Flexible non-greedy discriminant subspace feature extraction.
Neural Networks(2019)
摘要
Recently, L1-norm-based non-greedy linear discriminant analysis (NLDA-L1) for feature extraction has been shown to be effective for dimensionality reduction, which obtains projection vectors by a non-greedy algorithm. However, it usually acquires unsatisfactory performances due to the utilization of L1-norm distance measurement. Therefore, in this brief paper, we propose a flexible non-greedy discriminant subspace feature extraction method, which is an extension of NLDA-L1 by maximizing the ratio of Lp-norm inter-class dispersion to intra-class dispersion. Besides, we put forward a powerful iterative algorithm to solve the resulted objective function and also conduct theoretical analysis on the algorithm. Finally, experimental results on image databases show the effectiveness of our method
更多查看译文
关键词
L1-norm-based non-greedy discriminant analysis,Lp-norm inter-class dispersion,Intra-class dispersion,Robust distance measurement
AI 理解论文
溯源树
样例
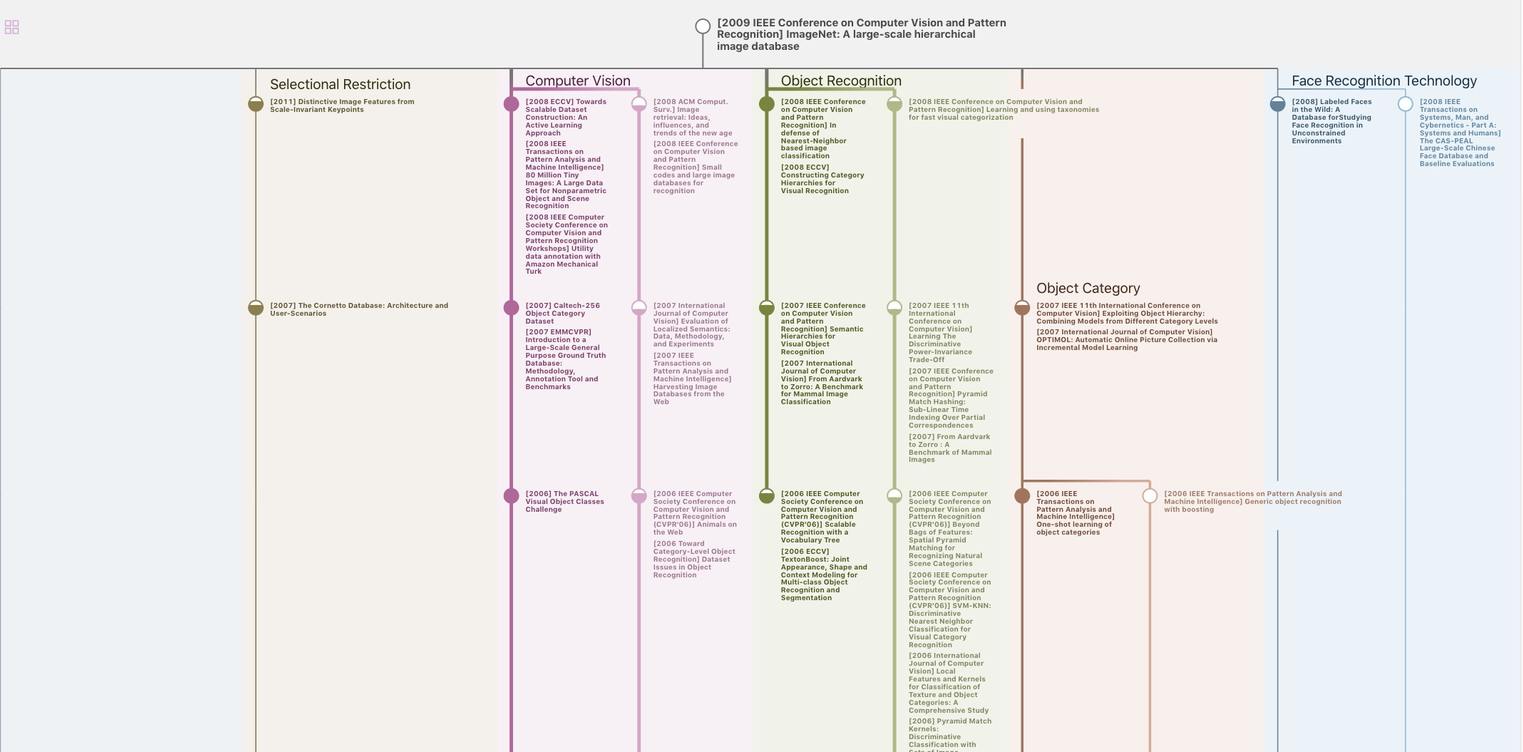
生成溯源树,研究论文发展脉络
Chat Paper
正在生成论文摘要