Semantic Segmentation of Geometric Primitives in Dense 3D Point Clouds
2018 IEEE International Symposium on Mixed and Augmented Reality Adjunct (ISMAR-Adjunct)(2018)
摘要
This paper presents an approach to semantic segmentation and structural modeling from dense 3D point clouds. The core contribution is an efficient method for fitting of geometric primitives based on machine learning. First, the dense 3D point cloud is acquired together with RGB images on a mobile handheld device. Then, RANSAC is used to estimate the presence of geometric primitives, followed by an evaluation of their fit based on classification of the fitting parameters. Finally, the approach iterates over successive frames to optimize the fitting parameters or replace a detected primitive by a better fitting one. As a result, we obtain a semantic model of the scene consisting of a set of geometric primitives. We evaluate the approach on an extensive set of scenarios and show its plausibility in augmented reality applications.
更多查看译文
关键词
Three-dimensional displays,Semantics,Support vector machines,Augmented reality,Solid modeling,Shape,Real-time systems
AI 理解论文
溯源树
样例
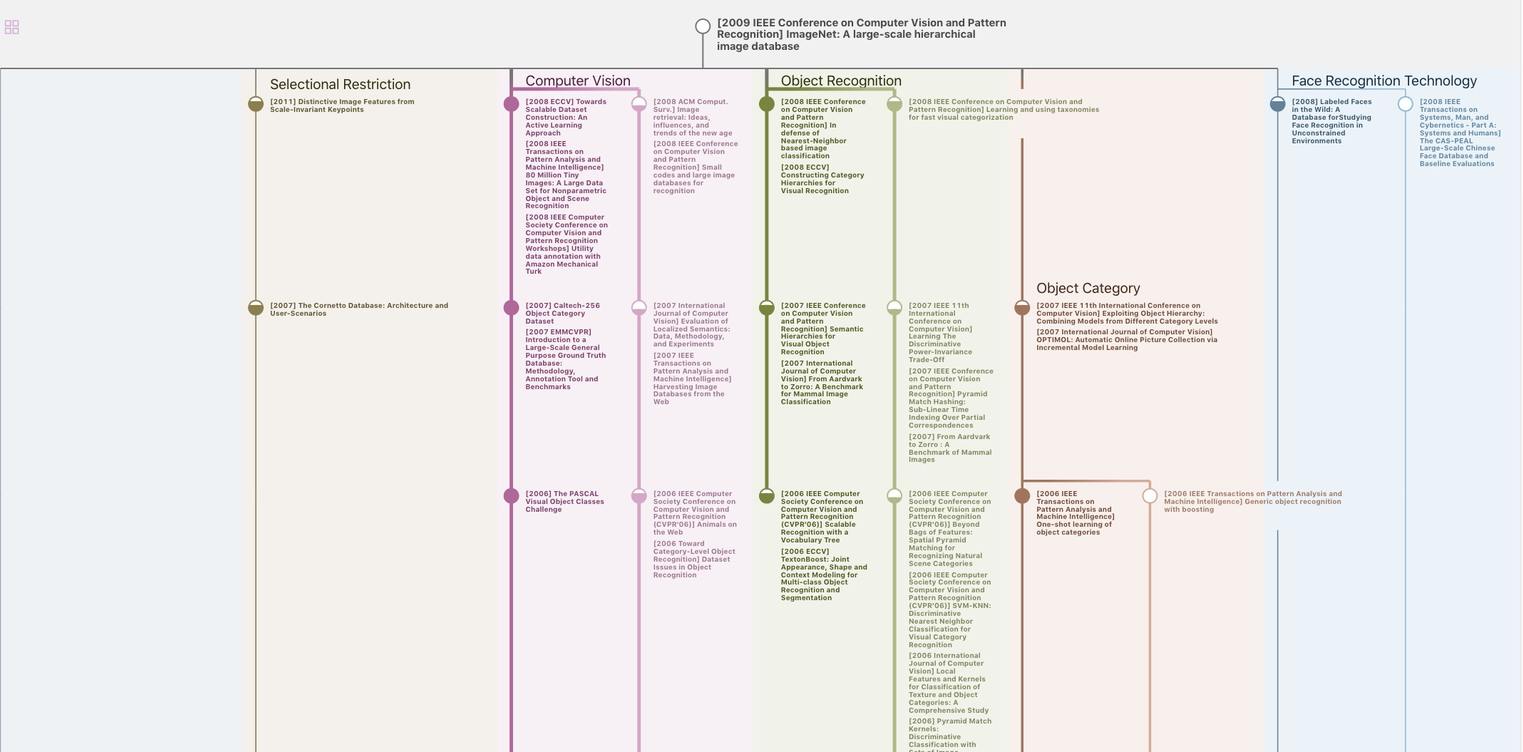
生成溯源树,研究论文发展脉络
Chat Paper
正在生成论文摘要