An improved rough set approach for optimal trust measure parameter selection in cloud environments
Soft Computing(2019)
摘要
The existence of a multitude of cloud service providers (CSPs) for each service type increases the difficulty in the identification of appropriate and trustworthy service providers based on their abilities and cloud users’ unique functional and non-functional quality-of-service (QoS) requirements. Further, the dynamic nature of the cloud ecosystem in terms of performance and new services increases the complexity of the cloud service selection problem. Trust-based service selection mechanisms which involve the intrinsic relations among the QoS parameters or trust measure parameters (TMPs) to evaluate the quality of the CSPs are the most preferred solution for the problem of cloud service selection. However, the accuracy of the trust-based service selection models and the CSP’s trust value relies on the optimality of the TMP subset obtained with respect to the service type. Hence, this work presents an efficient rough set theory-based hypergraph-binary fruit fly optimization (RST-HGBFFO), a cooperative bio-inspired technique to identify the optimal service-specific TMPs. Experiments on QWS dataset, Cloud Armor, and CISH—SASTRA trust feedback dataset reveal the predominance of RST-HGBFFO over the state-of-the-art feature selection techniques. The performance of RST-HGBFFO feature selection technique was validated using hypergraph-based computational model and WEKA tool in terms of reduct size, service ranking, classification accuracy, and time complexity.
更多查看译文
关键词
Trust measure parameters (TMPs),Rough set theory (RST),Hypergraph,Binary fruit fly optimization (BFFO),Hypergraph-based computational model (HGCM),Cloud service ranking
AI 理解论文
溯源树
样例
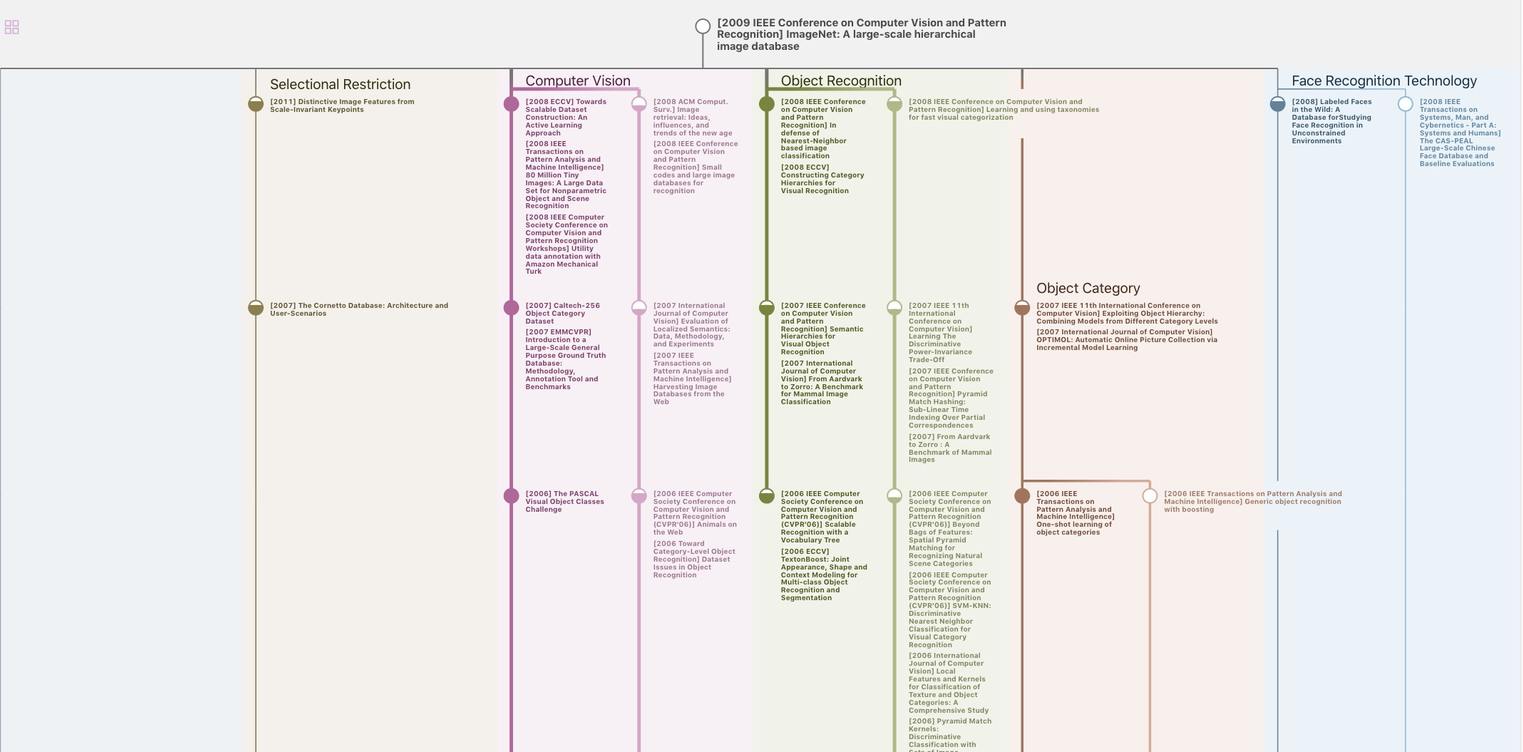
生成溯源树,研究论文发展脉络
Chat Paper
正在生成论文摘要