Strategyproof linear regression in high dimensions: an overview
ACM SIGecom Exchanges(2019)
摘要
In this letter, we outline some of the results from our recent work, which is part of an emerging line of research at the intersection of machine learning and mechanism design aiming to avoid noise in training data by correctly aligning the incentives of data sources. Specifically, we focus on the ubiquitous problem of linear regression, where strategyproof mechanisms have previously been identified in two dimensions. In our setting, agents have single-peaked preferences and can manipulate only their response variables. Our main contribution is the discovery of a family of group strategyproof linear regression mechanisms in any number of dimensions, which we call generalized resistant hyperplane mechanisms. The game-theoretic properties of these mechanisms --- and, in fact, their very existence --- are established through a connection to a discrete version of the Ham Sandwich Theorem.
更多查看译文
关键词
Algorithms, Economics, Theory
AI 理解论文
溯源树
样例
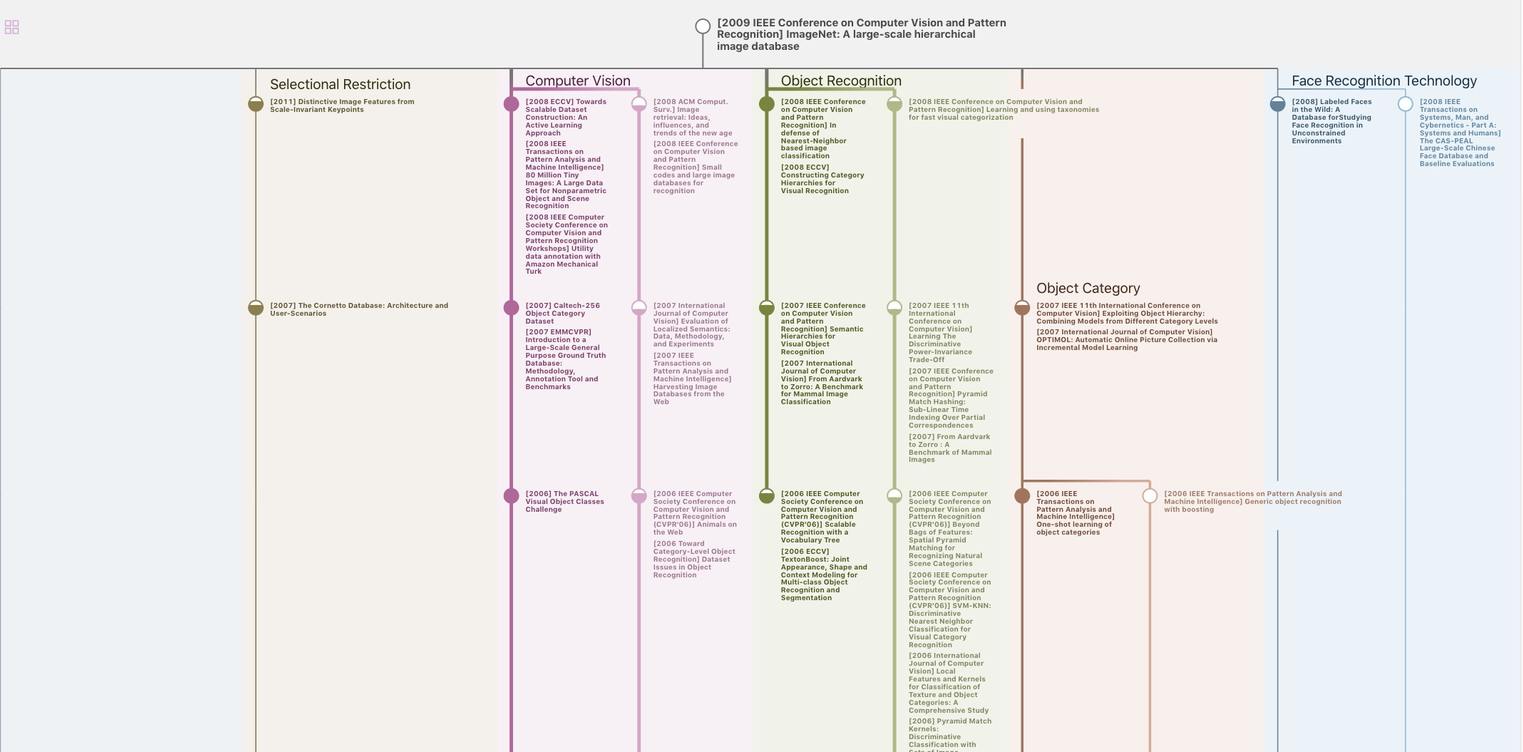
生成溯源树,研究论文发展脉络
Chat Paper
正在生成论文摘要