Stream-suitable optimization algorithms for some soft-margin support vector machine variants
Japanese Journal of Statistics and Data Science(2018)
摘要
Soft-margin support vector machines (SVMs) are an important class of classification models that are well known to be highly accurate in a variety of settings and over many applications. The training of SVMs usually requires that the data be available all at once, in batch. The Stochastic majorization–minimization (SMM) algorithm framework allows for the training of SVMs on streamed data instead. We utilize the SMM framework to construct algorithms for training hinge loss, squared-hinge loss, and logistic loss SVMs. We prove that our three SMM algorithms are each convergent and demonstrate that the algorithms are comparable to some state-of-the-art SVM-training methods. An application to the famous MNIST data set is used to demonstrate the potential of our algorithms.
更多查看译文
关键词
Big data, MNIST, Stochastic majorization–minimization algorithm, Streamed data, Support vector machines
AI 理解论文
溯源树
样例
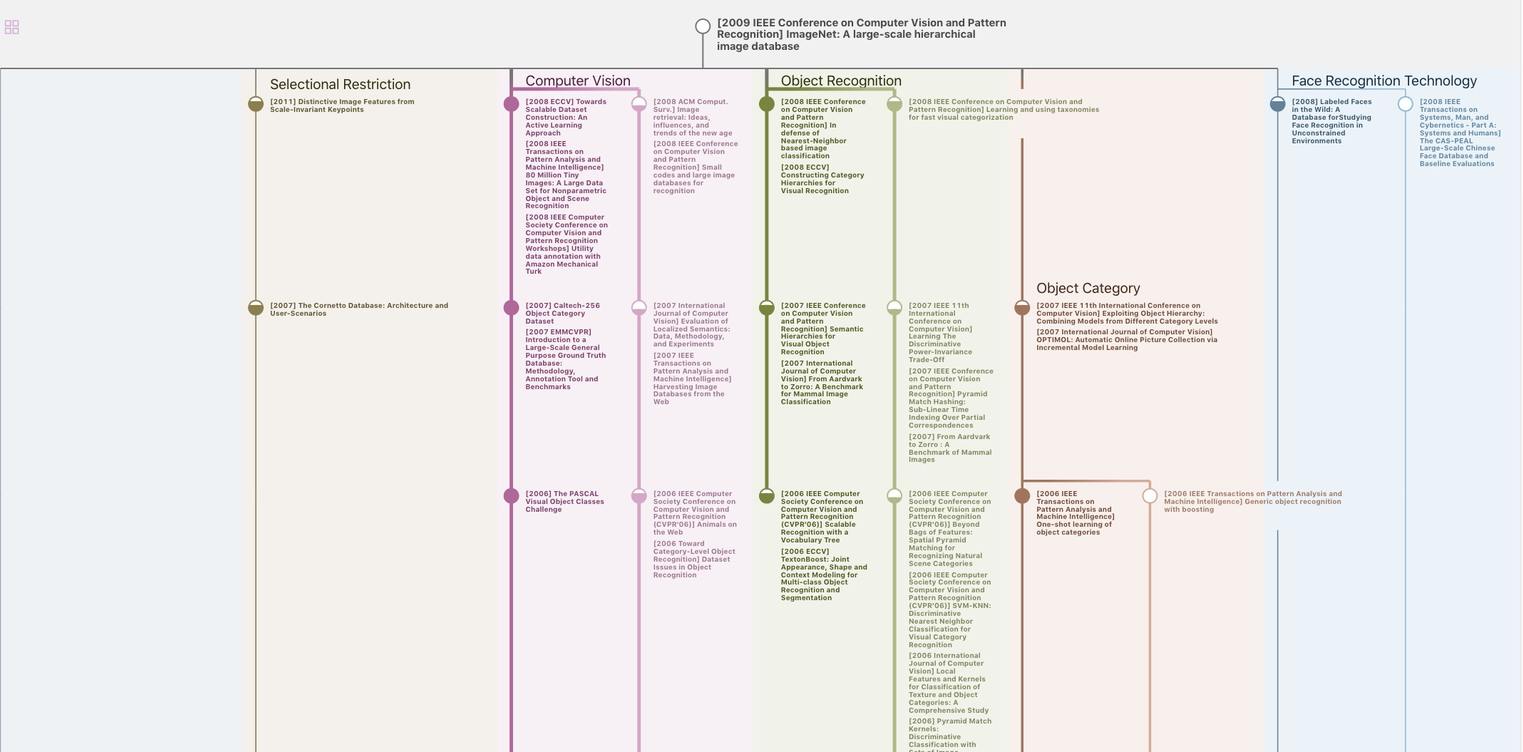
生成溯源树,研究论文发展脉络
Chat Paper
正在生成论文摘要