Adaptive long-term control of biological neural networks with Deep Reinforcement Learning.
Neurocomputing(2019)
摘要
Driving activity patterns in the brain to desired levels is an important therapeutic strategy for many neurological disorders. The highly dynamic nature of neuronal networks and changes with disease progression create an urgent need for closed-loop control. Without adequate mathematical models of such complex networks, it remains unclear how tractable control problems can be formulated and solved for neurobiological systems. Reinforcement Learning (RL) is a promising tool to address such challenges, but has rarely been used for the long-term control of live, plastic neural networks. This is a difficult problem since it requires the controller to adapt to poorly characterized non-stationary background processes that alter stimulus-response relations over time.
更多查看译文
关键词
Reinforcement Learning,Biological neural networks,Artificial neural networks,Deep learning,Deep Reinforcement Learning,Neurostimulation,Closed-loop neurostimulation
AI 理解论文
溯源树
样例
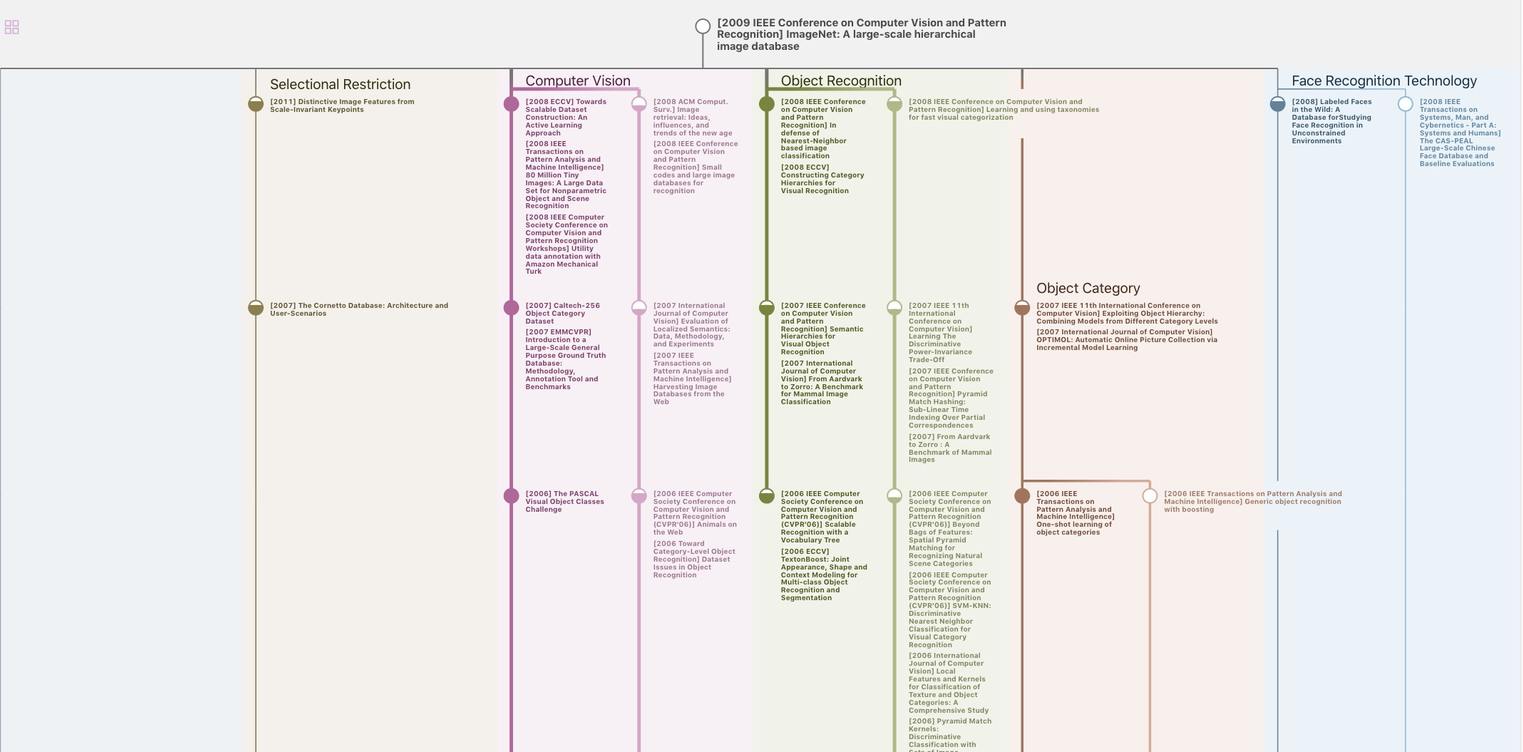
生成溯源树,研究论文发展脉络
Chat Paper
正在生成论文摘要