Outliers Removed via Spectral Clustering for Robust Model Fitting
2018 11th International Symposium on Computational Intelligence and Design (ISCID)(2018)
摘要
This paper proposes a robust model fitting method, called Outliers Removed via Spectral Clustering (ORSC), to estimate multiple inlier structures in the presence of a large number of outliers. The basic idea is to cast each data point to the conceptual space, where the distance distribution of inliers and outliers from the origin is significantly different. Therefore, all the points can be classified into inliers and outliers according to the distribution of points of each subspace, which is obtained by a spectral clustering algorithm. Furthermore, we can use the clustering result to guide the follow-up sampling to get more clean data points for hypotheses generation when handling the complex multi-structure model with a large proportion of outliers. Experimental results show that our method achieves superior performance compared with some state-of-the-art methods in terms of line fitting, circle fitting, and real images.
更多查看译文
关键词
model fitting,spectral clustering,outliers,multi-structure model
AI 理解论文
溯源树
样例
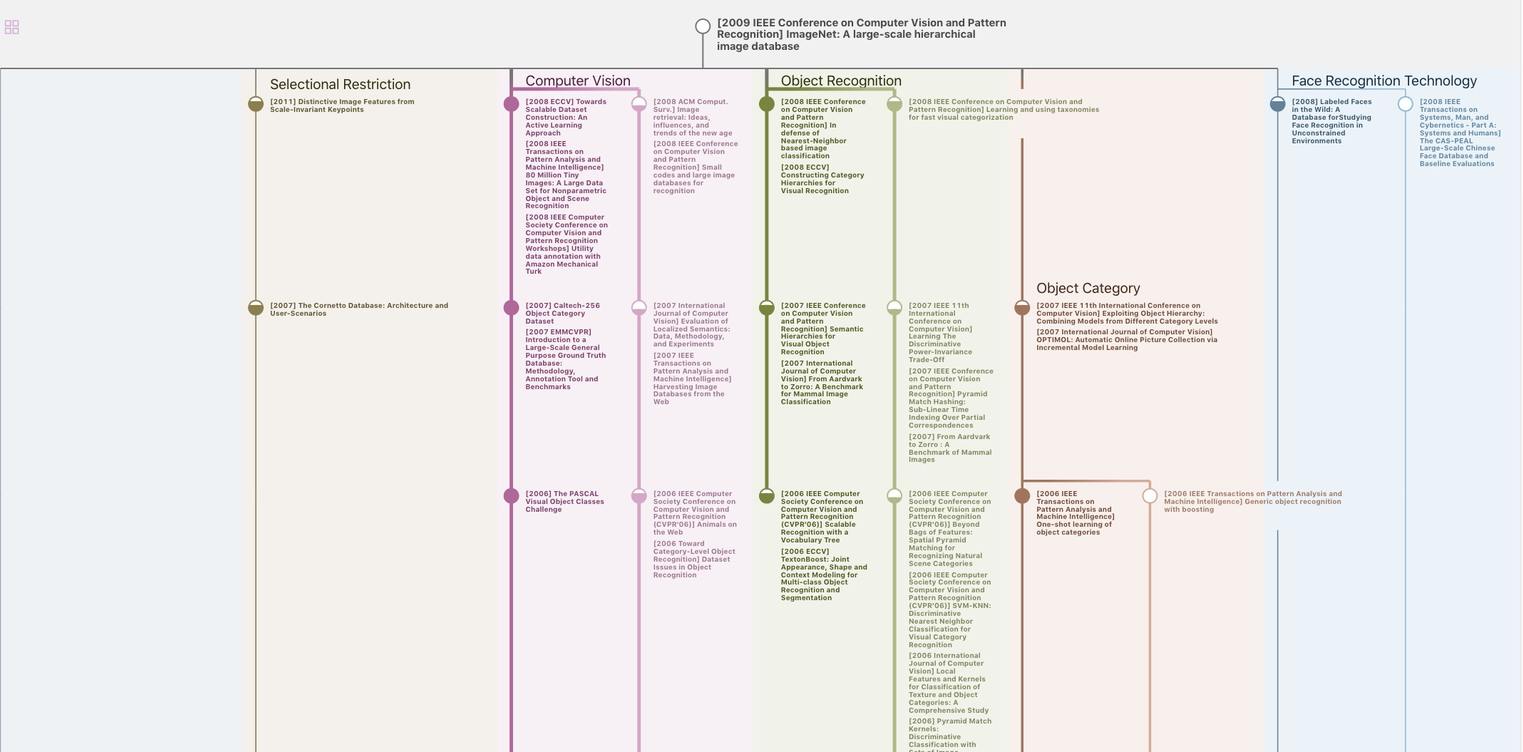
生成溯源树,研究论文发展脉络
Chat Paper
正在生成论文摘要