Learning performance prediction via convolutional GRU and explainable neural networks in e-learning environments
Computing(2019)
摘要
Students learning performance prediction is a challenging task due to the dynamic, virtual environments and the personalized needs for different individuals. To ensure that learners’ potential problems can be identified as early as possible, this paper aim to develop a predictive model for effective learning feature extracting, learning performance predicting and result reasoning. We first proposed a general learning feature quantification method to convert the raw data from e-learning systems into sets of independent learning features. Then, a weighted avg-pooling is chosen instead of typical max-pooling in a novel convolutional GRU network for learning performance prediction. Finally, an improved parallel xNN is provided to explain the prediction results. The relevance of positive/negative between features and result could help students find out which part should be improved. Experiments have been carried out over two real online courses data. Results show that our proposed approach performs favorably compared with several other state-of-the-art methods.
更多查看译文
关键词
Learning performance prediction,Learning feature quantification,Deep neural network,E-learning environments
AI 理解论文
溯源树
样例
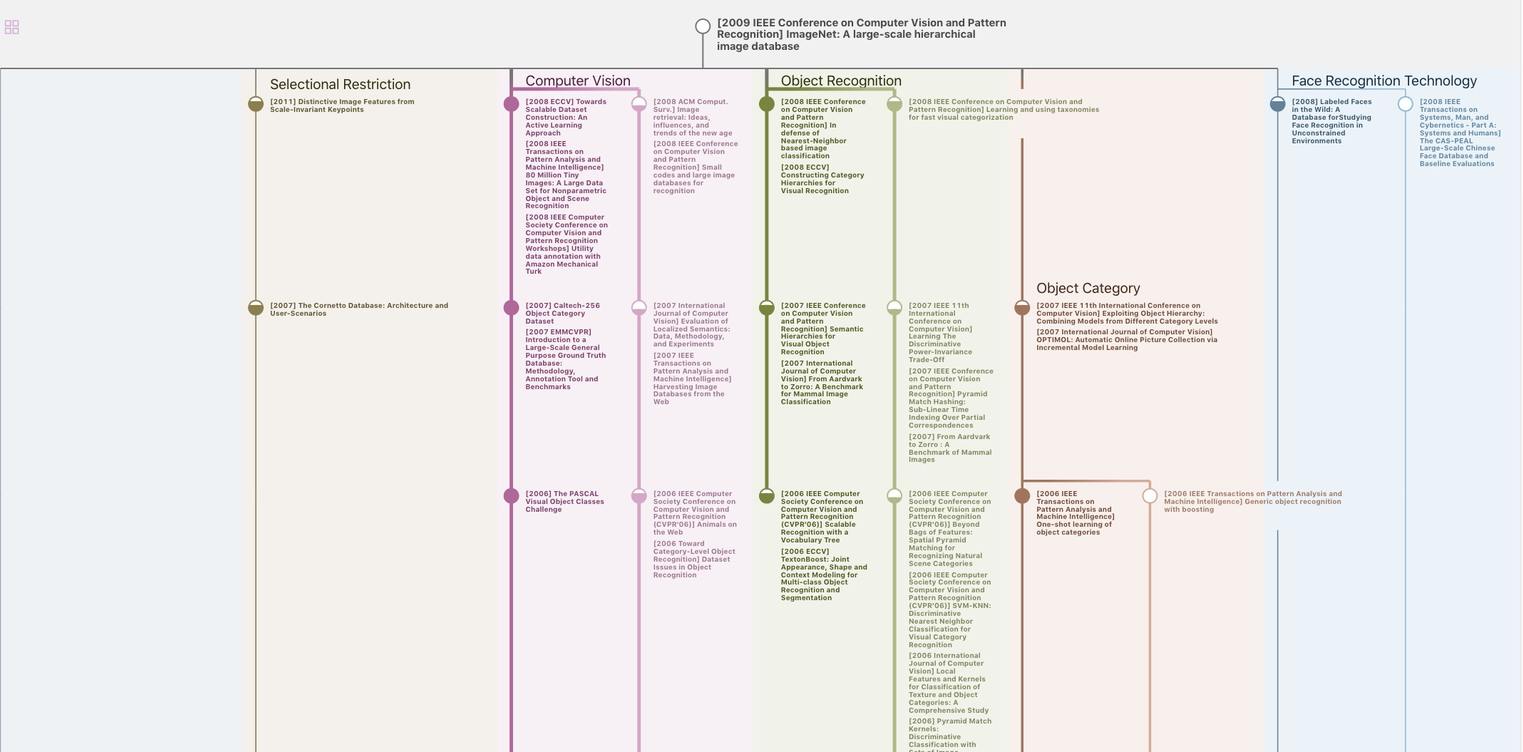
生成溯源树,研究论文发展脉络
Chat Paper
正在生成论文摘要