Stability and Generalization of Graph Convolutional Neural Networks
KDD'19: PROCEEDINGS OF THE 25TH ACM SIGKDD INTERNATIONAL CONFERENCCE ON KNOWLEDGE DISCOVERY AND DATA MINING(2019)
摘要
Inspired by convolutional neural networks on 1D and 2D data, graph convolutional neural networks (GCNNs) have been developed for various learning tasks on graph data, and have shown superior performance on real-world datasets. Despite their success, there is a dearth of theoretical explorations of GCNN models such as their generalization properties. In this paper, we take a first step towards developing a deeper theoretical understanding of GCNN models by analyzing the stability of single-layer GCNN models and deriving their generalization guarantees in a semi-supervised graph learning setting. In particular, we show that the algorithmic stability of a GCNN model depends upon the largest absolute eigenvalue of its graph convolution filter. Moreover, to ensure the uniform stability needed to provide strong generalization guarantees, the largest absolute eigenvalue must be independent of the graph size. Our results shed new insights on the design of new u0026 improved graph convolution filters with guaranteed algorithmic stability. We evaluate the generalization gap and stability on various real-world graph datasets and show that the empirical results indeed support our theoretical findings. To the best of our knowledge, we are the first to study stability bounds on graph learning in a semi-supervised setting and derive generalization bounds for GCNN models.
更多查看译文
关键词
deep learning, generalization guarantees, graph convolutional neural networks, graph mining, stability
AI 理解论文
溯源树
样例
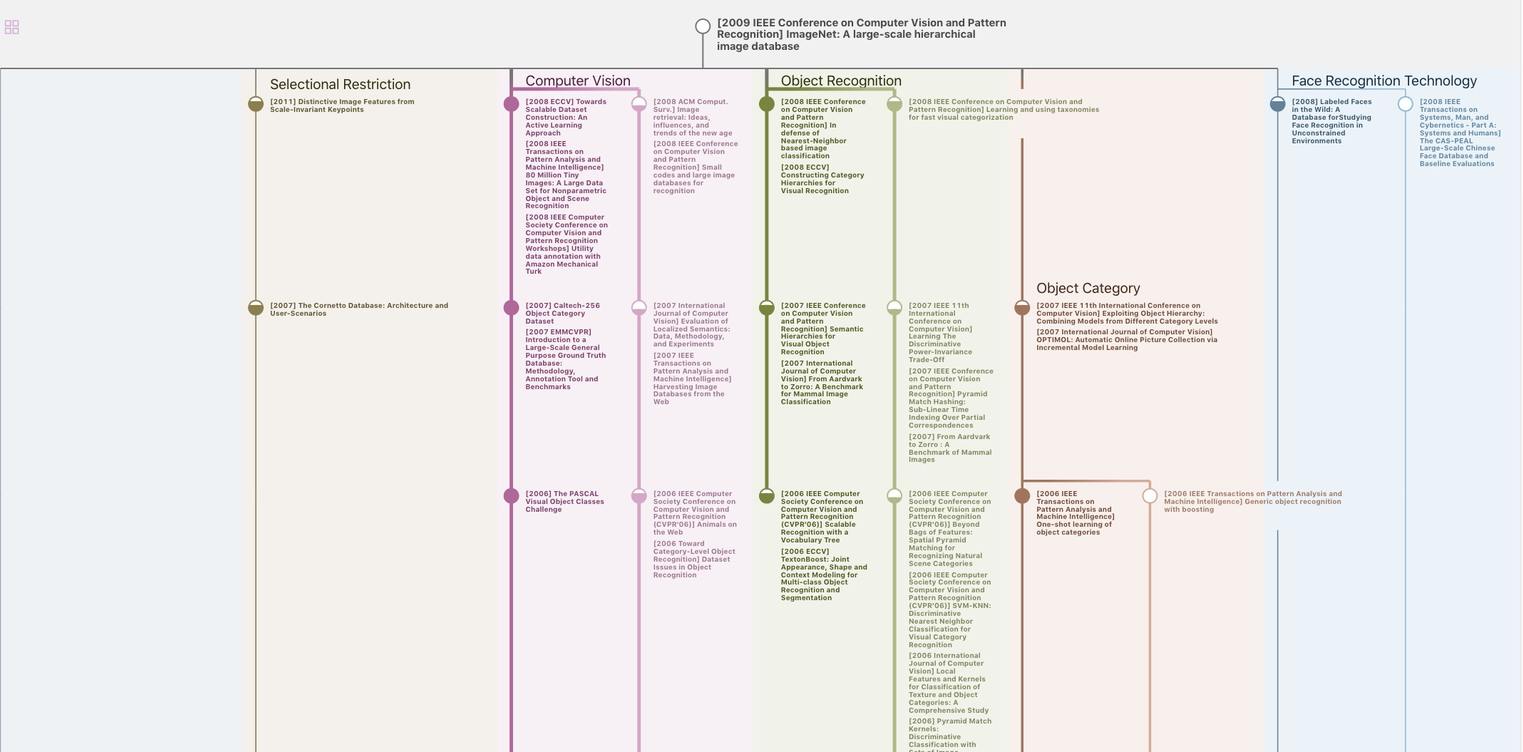
生成溯源树,研究论文发展脉络
Chat Paper
正在生成论文摘要