A Framework for Epilepsy Classification Using Modified Sparse Representation Classifiers and Naïve Bayesian Classifier from Electroencephalogram Signals
JOURNAL OF MEDICAL IMAGING AND HEALTH INFORMATICS(2016)
摘要
The record of the electrical activity of the brain is the Electroencephalogram (EEG). For diagnosing neurological disorders like epilepsy, EEG serves as an indispensable tool. In this paper, dimensionality reduction concepts like Fuzzy Mutual Information (FMI), Independent Component Analysis (ICA), Linear Graph Embedding (LGE), Linear Discriminant Analysis (LDA) and finally Variational Bayesian Matrix Factorization (VBMF) is utilized effectively to reduce the dimensions of the recorded EEG data. The dimensionally reduced EEG data values are then fed to the post classifiers for the perfect classification of epilepsy from EEG signals. This paper proposes novel epilepsy classification techniques using post classifiers like Mahalonobis based Sparse Representation Classifiers (M-SRC), Modified Expectation Maximization Based Sparse Representation Classifiers (MEM-SRC), Particle Swarm Optimization Based Sparse Representation Classifier (PSO-SRC) and finally Naive Bayesian Classifier (NBC). The highest accuracy is obtained when the dimensionality reduction technique used is LDA and classified with PSO-SRC as of 99.27%.
更多查看译文
关键词
M-SRC,MEM-SRC,PSO,SRC,NBC
AI 理解论文
溯源树
样例
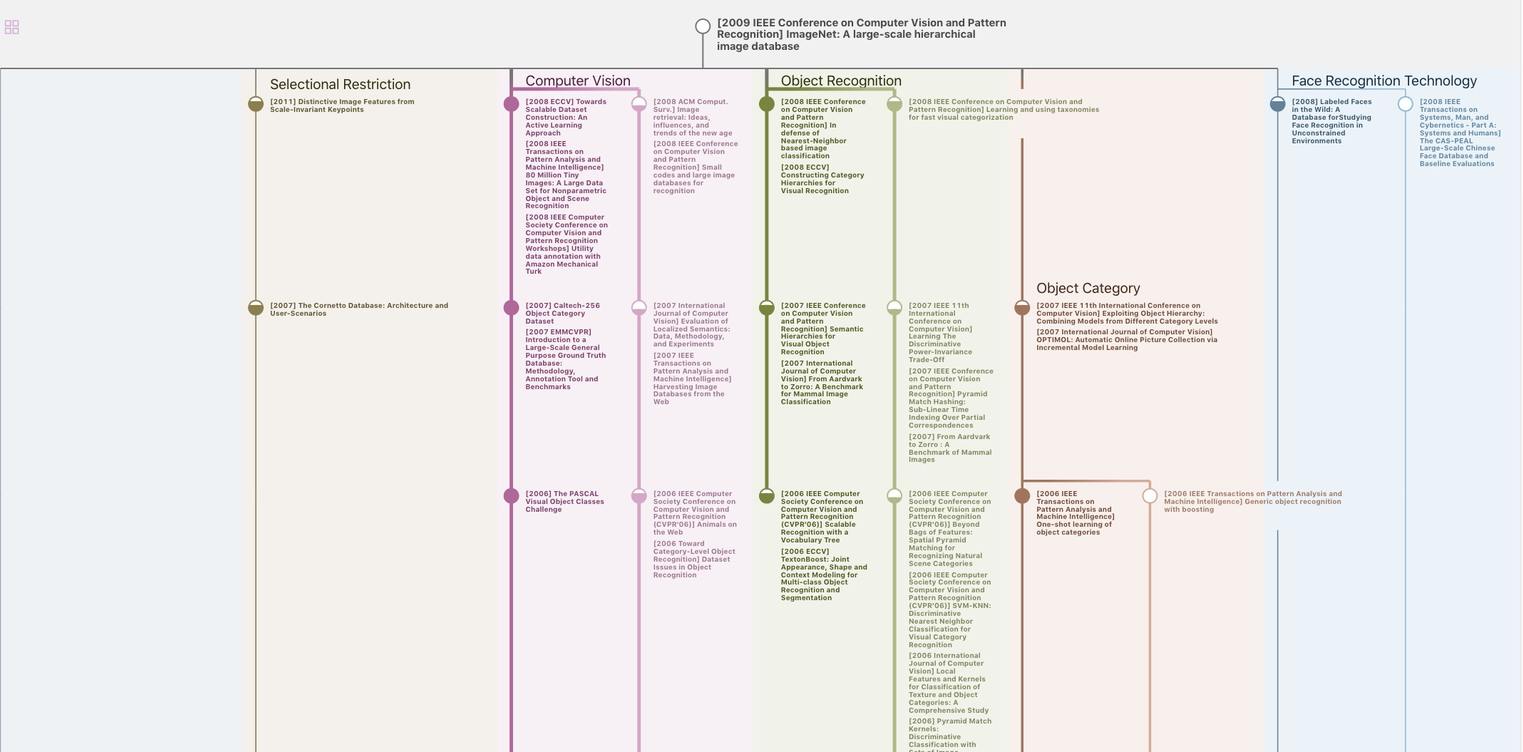
生成溯源树,研究论文发展脉络
Chat Paper
正在生成论文摘要