Physical Activity Change in an RCT: Comparison of Measurement Methods.
American journal of health behavior(2019)
摘要
Objectives: We aimed to quantify the agreement between self-report, standard cut-point accelerometer, and machine learning accelerometer estimates of physical activity (PA), and exam- ine how agreement changes over time among older adults in an intervention setting. Methods: Data were from a randomized weight loss trial that encouraged increased PA among 333 postmenopausal breast cancer survivors. PA was estimated using accelerometry and a validated questionnaire at baseline and 6-months. Accelerometer data were processed using standard cut-points and a validated machine learning algorithm. Agreement of PA at each time-point and change was assessed using mixed effects regression models and concordance correlation. Results: At baseline, self-report and machine learning provided similar PA estimates (mean dif- ference = 11.5 min/day) unlike self-report and standard cut-points (mean difference = 36.3 min/ day). Cut-point and machine learning methods assessed PA change over time more similarly than other comparisons. Specifically, the mean difference of PA change for the cut-point versus machine learning methods was 5.1 min/day for intervention group and 2.9 in controls, whereas it was ≥ 24.7 min/day for other comparisons. Conclusions: Intervention researchers are facing the issue of self-report measures introducing bias and accelerometer cut-points being insensi- tive. Machine learning approaches may bridge this gap.
更多查看译文
关键词
breast cancer,accelerometry,weight loss,intervention,machine learning
AI 理解论文
溯源树
样例
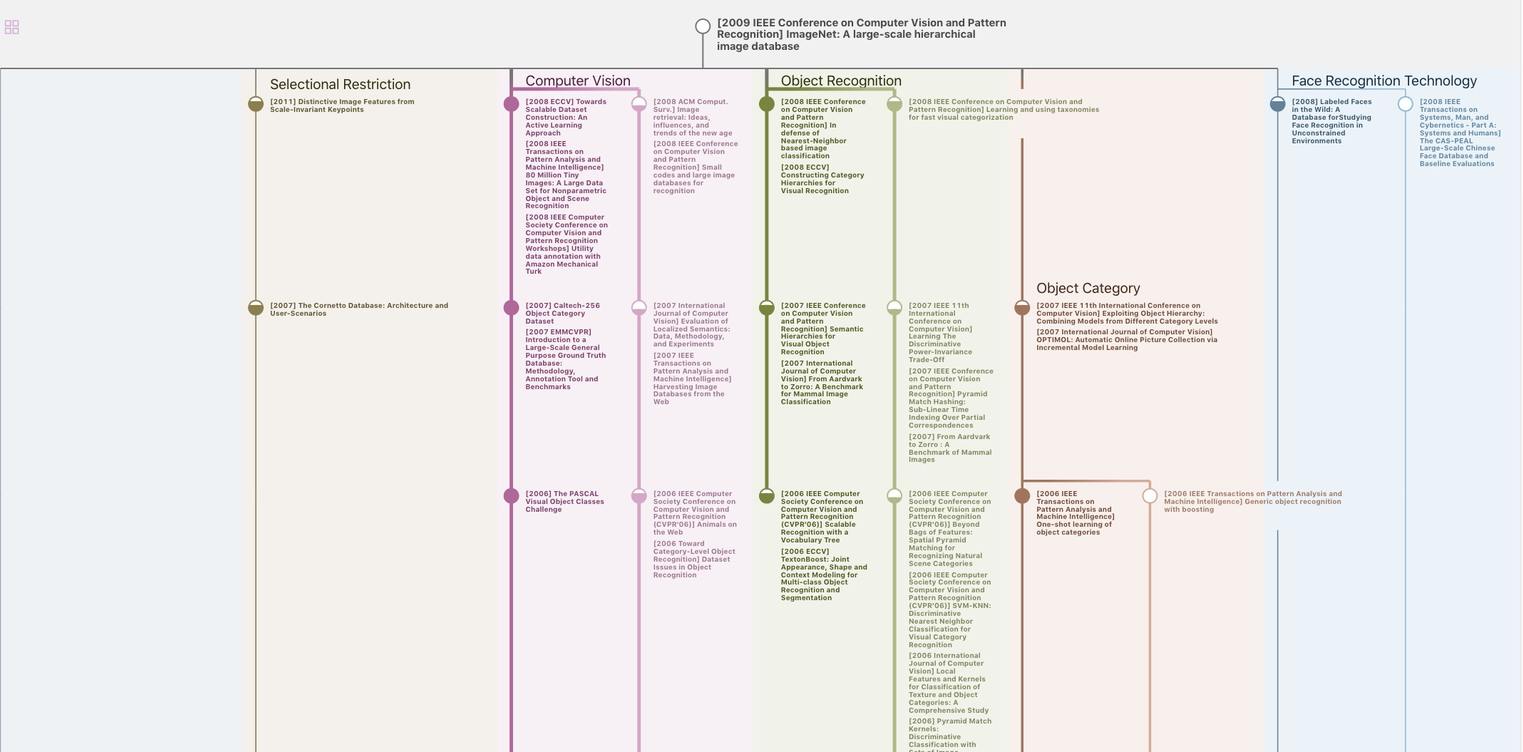
生成溯源树,研究论文发展脉络
Chat Paper
正在生成论文摘要