Knowledge-based Questions Generation with Seq2Seq Learning
2018 IEEE International Conference on Progress in Informatics and Computing (PIC)(2018)
摘要
Building human-computer interaction engines is a fundamental challenge in Artificial Intelligence, with the goal of building a system which can automatically talk to human in natural language. Therefore, the ability to ask thought-provoking questions and contain valuable information in a conversational system is obviously quite crucial and challenging. In this paper, we build a knowledge-based question generation(KBQG) system which can generate natural language questions based on the given knowledge triples to demonstrate how large-scale knowledge base can facilitate language understanding and generation. We propose to apply the neural SEQ2SEQ learning model to generate meaningful questions from the given triples. The encoder reads in triples and produces an answer-aware input representation, which is fed to the decoder to generate an answer-focused question. To be able to express semantics completely and guarantee semantic integrity, we develop a flexible copy-attention mechanism that automatically replicates words from triples when needed. Both automatic and human evaluation results show that our model achieves outstanding performance. Furthermore, we establish a new evaluation metrics called GLAVE(Generated Language’s automatic vector-pooling evaluation) to measure the similarity by pooling of word embeddings instead of word-overlapping information, on account of the diversity and uncertainty of utterances. There are also a series of experiments which can demonstrate the effectiveness of our model is much stronger than state-of-the-art baselines.
更多查看译文
关键词
Decoding,Task analysis,Knowledge based systems,Measurement,Natural languages,Semantics,Computer architecture
AI 理解论文
溯源树
样例
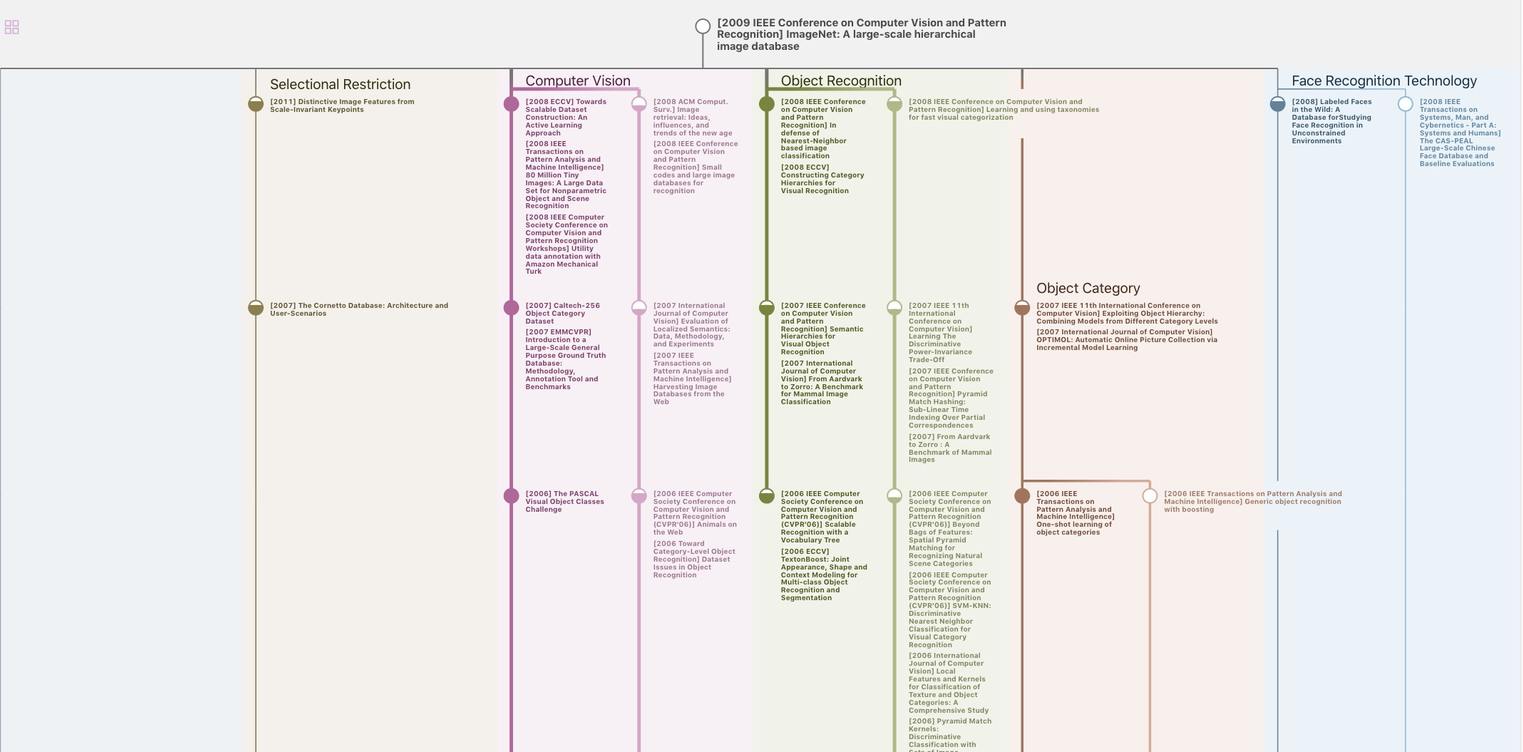
生成溯源树,研究论文发展脉络
Chat Paper
正在生成论文摘要