Appearance-Based Gaze Estimation via Gaze Decomposition and Single Gaze Point Calibration.
2020 IEEE Winter Conference on Applications of Computer Vision (WACV)(2019)
摘要
Appearance-based gaze estimation provides relatively unconstrained gaze tracking. However, subject-indepen\-dent models achieve limited accuracy partly due to individual variations. To improve estimation, we propose a novel gaze decomposition method and a single gaze point calibration method, motivated by our finding that the inter-subject squared bias exceeds the intra-subject variance for a subject-independent estimator. We decompose the gaze angle into a subject-dependent bias term and a subject-independent difference term between the gaze angle and the bias. The difference term is estimated by a deep convolutional network. For calibration-free tracking, we set the subject-dependent bias term to zero. For single gaze point calibration, we estimate the bias from a few images taken as the subject gazes at a point. Experiments on three datasets indicate that as a calibration-free estimator, the proposed method outperforms the state-of-the-art methods that use single model by up to $10.0\%$. The proposed calibration method is robust and reduces estimation error significantly (up to $35.6\%$), achieving state-of-the-art performance for appearance-based eye trackers with calibration.
更多查看译文
关键词
offset calibration,appearance-based gaze estimation,relatively unconstrained gaze tracking,subject-independent models,gaze decomposition method,calibration data,gaze target,inter-subject squared bias,intra-subject variance,subject-independent estimator,gaze estimate,subject-independent term,subject-dependent bias term,known gaze targets,low complexity calibration sets,calibration methods,complex calibration algorithms,calibration set,gaze targets
AI 理解论文
溯源树
样例
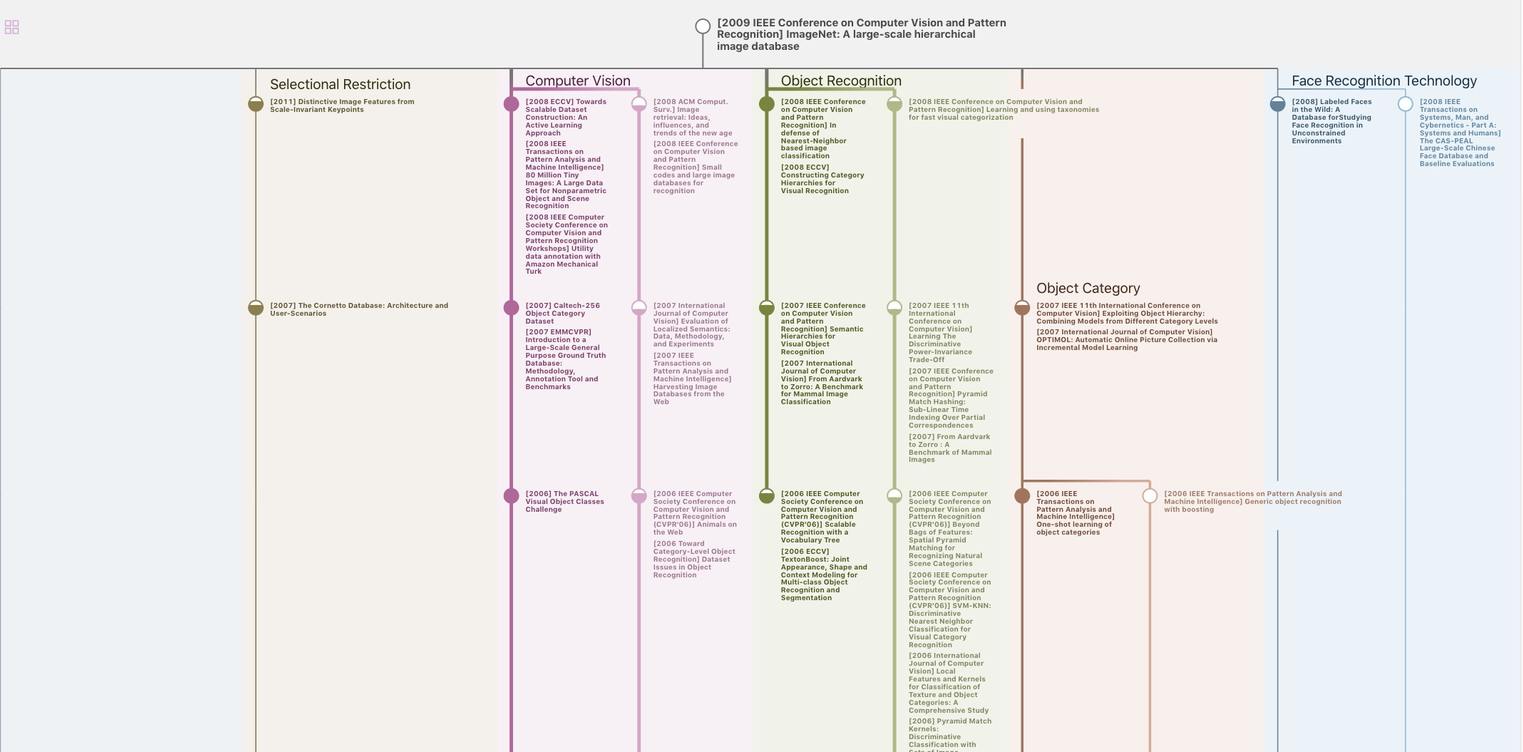
生成溯源树,研究论文发展脉络
Chat Paper
正在生成论文摘要