An in-depth study of similarity predicate committee
Information Processing and Management(2019)
摘要
In the last decades, many similarity measures are proposed, such as Jaccard coefficient, cosine similarity, BM25, language model, etc. Despite the effectiveness of the existing similarity measures, we observe that none of them can consistently outperform the others in most typical situations. Choosing which similarity predicate to use is usually treated as an empirical question by evaluating a particular task with a number of different similarity predicates, which is not computationally efficient and the obtained results are not portable. In this paper, we propose a novel approach to combine different similarity predicates together to form a committee so that we do not need to worry about choosing which of them to use. Empirically, we can obtain a better result than any individual similarity predicate, which is quite meaningful in practice. Specifically, our method models the problem of committee generation as a 0–1 integer programming problem based on the confidence of similarity predicates and the reliability of attributes. We demonstrate the effectiveness of our model by applying it on three datasets with controlled errors. Experimental results demonstrate that our similarity predicate committee is more robust and superior over existing individual similarity predicates. © 2018 Elsevier Ltd
更多查看译文
关键词
Ranking confidence,Reliability of attributes,Similarity predicate committee
AI 理解论文
溯源树
样例
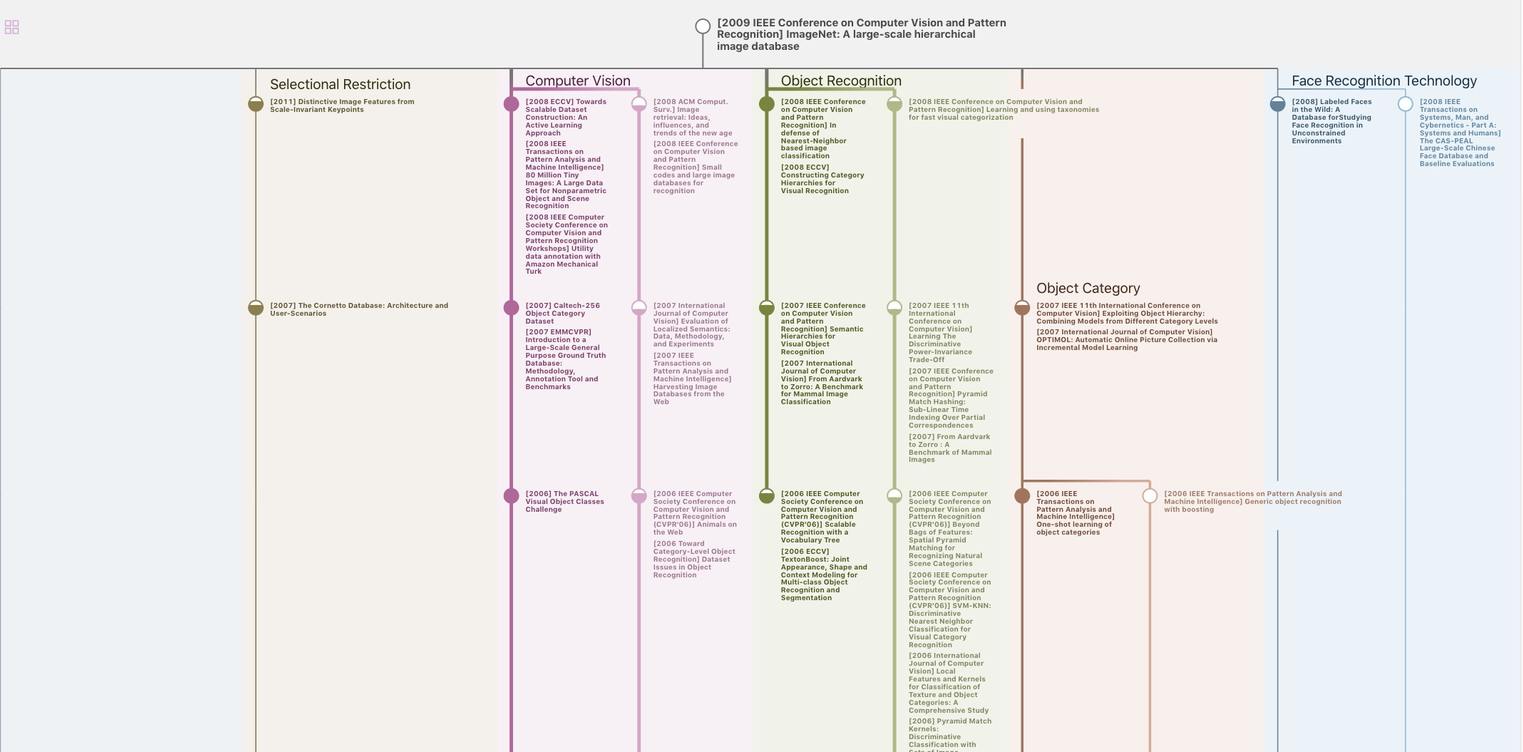
生成溯源树,研究论文发展脉络
Chat Paper
正在生成论文摘要