Exploring the Effects of Class-Specific Augmentation and Class Coalescence on Deep Neural Network Performance Using a Novel Road Feature Dataset
2018 IEEE Applied Imagery Pattern Recognition Workshop (AIPR)(2018)
摘要
The identification of nodal road network features in remote sensing imagery is an important object detection task due to its versatility of application. A successful capability enables urban sprawl tracking, automatic or semi-automated map accuracy validation and updating, and macro-scale infrastructure damage evaluation and tracking just to name a few. We have curated a custom, novel dataset that includes nodal road network features such as bridges, cul-de-sacs, freeway exchanges and exits, freeway overpasses, intersections, and traffic circles. From this curated data we have evaluated the use of deep machine learning for object recognition across two variations in this image dataset. These variations are expanded versus semantically coalesced classes. We have evaluated the performance of two deep convolutional neural networks, ResNet50 and Xception, to detect these features across these variations of the image datasets. We have also explored the use of class-specific data augmentation to improve the performance of the models trained for nodal road network feature detection. Cross-validation performance of the models evaluated on four variations of this nodal road network feature dataset range from 0.81 to 0.96 (F1 scores). Coalescing highly specific, semantically challenging classes into more semantically generalized classes has a significant impact on the accuracy of the models. Our analysis provides insight into if and how these techniques can improve the performance of machine learning models, facilitating application to broad area imagery analysis in numerous application domains.
更多查看译文
关键词
urban sprawl tracking,deep machine learning,image dataset,semantically coalesced classes,deep convolutional neural networks,class-specific data augmentation,remote sensing imagery,object detection task,deep neural network,road feature dataset,road network feature dataset,road network feature detection
AI 理解论文
溯源树
样例
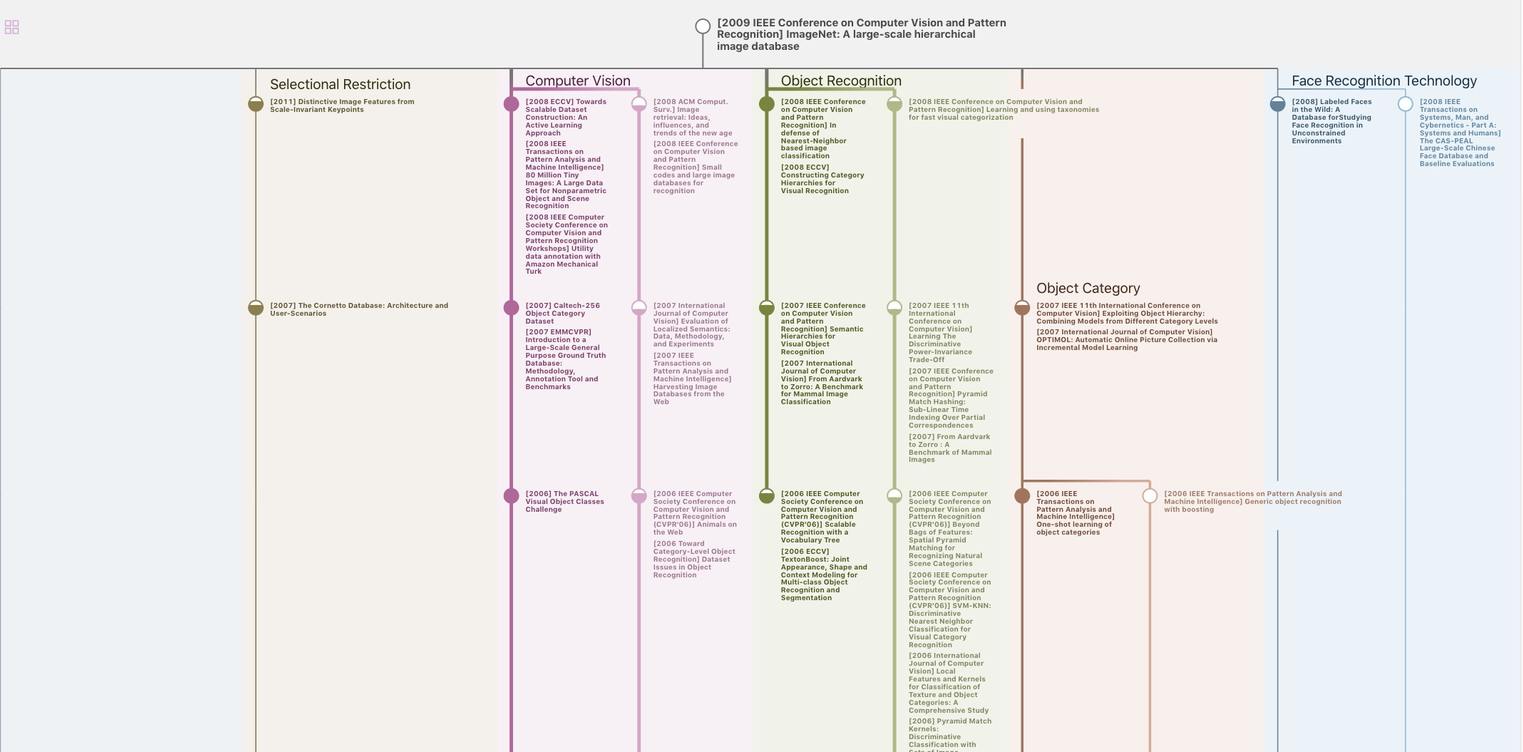
生成溯源树,研究论文发展脉络
Chat Paper
正在生成论文摘要