deWristified: handwriting inference using wrist-based motion sensors revisited
Proceedings of the 12th Conference on Security and Privacy in Wireless and Mobile Networks(2019)
摘要
Several recent research efforts have shown that privacy of handwritten information is vulnerable to inference threats that employ zero-permission motion sensors commonly found on wrist-wearables (e.g., smart watches and fitness bands) as information side-channels. While the adversary model in these earlier efforts have been reasonable and the proposed inference (or threat) frameworks themselves are practical and have technical merit, the related empirical evaluations suffer from several significant shortcomings, such as, use of specialized sensor hardware and highly constrained or restrictive experimental procedures, to name a few. As a result, it is hard to estimate the practical feasibility of these threats from existing research results in the literature, and thus, the extent to which end-users must be concerned about the possibility of such attacks in real-life. To answer the above question, this paper replicates some of the well-known wrist motion-based handwriting inference frameworks in the literature in order to (re)evaluate their success or accuracy in natural, unrestricted handwriting scenarios and settings by employing commercially available wrist-wearables. The results of these extensive replication and (re)evaluation studies highlight several characteristics in motion data corresponding to natural handwriting scenarios, which were either not observed or ignored by earlier efforts, and contribute to poor inference accuracy of the corresponding frameworks. In summary, accurate and practical handwriting inference using motion data (side-channeled) from consumer-grade wrist-wearables is difficult primarily due to unique and/or inconsistent handwriting behavior observed in natural writing.
更多查看译文
AI 理解论文
溯源树
样例
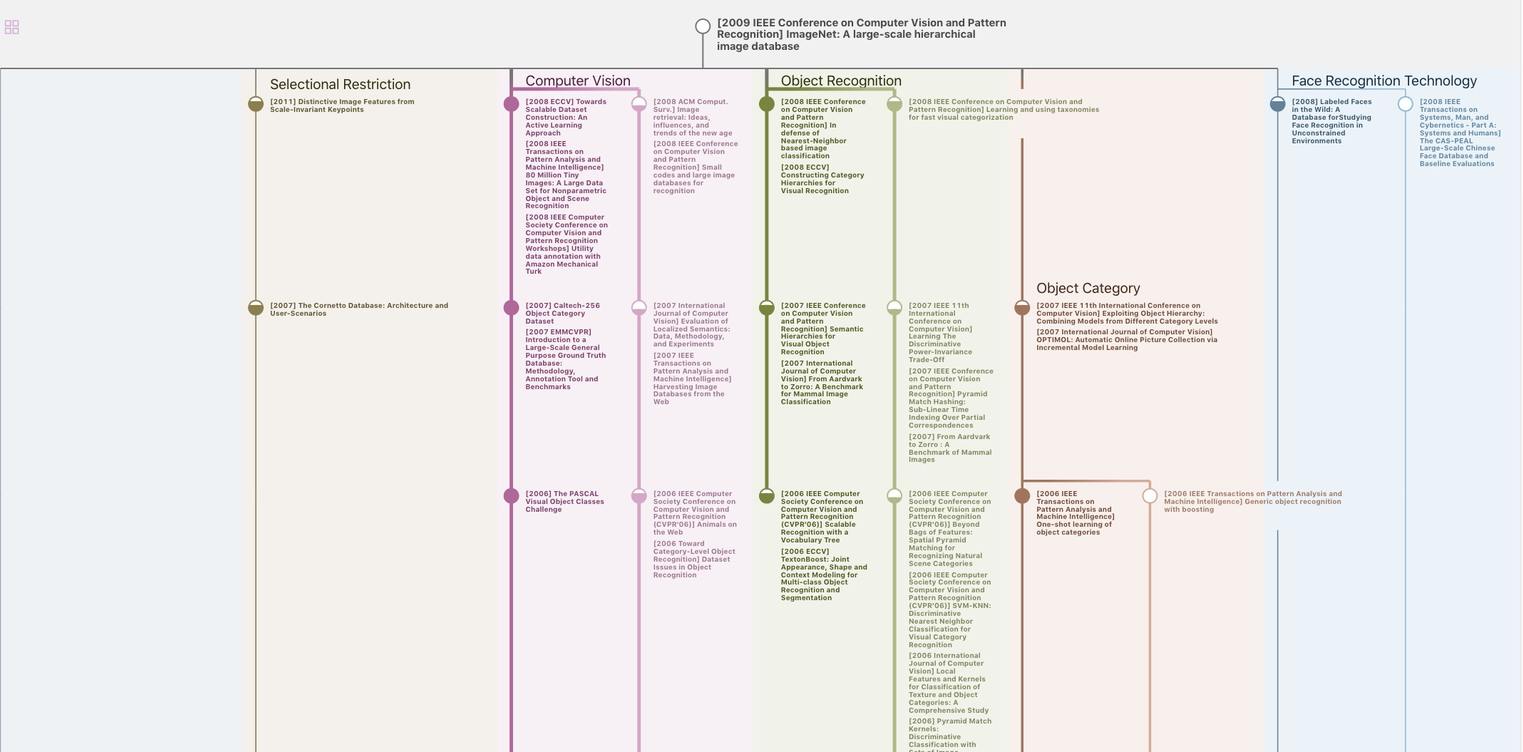
生成溯源树,研究论文发展脉络
Chat Paper
正在生成论文摘要