Decouple and Stretch: A Boost to Channel Pruning
2018 IEEE 37th International Performance Computing and Communications Conference (IPCCC)(2018)
摘要
Deep Neural Networks (DNNs) have shown superior performance on a variety of artificial intelligence problems. Reducing the resource usage of DNN is critical to adding intelligence on Internet of Things (IoT) devices. Channel pruning based network compression shows effective reduction simultaneously on storage, memory and computation without specialized software on general platforms. But limited by pruning flexibility, channel pruning methods have relatively low compression rate for a given target performance. In this paper, we demonstrate that channel pruning becomes more robust to decision errors by reducing the granularity of filters. Then we propose a Decouple and Stretch (DS) scheme to enhance channel pruning. Under this scheme, each filter in a specific layer is decoupled into two small spatial-wise filters, and the spatial-wise filters are stretched into two successive convolutional layers. Our scheme obtains up to 49% improvement on compression and 35% improvement on acceleration. To further demonstrate hardware compatibility, we deploy pruned networks on the FPGA, and the network produced by Decouple and Stretch scheme is more hardware-friendly with latency reduced by 42%.
更多查看译文
关键词
deep learning,Internet of Things,network compression,channel pruning,hardware resources
AI 理解论文
溯源树
样例
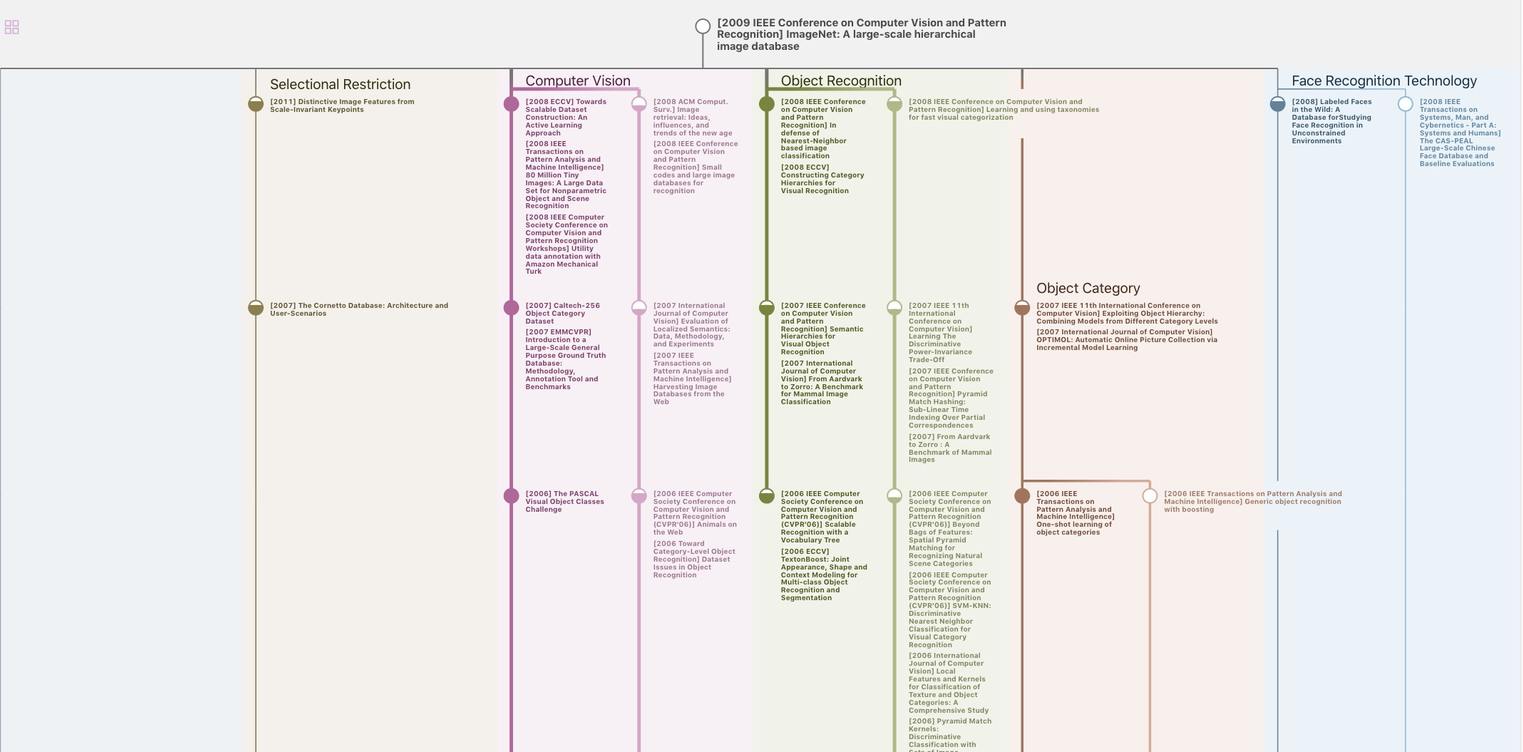
生成溯源树,研究论文发展脉络
Chat Paper
正在生成论文摘要