Choosing the Right Loss Function for Multi-Label Emotion Classification
Journal of intelligent & fuzzy systems(2019)
摘要
Natural Language Processing problems has recently been benefited for the advances in Deep Learning. Many of these problems can be addressed as a multi-label classification problem. Usually, the metrics used to evaluate classification models are different from the loss functions used in the learning process. In this paper, we present a strategy to incorporate evaluation metrics in the learning process in order to increase the performance of the classifier according to the measure we are interested to favor. Concretely, we propose soft versions of the Accuracy, micro-F-1, and macro-F-1 measures that can be used as loss functions in the back-propagation algorithm. In order to experimentally validate our approach, we tested our system in an Emotion Classification task proposed at the International Workshop on Semantic Evaluation, SemEval-2018. Using a Convolutional Neural Network trained with the proposed loss functions we obtained significant improvements both for the English and the Spanish corpora.
更多查看译文
关键词
Deep Learning,loss function,multi-label classification,Natural Language Processing,Emotion Classification
AI 理解论文
溯源树
样例
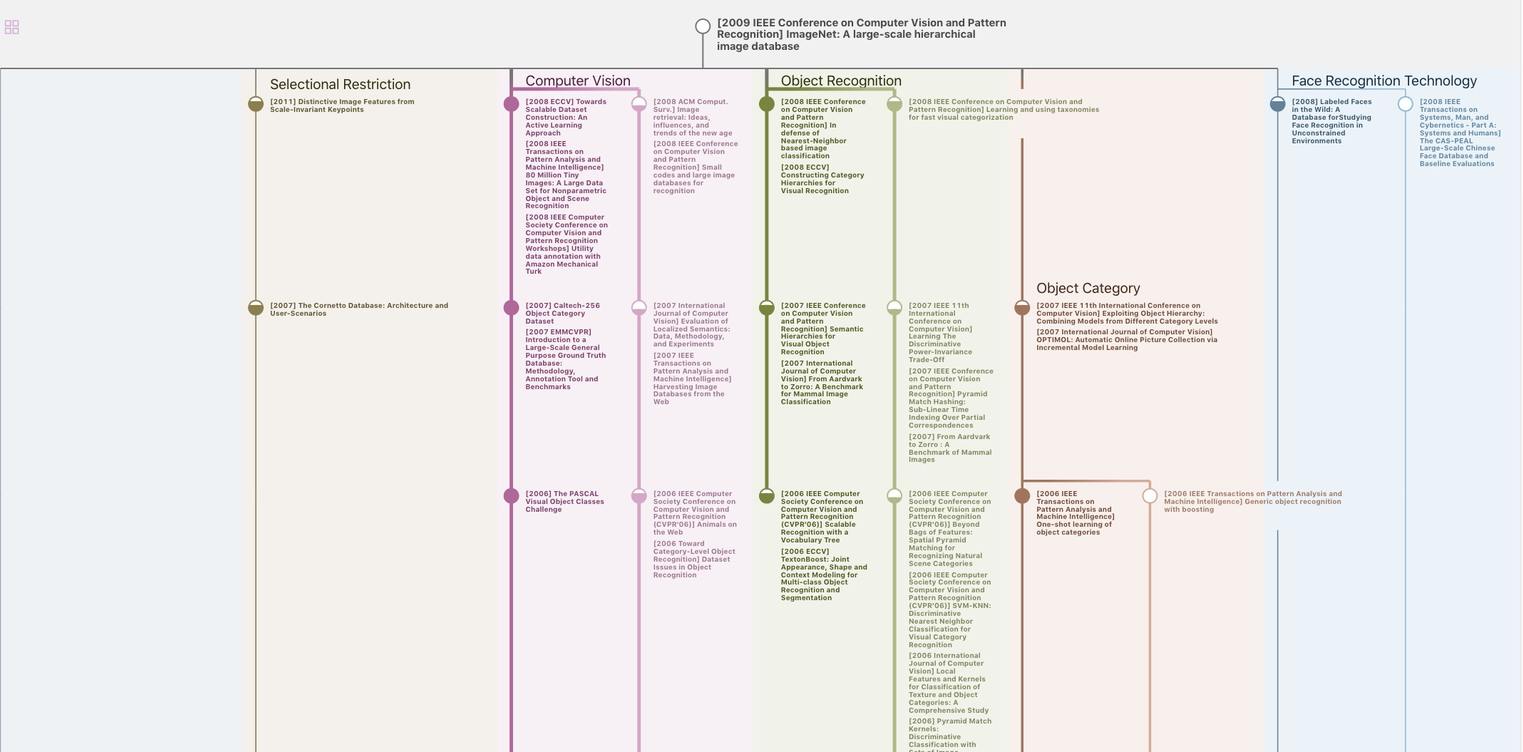
生成溯源树,研究论文发展脉络
Chat Paper
正在生成论文摘要