Automatic Building Footprint Extraction from Multi-Resolution Remote Sensing Images Using a Hybrid FCN.
ISPRS INTERNATIONAL JOURNAL OF GEO-INFORMATION(2019)
摘要
Recent technical developments made it possible to supply large-scale satellite image coverage. This poses the challenge of efficient discovery of imagery. One very important task in applications like urban planning and reconstruction is to automatically extract building footprints. The integration of different information, which is presently achievable due to the availability of high-resolution remote sensing data sources, makes it possible to improve the quality of the extracted building outlines. Recently, deep neural networks were extended from image-level to pixel-level labelling, allowing to densely predict semantic labels. Based on these advances, we propose an end-to-end U-shaped neural network, which efficiently merges depth and spectral information within two parallel networks combined at the late stage for binary building mask generation. Moreover, as satellites usually provide high-resolution panchromatic images, but only low-resolution multi-spectral images, we tackle this issue by using a residual neural network block. It fuses those images with different spatial resolution at the early stage, before passing the fused information to the Unet stream, responsible for processing spectral information. In a parallel stream, a stereo digital surface model (DSM) is also processed by the Unet. Additionally, we demonstrate that our method generalizes for use in cities which are not included in the training data.
更多查看译文
关键词
deep learning,building footprint extraction,fully convolutional neural network,World View-2 Imagery,Unet,stereo imagery,stereo DSM,pansharpening
AI 理解论文
溯源树
样例
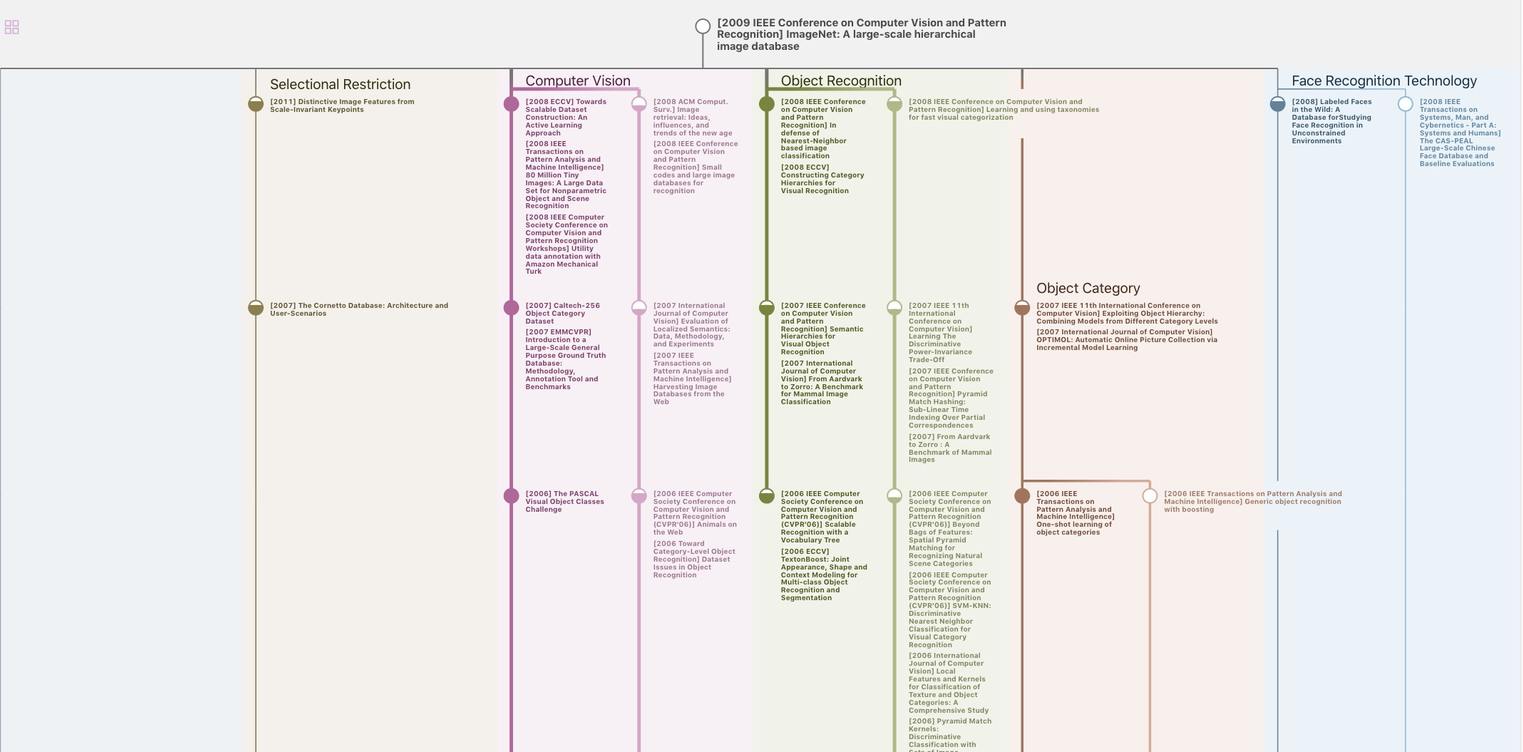
生成溯源树,研究论文发展脉络
Chat Paper
正在生成论文摘要