Circumventing kinetics in biogeochemical modeling.
Proceedings of the National Academy of Sciences of the United States of America(2019)
摘要
Microbial metabolism drives biogeochemical fluxes in virtually every ecosystem. Modeling these fluxes is challenged by the incredible diversity of microorganisms, whose kinetic parameters are largely unknown. In poorly mixed systems, such as stagnant water columns or sediments, however, long-term bulk microbial metabolism may become limited by physical transport rates of substrates across space. Here we mathematically show that under these conditions, biogeochemical fluxes are largely predictable based on the system's transport properties, chemical boundary conditions, and the stoichiometry of metabolic pathways, regardless of the precise kinetics of the resident microorganisms. We formalize these considerations into a predictive modeling framework and demonstrate its use for the Cariaco Basin subeuphotic zone, one of the largest anoxic marine basins worldwide. Using chemical concentration data solely from the upper boundary (depth 180 m) and lower boundary (depth 900 m), but without a priori knowledge of metabolite fluxes, chemical depth profiles, kinetic parameters, or microbial species composition, we predict the concentrations and vertical fluxes of biologically important substances, including oxygen, nitrate, hydrogen sulfide, and ammonium, across the entire considered depth range (180-900 m). Our predictions largely agree with concentration measurements over a period of 14 years ([Formula: see text] = 0.78-0.92) and become particularly accurate during a period where the system was near biogeochemical steady state (years 2007-2009, [Formula: see text] = 0.86-0.95). Our work enables geobiological predictions for a large class of ecosystems without knowledge of kinetic parameters or geochemical depth profiles. Conceptually, our work provides a possible explanation for the decoupling between microbial species composition and bulk metabolic function, observed in various ecosystems.
更多查看译文
AI 理解论文
溯源树
样例
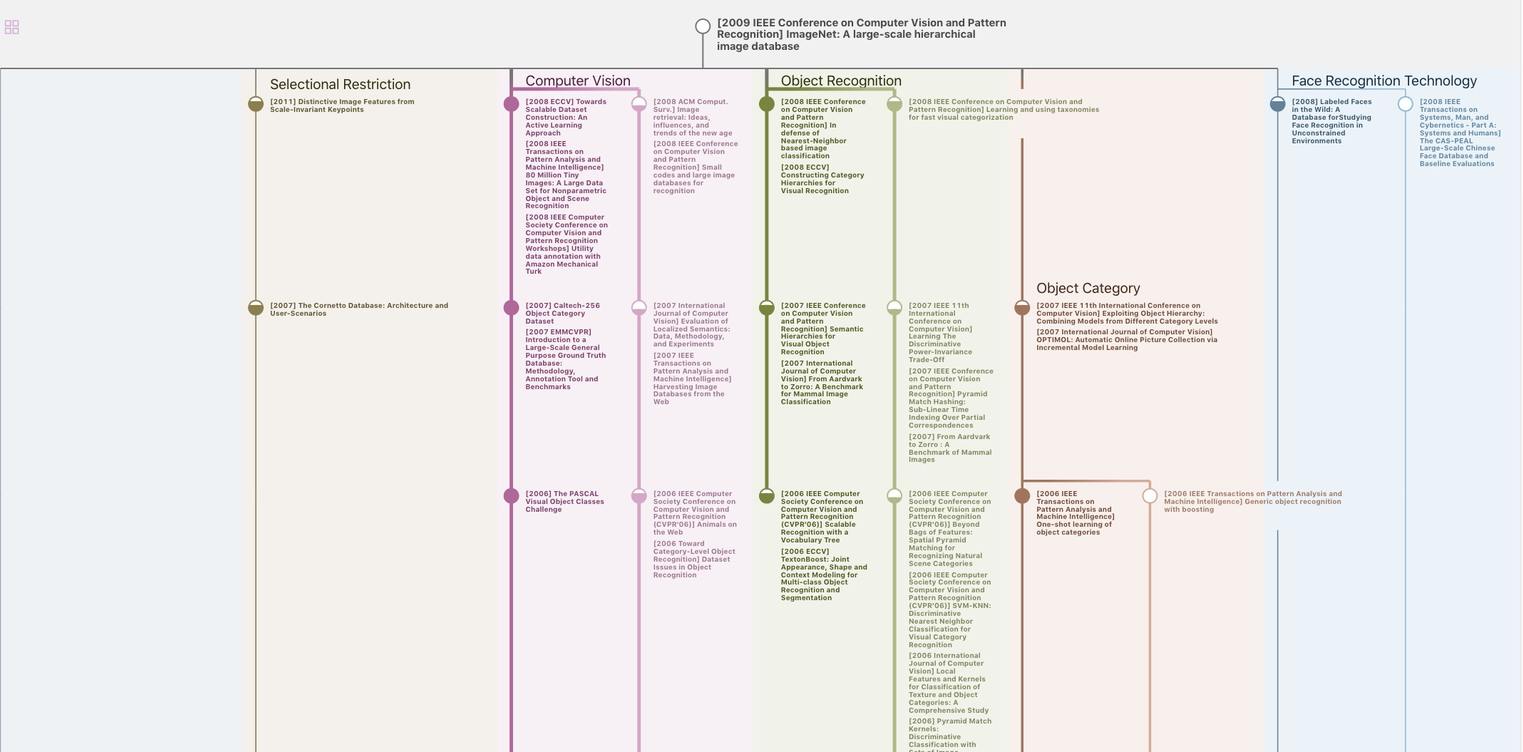
生成溯源树,研究论文发展脉络
Chat Paper
正在生成论文摘要