EAT-Rice: A predictive model for flanking gene expression of T-DNA insertion activation-tagged rice mutants by machine learning approaches.
PLOS COMPUTATIONAL BIOLOGY(2019)
摘要
T-DNA activation-tagging technology is widely used to study rice gene functions. When T-DNA inserts into genome, the flanking gene expression may be altered using CaMV 35S enhancer, but the affected genes still need to be validated by biological experiment. We have developed the EAT-Rice platform to predict the flanking gene expression of T-DNA insertion site in rice mutants. The three kinds of DNA sequences including UPS1K, DISTANCE, and MIDDLE were retrieved to encode and build a forecast model of two-layer machine learning. In the first-layer models, the features nucleotide context (N-gram), cis-regulatory elements (Motif), nucleotide physicochemical properties (NPC), and CG-island (CGI) were used to build SVM models by analysing the concealed information embedded within the three kinds of sequences. Logistic regression was used to estimate the probability of gene activation which as feature-encoding weighting within first-layer model. In the second-layer models, the NaiveBayesUpdateable algorithm was used to integrate these first layer-models, and the system performance was 88.33% on 5-fold cross-validation, and 79.17% on independent-testing finally. In the three kinds of sequences, the model constructed by Middle had the best contribution to the system for identifying the activated genes. The EAT-Rice system provided better performance and gene expression prediction at further distances when compared to the TRIM database. An online server based on EAT-rice is available at http://predictor.nchu.edu.tw/EAT-Rice. Author summary Among all the food crops, the rice is one of the staple foods in the human population, especially in Asia. However, the human population increases rapidly and the cultivated areas decrease in these decades. To solve the food crisis in the future, the rice researchers devote themselves to study on the gene function to increase the rice yield and stress tolerant ability. There are around 39000 annotated genes in rice, so scientists are hard to survey the gene functional because of the complexity and interactivity among the genes. Therefore, scientists put into a lot of manpower and funds into the field. The T-DNA (Transfer DNA) activation-tagging biotechnology has been wildly used on studies of rice gene function, however, it might influence the flanking genes expression when T-DNA inserted into the rice genome randomly. Thus, it will take lot of time for the researchers to validate the activation of genes by T-DNA enhancer. In these decades, as the increase of the biological data accumulation, the extraction of hidden information from this data is getting more and more important. To assist rice biologists in rapidly focusing the target gene affected by T-DNA. The application of machine learning methods in artificial intelligence (AI) and the establishment of prediction tool with biological data construction to correctly identify and classify target genes are of great significance in both theory and practice.
更多查看译文
AI 理解论文
溯源树
样例
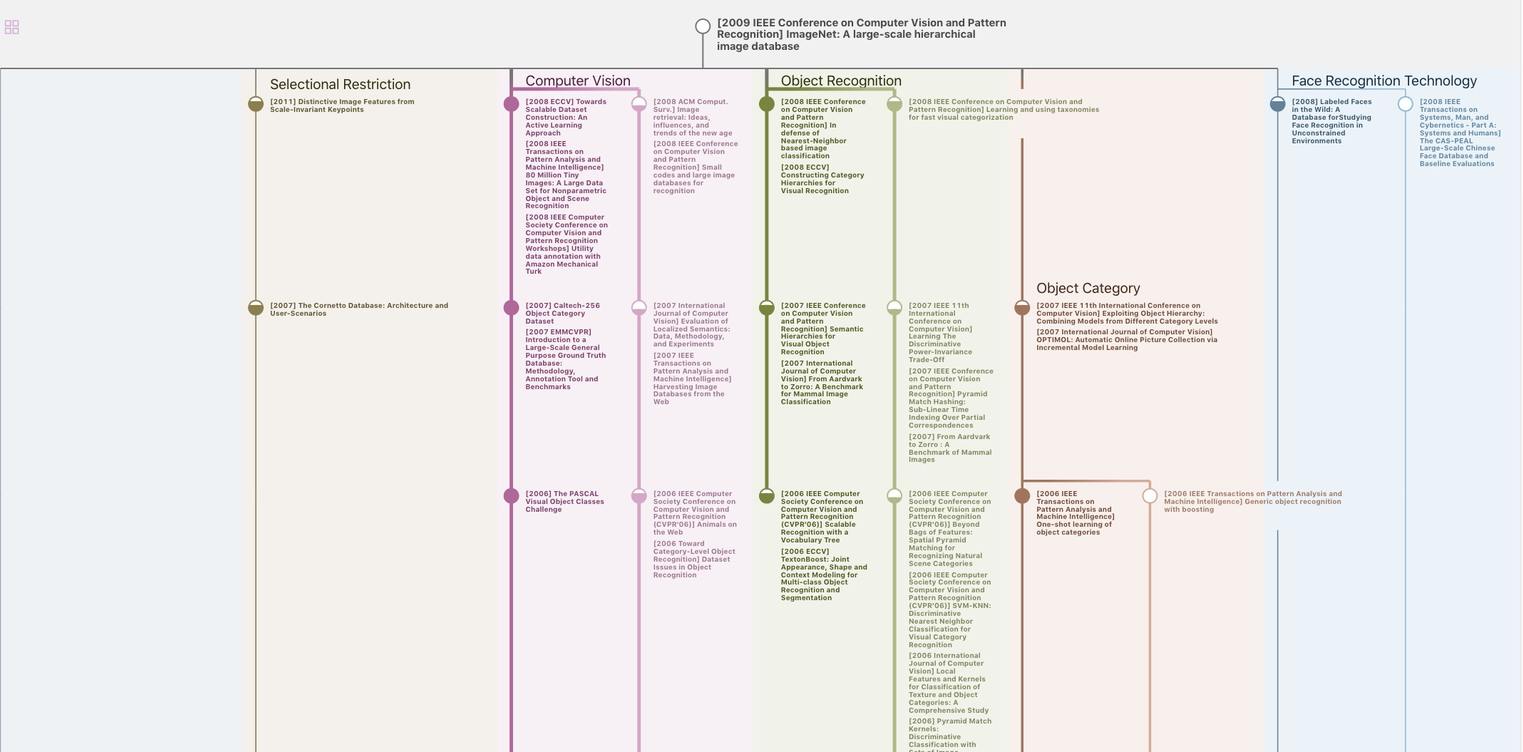
生成溯源树,研究论文发展脉络
Chat Paper
正在生成论文摘要