Beyond global fusion: A group-aware fusion approach for multi-view image clustering.
Information Sciences(2019)
摘要
Images can be represented by multiple views and each view describes a specific visual appearance. Compared with single view learning method, multi-view methods can integrate information of different views to generate better clustering performance. Most of the existing multi-view methods assume that the importance of each view is the same to all the images. However, since visual appearance of images are different, the description abilities of different features vary with images. To solve this problem, a group-aware multi-view fusion approach is proposed in this paper. Specifically, images are partitioned into groups according to their visual appearance, and different fusion weights are assigned to different groups. We develop two paradigms under our group-aware fusion framework: pair-wise fusion and center-wise fusion. The former focuses on generating more accurate fusion results, while the latter achieves lower computational complexity. We design an optimization objective function which combines consensus and discrimination criterion to select more reliable and discriminative views for multi-view fusion. The clustering results and the fusion weights are learned by an iterative optimization algorithm. Experiments on four real-world image datasets indicate that our approach achieves promising image clustering performance over the existing methods.
更多查看译文
关键词
Multi-view learning,Local fusion strategy,Group-aware fusion,Image clustering
AI 理解论文
溯源树
样例
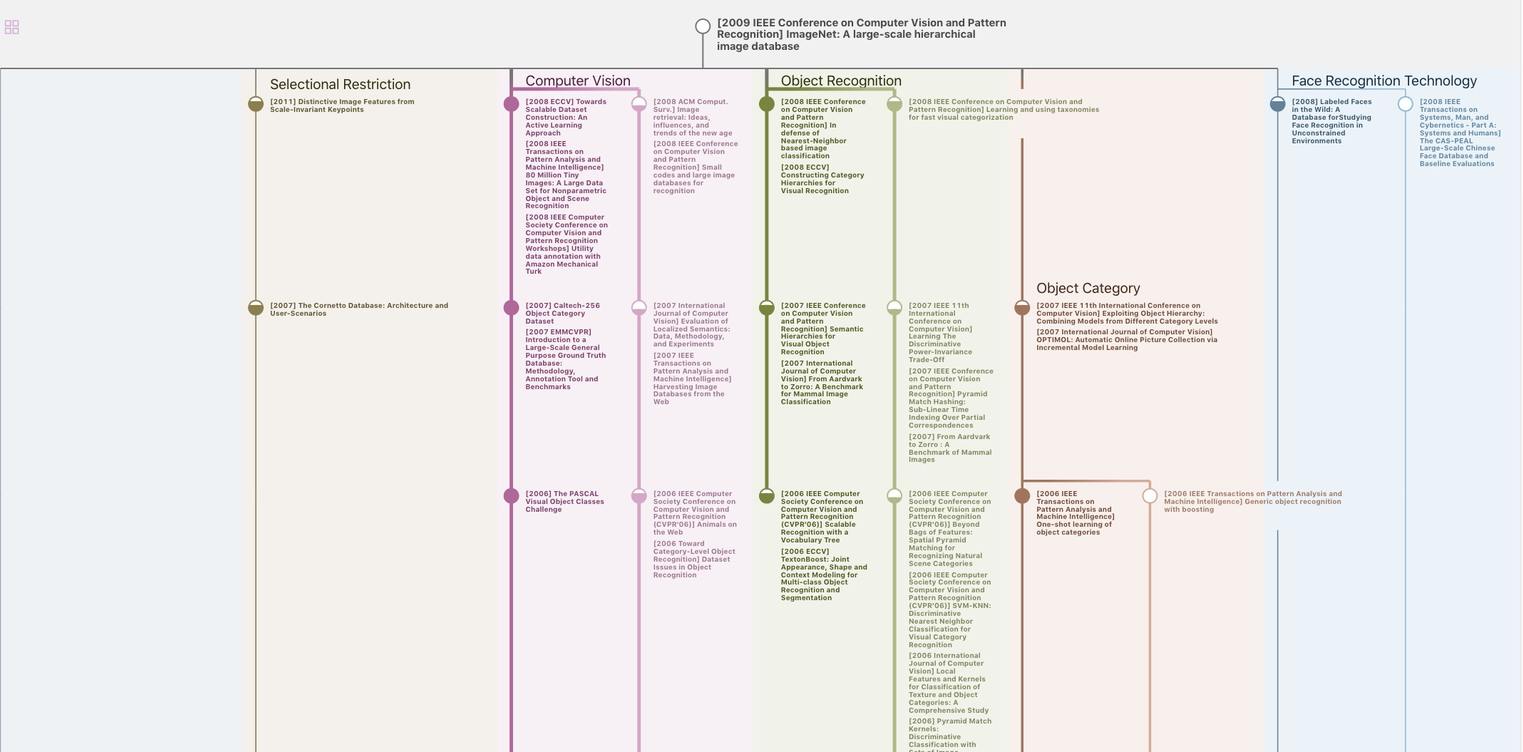
生成溯源树,研究论文发展脉络
Chat Paper
正在生成论文摘要