Learning and Planning in the Feature Deception Problem
GameSec(2020)
摘要
Today’s high-stakes adversarial interactions feature attackers who constantly breach the ever-improving security measures. Deception mitigates the defender’s loss by misleading the attacker to make suboptimal decisions. In order to formally reason about deception, we introduce the feature deception problem (FDP), a domain-independent model and present a learning and planning framework for finding the optimal deception strategy, taking into account the adversary’s preferences which are initially unknown to the defender. We make the following contributions. (1) We show that we can uniformly learn the adversary’s preferences using data from a modest number of deception strategies. (2) We propose an approximation algorithm for finding the optimal deception strategy given the learned preferences and show that the problem is NP-hard. (3) We perform extensive experiments to validate our methods and results. In addition, we provide a case study of the credit bureau network to illustrate how FDP implements deception on a real-world problem.
更多查看译文
关键词
Deception,Adversary,Approximation algorithm,Adversarial system,Computer security,Computer science
AI 理解论文
溯源树
样例
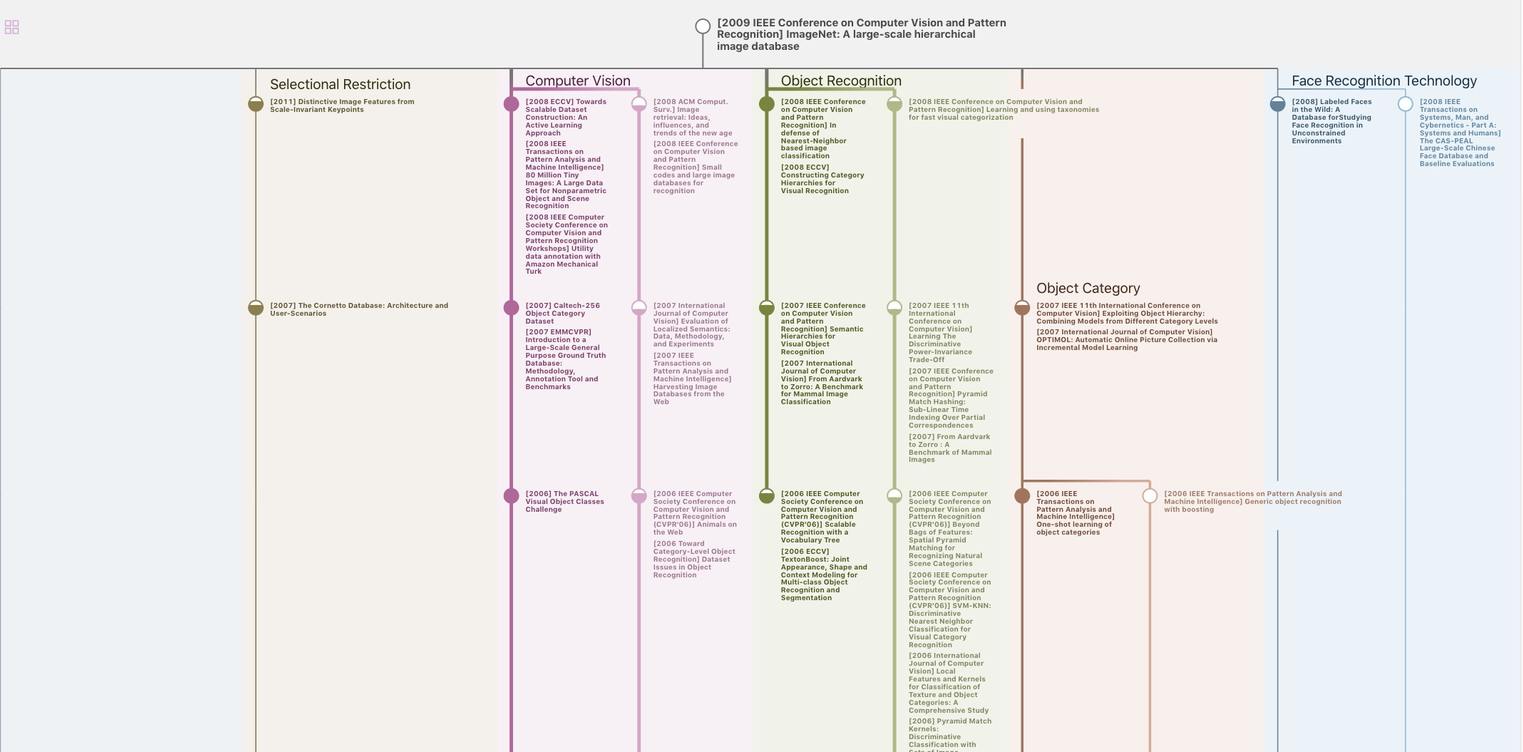
生成溯源树,研究论文发展脉络
Chat Paper
正在生成论文摘要