Driving with Style: Inverse Reinforcement Learning in General-Purpose Planning for Automated Driving
2019 IEEE/RSJ INTERNATIONAL CONFERENCE ON INTELLIGENT ROBOTS AND SYSTEMS (IROS)(2019)
摘要
Behavior and motion planning play an important role in automated driving. Traditionally, behavior planners instruct local motion planners with predefined behaviors. Due to the high scene complexity in urban environments, unpredictable situations may occur in which behavior planners fail to match predefined behavior templates. Recently, general-purpose planners have been introduced, combining behavior and local motion planning. These general-purpose planners allow behavior-aware motion planning given a single reward function. However, two challenges arise: First, this function has to map a complex feature space into rewards. Second, the reward function has to be manually tuned by an expert. Manually tuning this reward function becomes a tedious task. In this paper, we propose an approach that relies on human driving demonstrations to automatically tune reward functions. This study offers important insights into the driving style optimization of general-purpose planners with maximum entropy inverse reinforcement learning. We evaluate our approach based on the expected value difference between learned and demonstrated policies. Furthermore, we compare the similarity of human driven trajectories with optimal policies of our planner under learned and expert-tuned reward functions. Our experiments show that we are able to learn reward functions exceeding the level of manual expert tuning without prior domain knowledge.
更多查看译文
关键词
local motion planning,general-purpose planners,behavior-aware motion planning,single reward function,human driving demonstrations,driving style optimization,maximum entropy inverse reinforcement learning,expert-tuned reward functions,manual expert tuning,general-purpose planning,automated driving,behavior planners,local motion planners,predefined behaviors,high scene complexity,predefined behavior templates
AI 理解论文
溯源树
样例
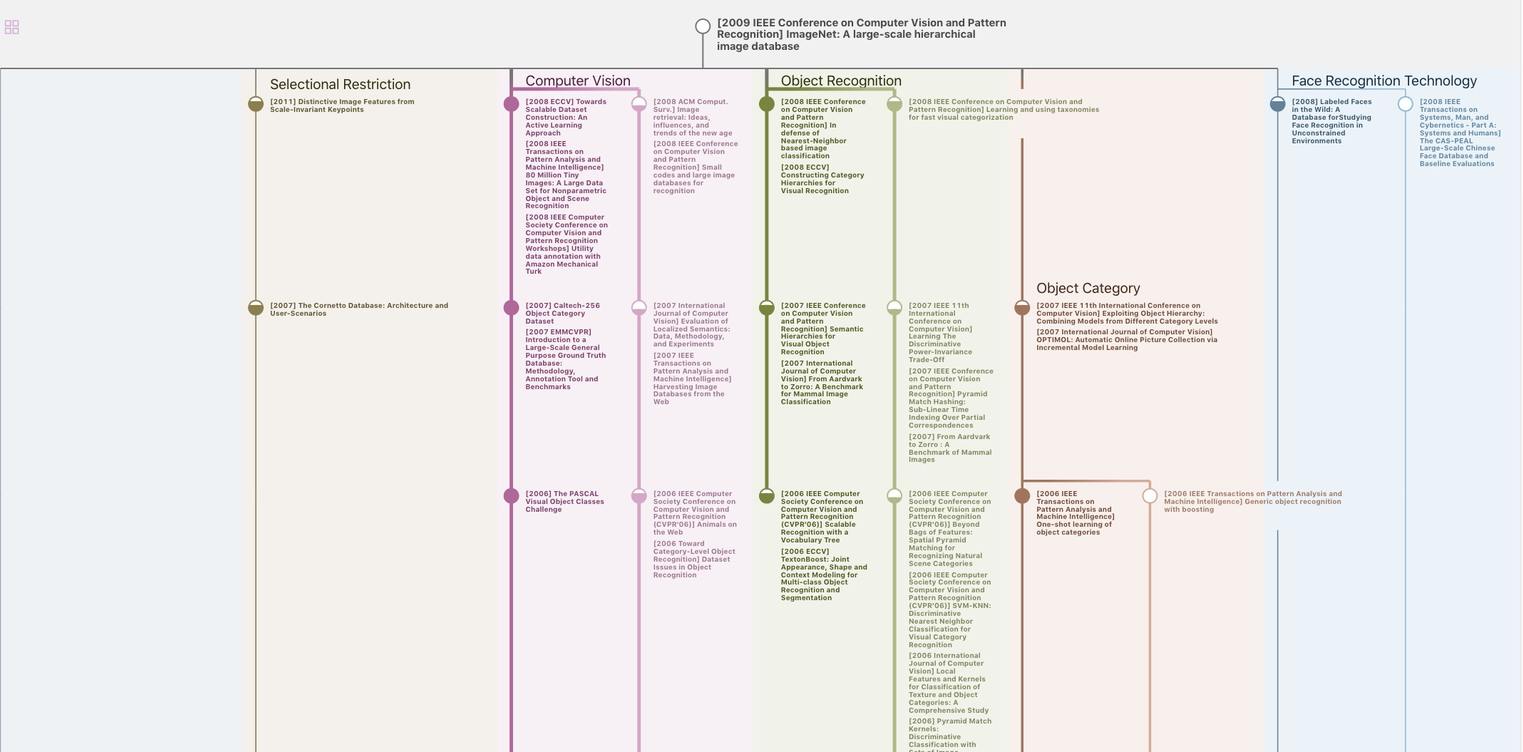
生成溯源树,研究论文发展脉络
Chat Paper
正在生成论文摘要