Hierarchical Neural Variational Model for Personalized Sequential Recommendation
WWW '19: The Web Conference on The World Wide Web Conference WWW 2019(2019)
摘要
In this paper, we study the problem of recommending personalized items to users given their sequential behaviors. Most sequential recommendation models only capture a user's short-term preference in a short session, and neglect his general (unchanged over time) and long-term preferences. Besides, they are all based on deterministic neural networks, and consider users' latent preferences as point vectors in a low-dimensional continuous space. However, in real world, the evolutions of users' preferences are full of uncertainties. We address this problem by proposing a hierarchical neural variational model (HNVM). HNVM models users' three preferences: general, long-term and short-term preferences through an unified hierarchical deep generative process. HNVM is a hierarchical recurrent neural network that enables it to capture both user's long-term and short-term preferences. Experiments on two public datasets demonstrate that HNVM outperforms state-of-the-art sequential recommendation methods.
更多查看译文
关键词
Generative Model, Recurrent Networks, Sequential Recommendation
AI 理解论文
溯源树
样例
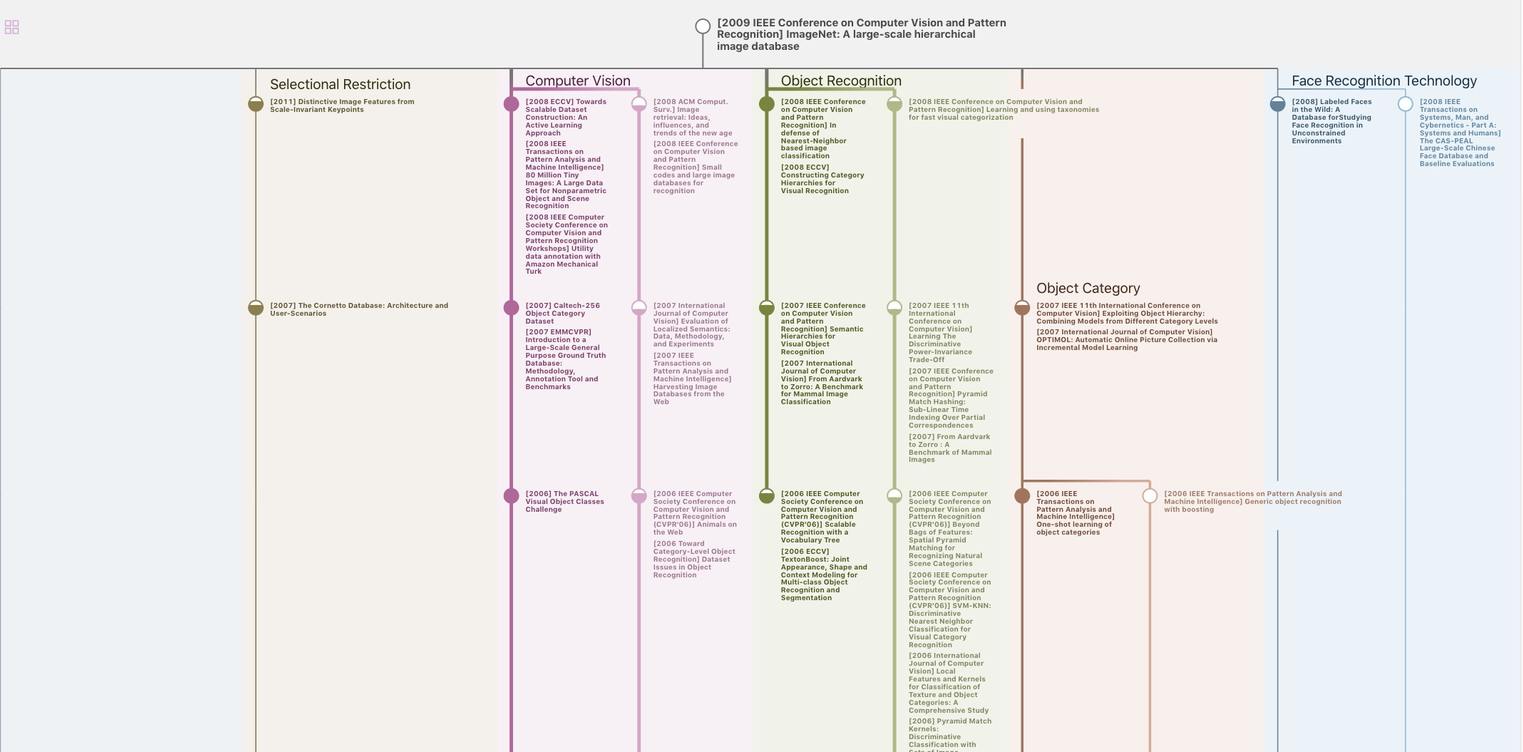
生成溯源树,研究论文发展脉络
Chat Paper
正在生成论文摘要