Self-Guided Hash Coding for Large-Scale Person Re-identification
2019 IEEE Conference on Multimedia Information Processing and Retrieval (MIPR)(2019)
摘要
The laborious manual person ID annotation results in limited training data and increased difficulty in learning discriminative representations. Meanwhile, high dimensional deep features are not ready for fast indexing and matching. Those challenges hinder the application of person Re-Identification (ReID) in large-scale data. To conquer those challenges, we propose a novel training strategy to learn compact binary hash codes. To facilitate feature learning, person images are decomposed into body parts, which are then composed across images into new positive and negative training samples. Binary code quality restrictions are also applied the during training procedure. Requiring no extra annotation costs, our algorithm iteratively generates hard training samples by itself and makes discriminative hash code learning with a limited number of labeled data possible. We hence use "self-guided" to describe this training procedure. Extensive experiments are conducted on two large-scale person ReID datasets, i.e., Market1501 and MSMT17 with distractors, showing our method is competitive compared with recent works.
更多查看译文
关键词
Person ReID,Convolutional Neural Network,Hash Coding
AI 理解论文
溯源树
样例
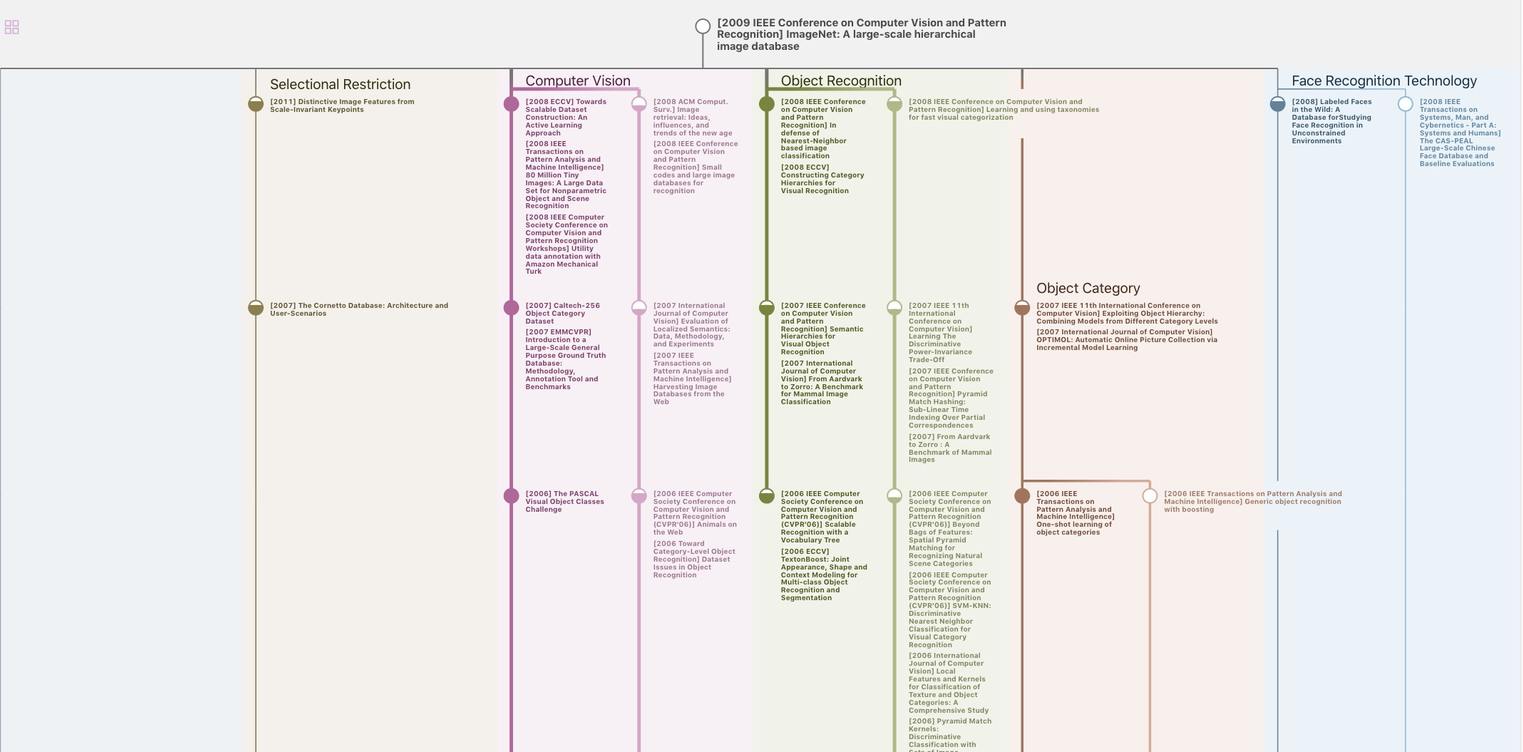
生成溯源树,研究论文发展脉络
Chat Paper
正在生成论文摘要