Prsim: Sublinear Time Simrank Computation On Large Power-Law Graphs
SIGMOD '19: PROCEEDINGS OF THE 2019 INTERNATIONAL CONFERENCE ON MANAGEMENT OF DATA(2019)
摘要
SimRank is a classic measure of the similarities of nodes in a graph. Given a node u in graph G = (V, E), a single-source SimRank query returns the SimRank similarities s (u, v) between node u and each node v. V. This type of queries has numerous applications in web search and social networks analysis, such as link prediction, web mining, and spam detection. Existing methods for single-source SimRank queries, however, incur query cost at least linear to the number of nodes n, which renders them inapplicable for real-time and interactive analysis.This paper proposes PRSim, an algorithm that exploits the structure of graphs to efficiently answer single-source SimRank queries. PRSim uses an index of size O(m), where m is the number of edges in the graph, and guarantees a query time that depends on the reverse PageRank distribution of the input graph. In particular, we prove that PRSim runs in sub-linear time if the degree distribution of the input graph follows the power-law distribution, a property possessed by many real-world graphs. Based on the theoretical analysis, we show that the empirical query time of all existing SimRank algorithms also depends on the reverse PageRank distribution of the graph. Finally, we present the first experimental study that evaluates the absolute errors of various SimRank algorithms on large graphs, and we show that PRSim outperforms the state of the art in terms of query time, accuracy, index size, and scalability.
更多查看译文
关键词
SimRank,Power-Law Graphs,Personalized PageRank
AI 理解论文
溯源树
样例
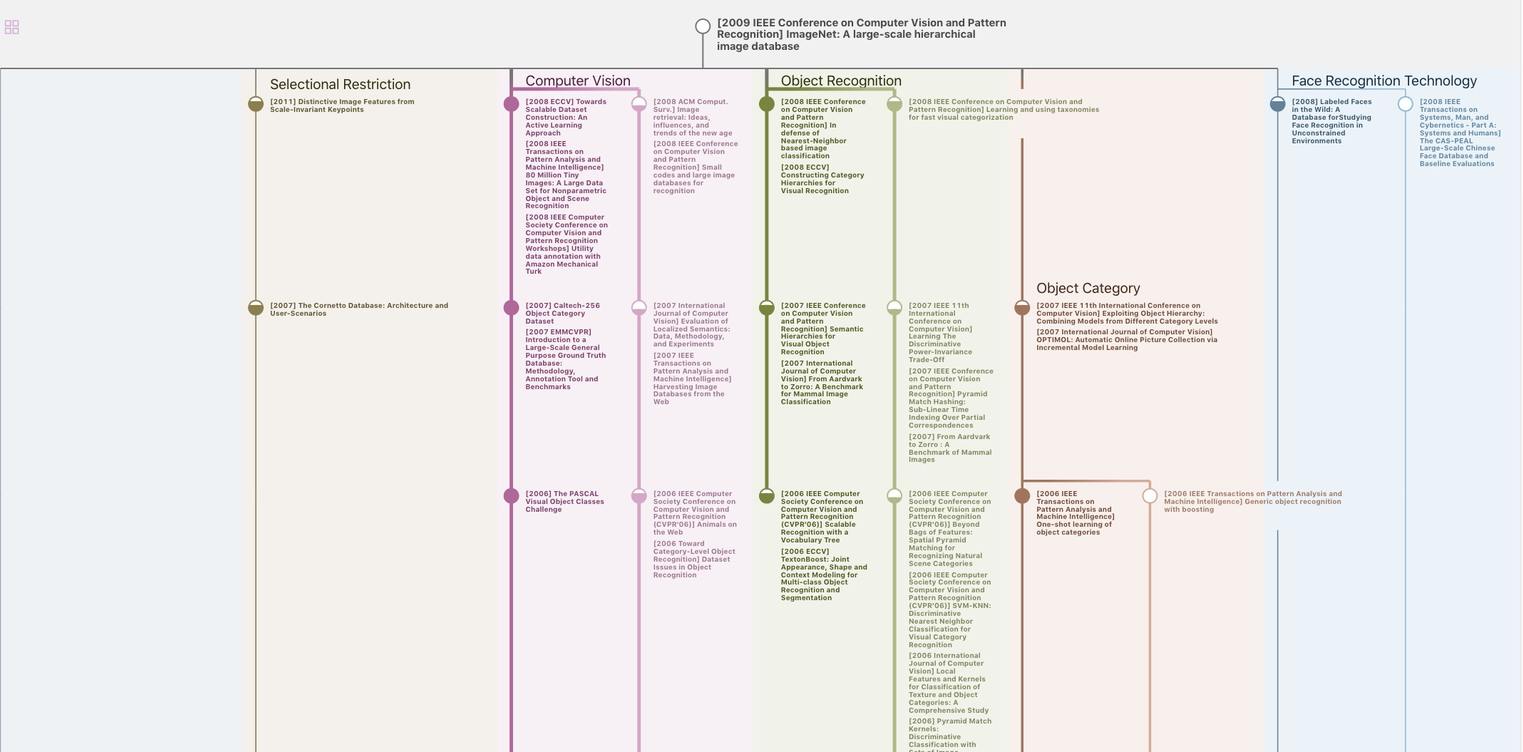
生成溯源树,研究论文发展脉络
Chat Paper
正在生成论文摘要