Adaptivity and Optimality: A Universal Algorithm for Online Convex Optimization
UAI(2020)
摘要
In this paper, we study adaptive online convex optimization, and aim to design a universal algorithm that achieves optimal regret bounds for multiple common types of loss functions. Existing universal methods are limited in the sense that they are optimal for only a subclass of loss functions. To address this limitation, we propose a novel online algorithm, namely Maler, which enjoys the optimal O(root T), O(d log T) and O(log T) regret bounds for general convex, exponentially concave, and strongly convex functions respectively. The essential idea is to run multiple types of learning algorithms with different learning rates in parallel, and utilize a meta-algorithm to track the best on the fly. Empirical results demonstrate the effectiveness of our method.
更多查看译文
AI 理解论文
溯源树
样例
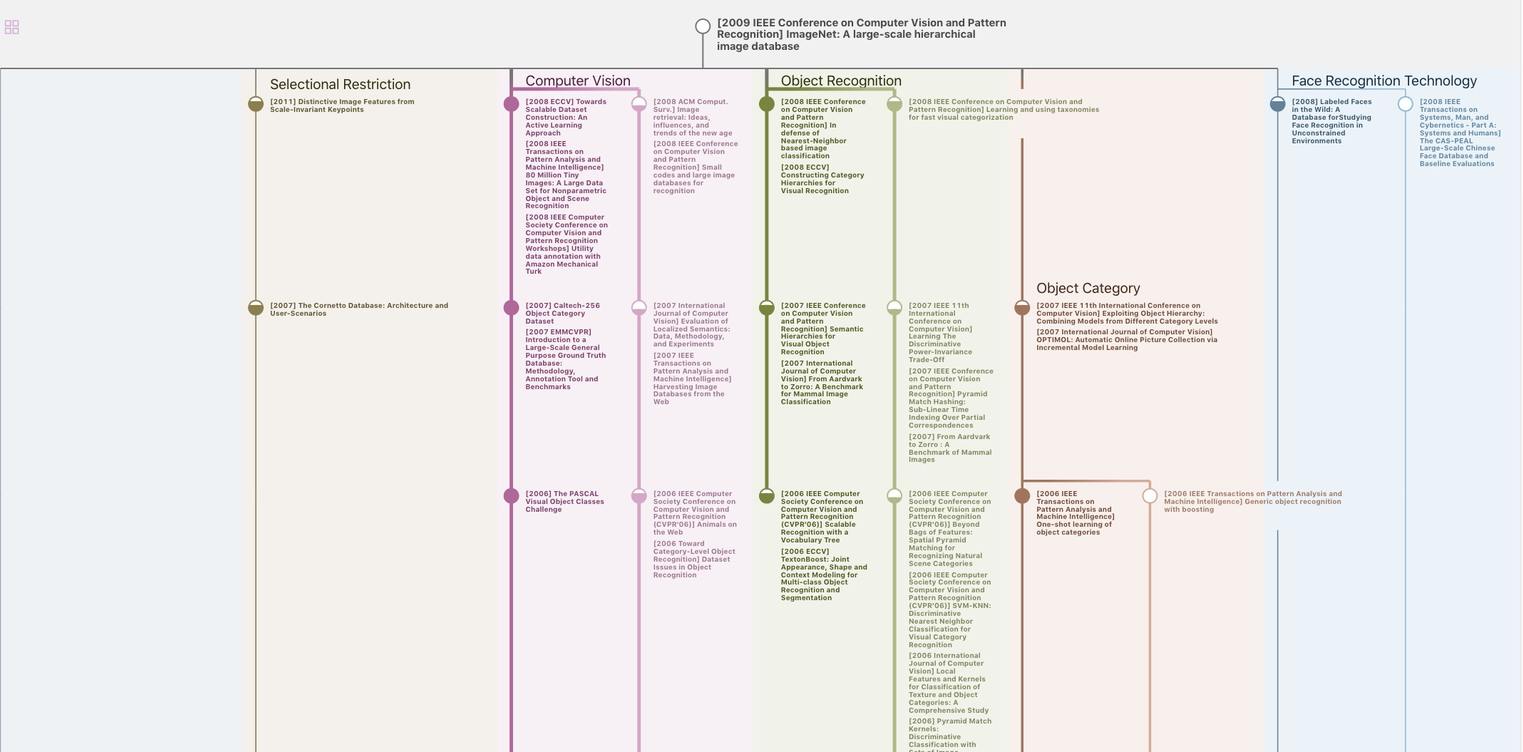
生成溯源树,研究论文发展脉络
Chat Paper
正在生成论文摘要