Embedding Based Cross-network User Identity Association Technology
Proceedings of the 2019 3rd International Conference on Digital Signal Processing(2019)
摘要
With the prosperity of online social networks, more and more users have multiple social accounts at the same time in heterogeneous social networks. Associating the same user identity between different social networks is beneficial for applications such as across-network information diffusion and cross-domain recommendation. User identity association across distinct social networks is to find accounts belonging to the same user without knowing the real identity of the users. Most of the existing identity correlation methods, including supervised learning and unsupervised learning methods, only use user's entity information in social networks, such as user attribute information and content information, nevertheless the inherent structural information of the networks is not fully used, so their effectiveness is often sensitive to the high dimension and sparsity of feature spaces. In this paper, we propose a novel model, called EUIA, which employs network embedding method to learn two low-dimensional representations of nodes of the two original networks respectively. Besides, we learn a mapping function across the learned two low-dimensional spaces, supervised by observed anchor links, for further predicting. In addition, we propose an effective optimization program to improve the accuracy of the model. Through experiments on the dataset of Facebook, we prove that the proposed EUIA model performs much better in accuracy than other baseline methods in cross-network user identity association problem.
更多查看译文
关键词
Cross-network, anchor links, cross-network mapping, network embedding, user identity associate
AI 理解论文
溯源树
样例
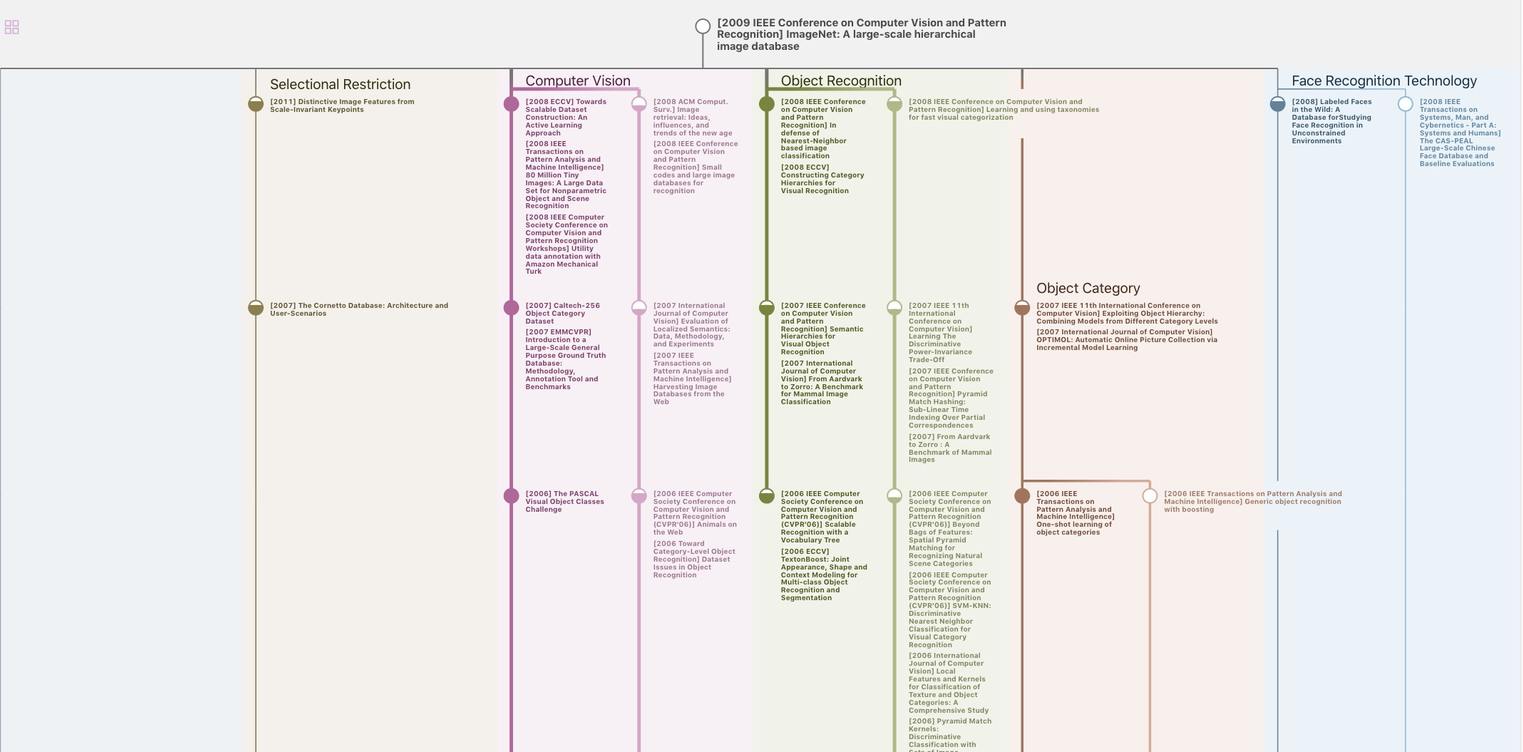
生成溯源树,研究论文发展脉络
Chat Paper
正在生成论文摘要