Hybrid Human Machine workflows for mobility management
Companion Proceedings of The 2019 World Wide Web Conference(2019)
摘要
Sustainable mobility is one of the main goals of both European and United Nations plans for 2030. The concept of Smart Cities has arisen as a way to achieve this goal by leveraging IoT interconnected devices to collect and analyse large quantities of data. However, several works have pointed out the importance of including the human factor, and in particular, citizens, to make sense of the collected data and ensure their engagement along the data value chain. This paper presents the design and implementation of two end-to-end hybrid human-machine workflows for solving two mobility problems: modal split estimation, and mapping mobility infrastructure. For modal split, we combine the use of i-Log, an app to collect data and interact with citizens, with reinforcement learning classifiers to continuously improve the accuracy of the classification, aiming at reducing the required interactions from citizens. For mobility infrastructure, we developed a system that uses remote crowdworkers to explore the city looking for Points of Interest, that is more scalable than sending agents on the field. Crowdsourced maps are then fused with existing maps (if available) to create a final map that then is validated on the field by citizens engaged through the i-Log app.
更多查看译文
关键词
crowdsourcing, hybrid workflows, map generation, modal split
AI 理解论文
溯源树
样例
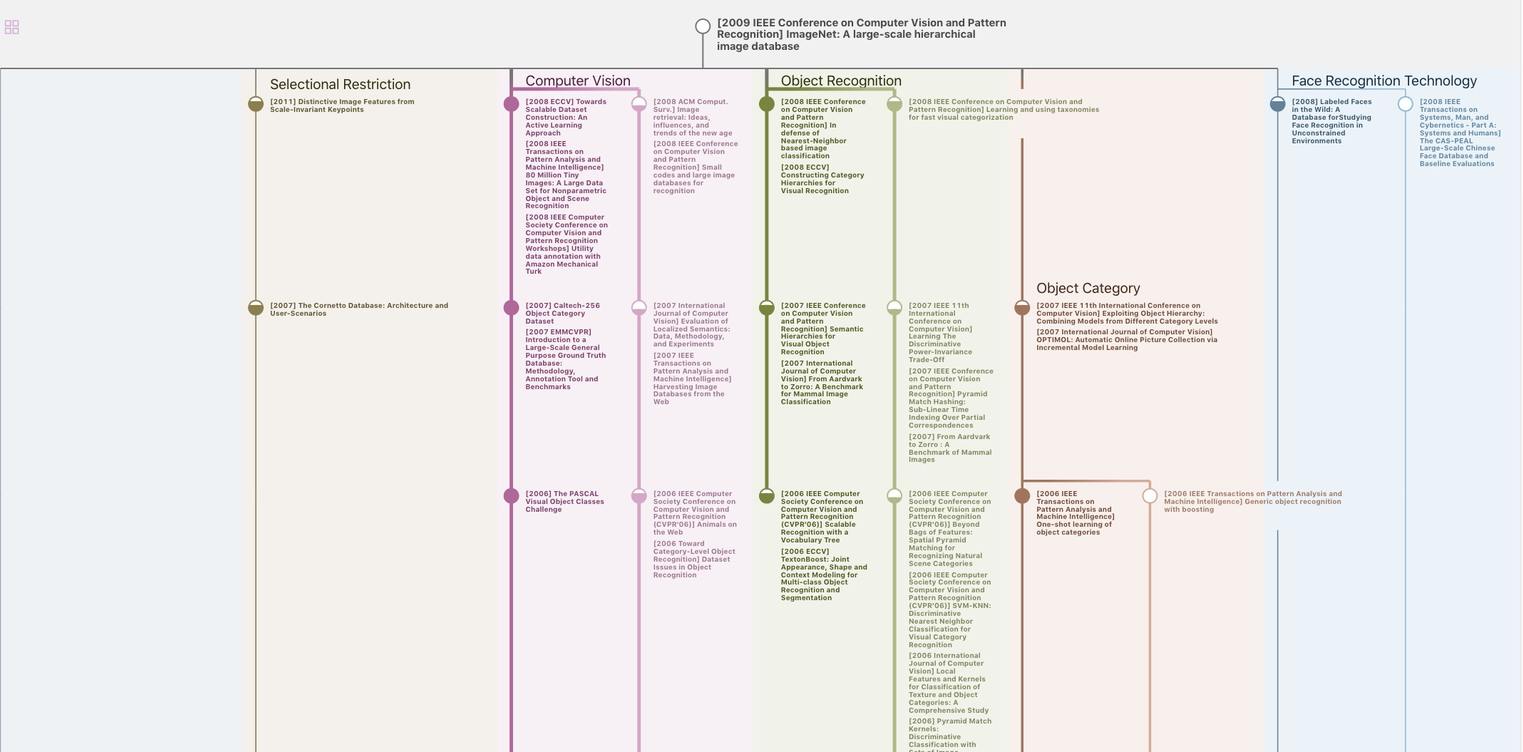
生成溯源树,研究论文发展脉络
Chat Paper
正在生成论文摘要