Ensemble mating selection in evolutionary many-objective search.
Applied Soft Computing(2019)
摘要
Traditional multi-objective evolutionary algorithms have encountered difficulties when handling many-objective problems. This is due to the loss of selection pressure incurred by the growing size of objective space. A variety of environmental selection operators have been proposed to address the issue, each has its distinct benefits and drawbacks. We develop a novel ensemble framework to enhance the effectiveness and robustness of many-objective optimization. The framework incorporates multiple environmental selection operators to guide the search, which are then viewed as voters to construct a mating pool. We design an ensemble mating selection strategy that makes decisions based on the preference information provided by the voters: individuals elected by more voters will be assigned larger possibilities to enter the mating pool. By doing so, high quality offspring can be reproduced from the elected promising candidates. To accommodate the multiple selection operators for voting, the framework maintains multiple parallel populations, where each population is updated by one of the selection operators. An instantiation of the framework with three popular operators is presented as a prime example. Extensive experiments have been conducted on a number of many-objective problems to examine the effectiveness of the proposed approach. Experimental results show that the mating selection strategy is capable of improving the quality of the obtained solution set.
更多查看译文
关键词
Evolutionary algorithm,Mating selection,Multiple parallel populations,Many-objective optimization,Venn diagram
AI 理解论文
溯源树
样例
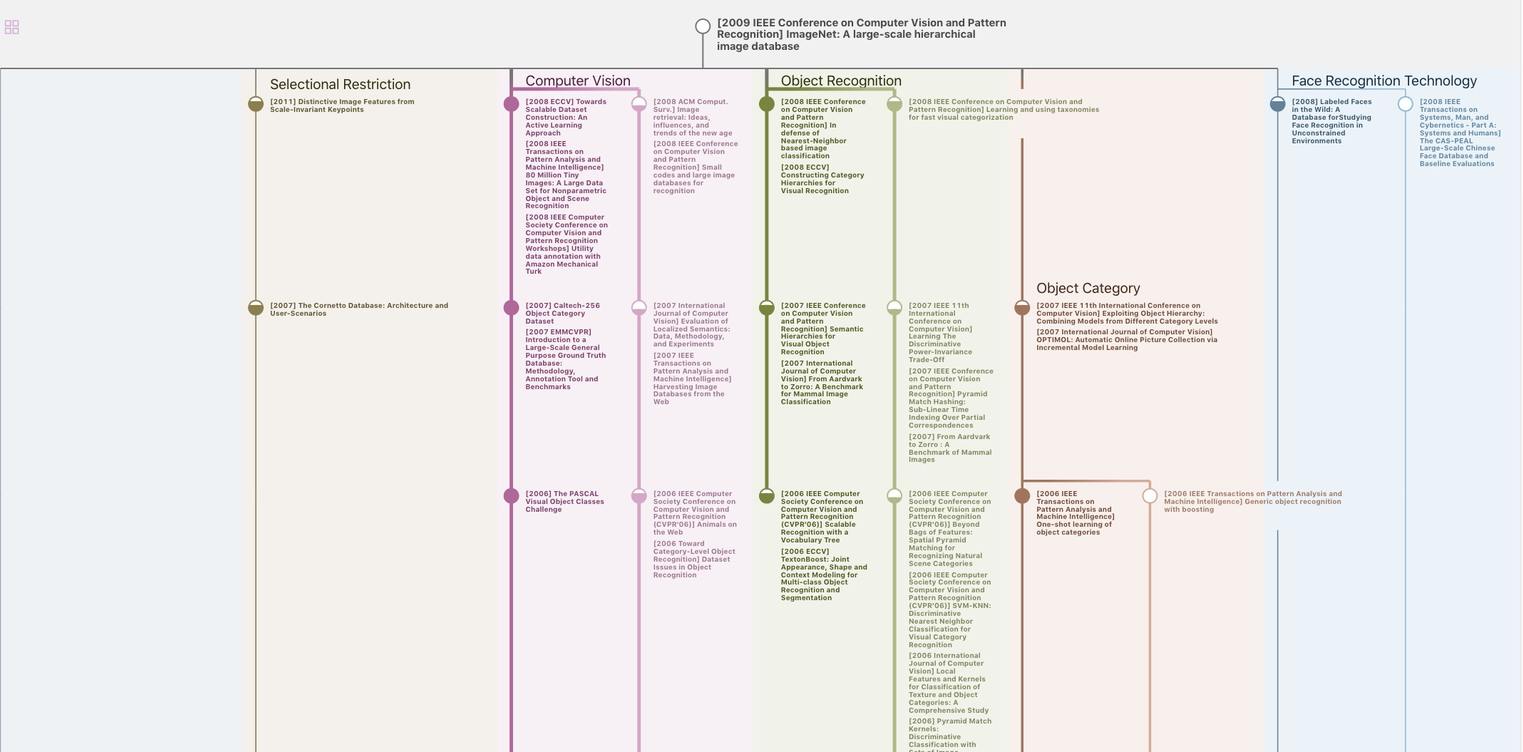
生成溯源树,研究论文发展脉络
Chat Paper
正在生成论文摘要