Fast Communication-Efficient Spectral Clustering over Distributed Data.
IEEE transactions on big data(2021)
摘要
The last decades have seen a surge of interests in distributed computing thanks to advances in clustered computing and big data technology. Existing distributed algorithms typically assume all the data are already in one place, and divide the data and conquer on multiple machines. However, it is increasingly often that the data are located at a number of distributed sites, and one wishes to compute over all the data with low communication overhead. For spectral clustering, we propose a novel framework that enables its computation over such distributed data, with “minimal” communications while a major speedup in computation. The loss in accuracy is negligible compared to the non-distributed setting. Our approach allows local parallel computing at where the data are located, thus turns the distributed nature of the data into a blessing; the speedup is most substantial when the data are evenly distributed across sites. Experiments on synthetic and large UC Irvine datasets show almost no loss in accuracy with our approach while a 2x speedup under various settings with two distributed sites. As the transmitted data need not be in their original form, our framework readily addresses the privacy concern for data sharing in distributed computing.
更多查看译文
关键词
Distributed databases,Big Data,Clustering algorithms,Data mining,Distortion,Business,Spectral clustering,distributed data,data sharing,communication efficient,distortion minimizing local transformation
AI 理解论文
溯源树
样例
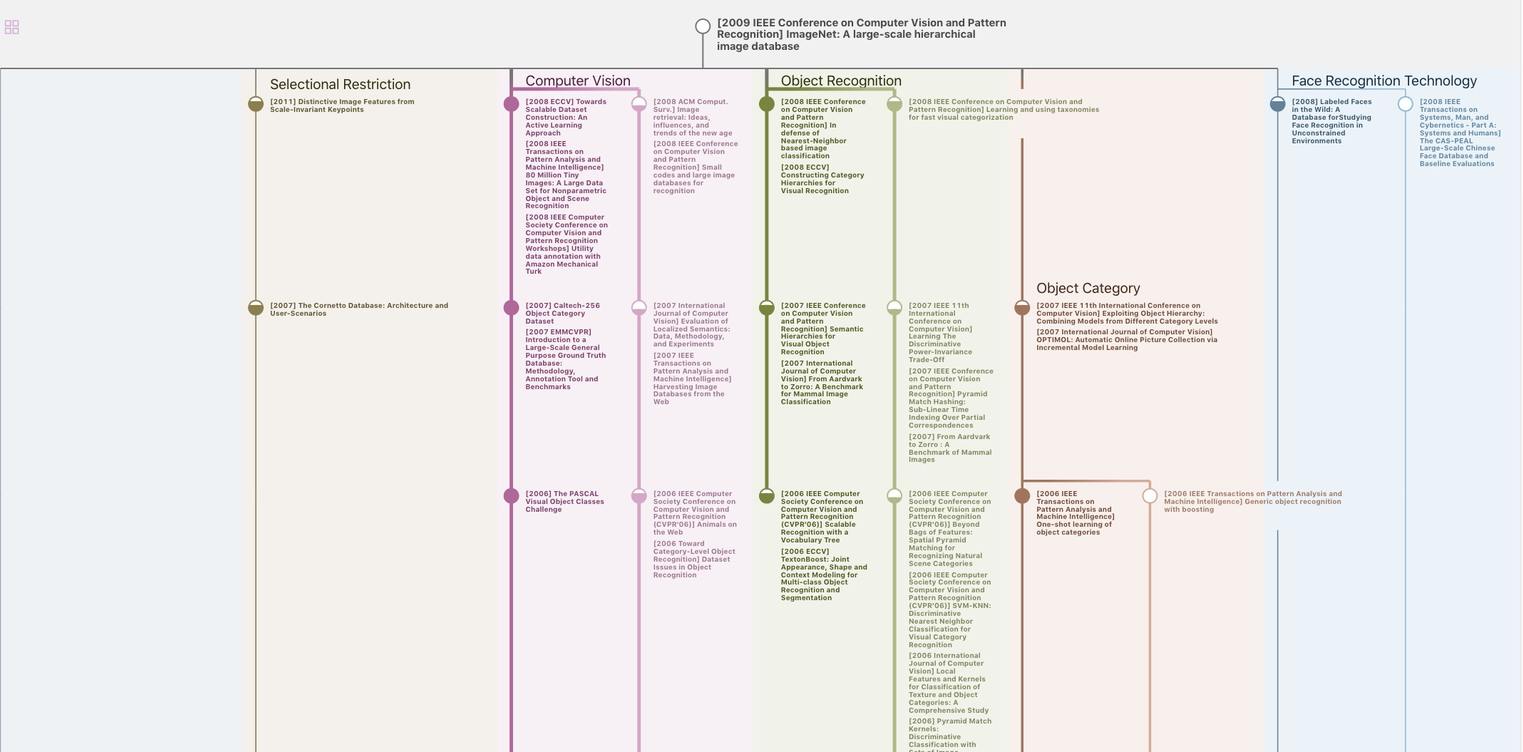
生成溯源树,研究论文发展脉络
Chat Paper
正在生成论文摘要