Fairness-Preserving Text Summarzation
arxiv(2019)
摘要
Given the rapid growth in online information content, text summarization algorithms are progressively used to provide users a succinct idea about the total information content. Historically, summarization algorithms are evaluated only based on how close their outputs are to human-written gold standard summaries. In this work, we propose to evaluate summarization algorithms from an unprecedented viewpoint. Considering that an extractive summarization algorithm selects a subset of the textual units (tweets / sentences) in the input data for inclusion in the summary, we examine the fairness of this selection. Importantly, if the data to be summarized is generated by different socially salient groups, or different political groups, or different news media sources, then we check whether the generated summaries fairly represent these different groups or sources. In real-world datasets we observe that existing summarization algorithms often represent the groups very differently compared to their distributions in the input data. To alleviate such adverse impacts, we propose a novel fair summarization algorithm 'FairSumm' capable of generating high-quality fair summaries.
更多查看译文
AI 理解论文
溯源树
样例
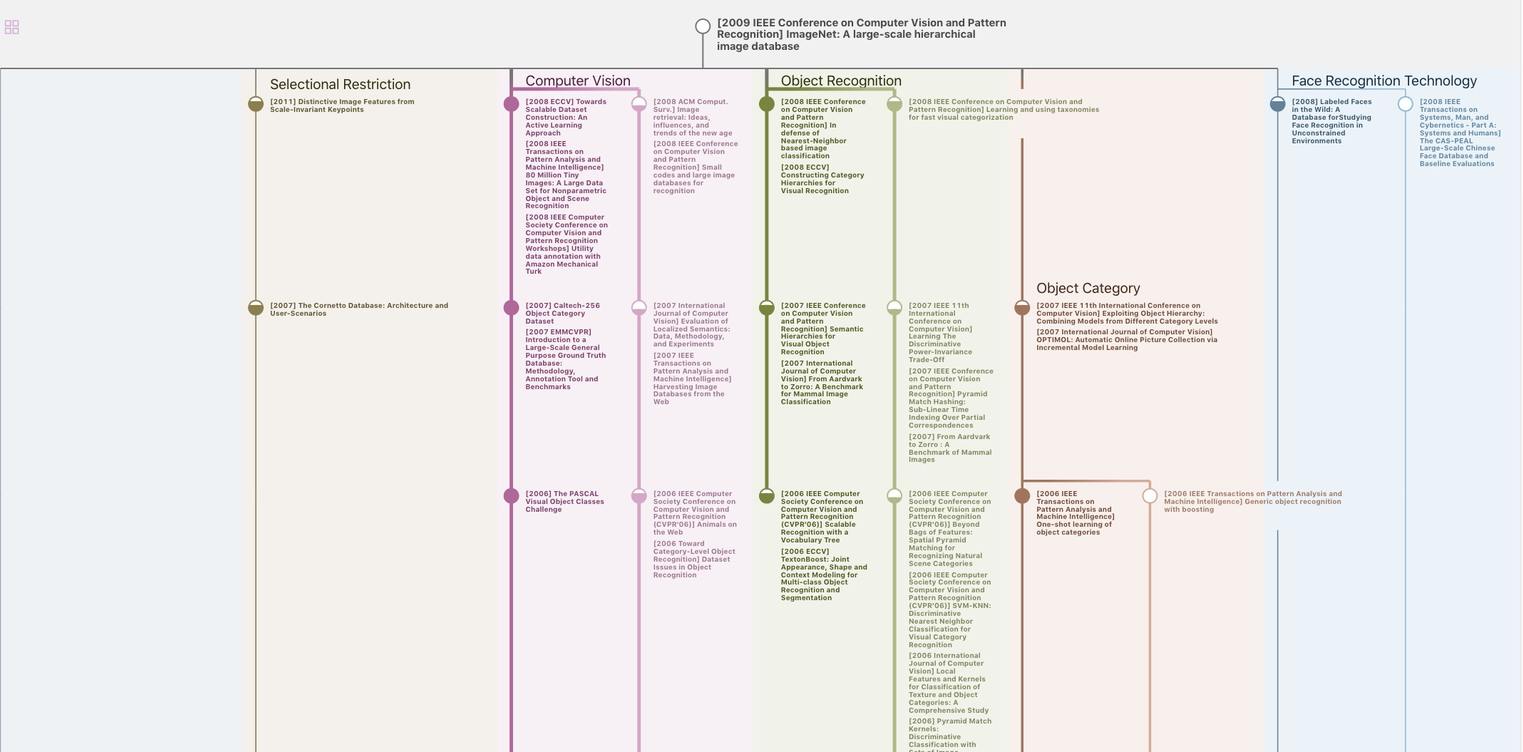
生成溯源树,研究论文发展脉络
Chat Paper
正在生成论文摘要