Convolutional Neural Network-Based Question Answering Over Knowledge Base with Type Constraint.
CCKS(2018)
摘要
We propose a staged framework for question answering over a largescale structured knowledge base. Following existing methods based on semantic parsing, our method relies on various components for solving different sub-tasks of the problem. In the first stage, we directly use the result of entity linking to obtain the topic entity in a question, and simplify the process as a semantic matching problem. We train a neural network to match questions and predicate sequences to get a rough set of candidate answer entities from the knowledge base. Unlike traditional methods, we also consider entity type as a constraint on candidate answers to remove wrong candidates from the rough set in the second stage. By applying a convolutional neural network model to match questions and predicate sequences and a type constraint to filter candidate answers, our method achieves an average F1 measure of 74.8% on the WEBQUESTIONSSP dataset, it is competitive with state-of-the-art semantic parsing approaches.
更多查看译文
关键词
Question answering, Type constraint, Convolutional neural network, Knowledge base, Semantic parsing
AI 理解论文
溯源树
样例
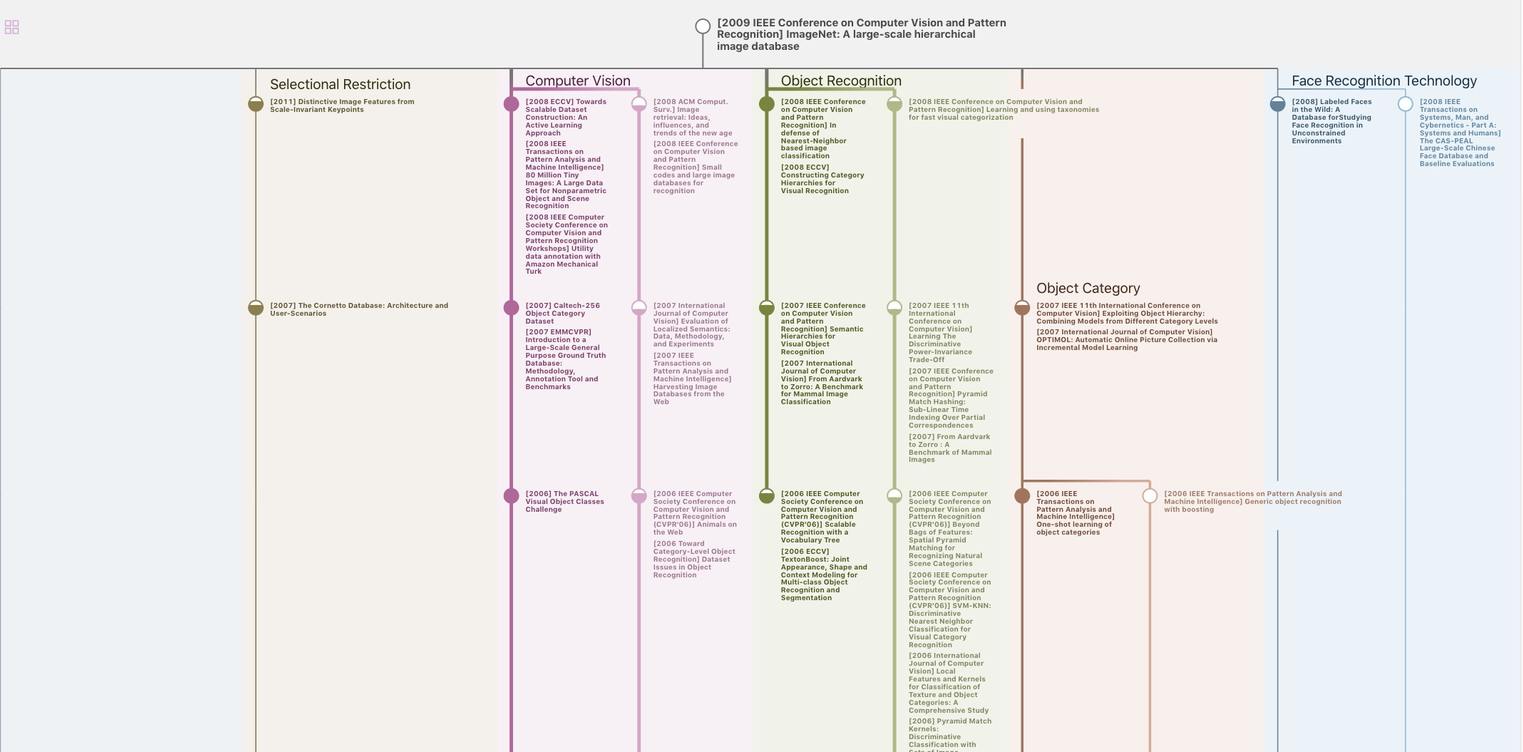
生成溯源树,研究论文发展脉络
Chat Paper
正在生成论文摘要