Multiple Empirical Kernel Learning with Majority Projection for imbalanced problems.
Applied Soft Computing(2019)
摘要
Traditional Multiple Empirical Kernel Learning (MEKL) expands the expressions of the sample and brings better classification ability by using different empirical kernels to map the original data space into multiple kernel spaces. To make MEKL suit for the imbalanced problems, this paper introduces a weight matrix and a regularization term into MEKL. The weight matrix assigns high misclassification cost to the minority samples to balanced misclassification cost between minority and majority class. The regularization term named Majority Projection (MP) is used to make the classification hyperplane fit the distribution shape of majority samples and enlarge the between-class distance of minority and majority class. The contributions of this work are: (i) assigning high cost to minority samples to deal with imbalanced problems, (ii) introducing a new regularization term to concern the property of data distribution, (iii) and modifying the original PAC-Bayes bound to test the error upper bound of MEKL-MP. Through analyzing the experimental results, the proposed MEKL-MP is well suited to the imbalanced problems and has lower generalization risk in accordance with the value of PAC-Bayes bound.
更多查看译文
关键词
Multiple Empirical Kernel Learning,Imbalanced problem,Regularization term,PAC-Bayes bound,Machine learning
AI 理解论文
溯源树
样例
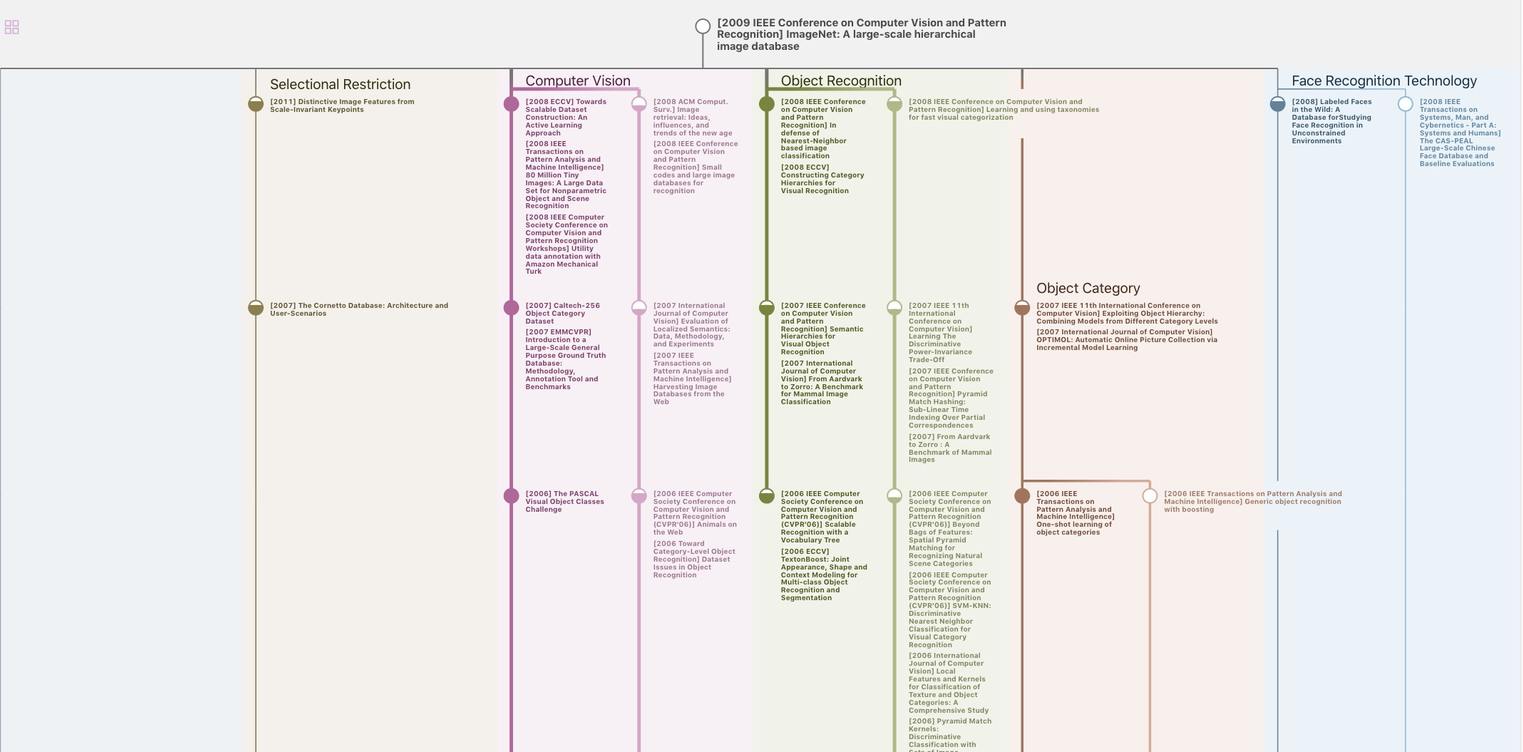
生成溯源树,研究论文发展脉络
Chat Paper
正在生成论文摘要