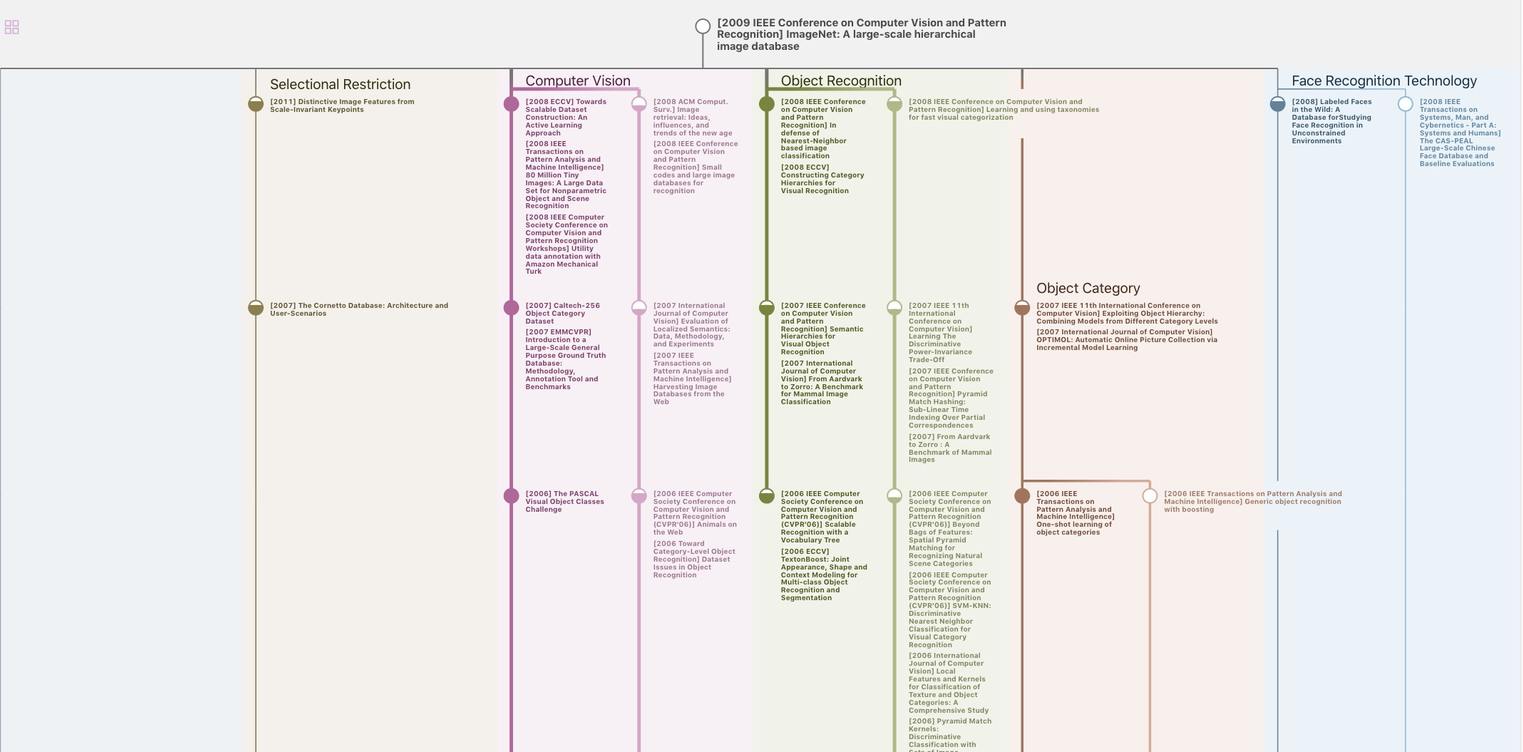
生成溯源树,研究论文发展脉络
Chat Paper
正在生成论文摘要