Derivative‐Based Global Sensitivity Analysis: Upper Bounding of Sensitivities in Seismic‐Hazard Assessment Using Automatic Differentiation
BULLETIN OF THE SEISMOLOGICAL SOCIETY OF AMERICA(2017)
摘要
Seismic-hazard assessment is of great importance within the field of engineering seismology. Nowadays, it is common practice to define future seismic demands using probabilistic seismic-hazard analysis (PSHA). Often it is neither obvious nor transparent how PSHA responds to changes in its inputs. In addition, PSHA relies on many uncertain inputs. Sensitivity analysis (SA) is concerned with the assessment and quantification of how changes in the model inputs affect the model response and how input uncertainties influence the distribution of the model response. Sensitivity studies are challenging primarily for computational reasons; hence, the development of efficient methods is of major importance. Powerful local (deterministic) methods widely used in other fields can make SA feasible, even for complex models with a large number of inputs; for example, automatic/algorithmic differentiation (AD)-based adjoint methods. Recently developed derivative-based global sensitivity measures can combine the advantages of such local SA methods with efficient sampling strategies facilitating quantitative global sensitivity analysis (GSA) for complex models. In our study, we propose and implement exactly this combination. It allows an upper bounding of the sensitivities involved in PSHA globally and, therefore, an identification of the noninfluential and the most important uncertain inputs. To the best of our knowledge, it is the first time that derivative-based GSA measures are combined with AD in practice. In addition, we show that first-order uncertainty propagation using the delta method can give satisfactory approximations of global sensitivity measures and allow a rough characterization of the model output distribution in the case of PSHA. An illustrative example is shown for the suggested derivative-based GSA of a PSHA that uses stochastic ground-motion simulations.
更多查看译文
AI 理解论文
溯源树
样例
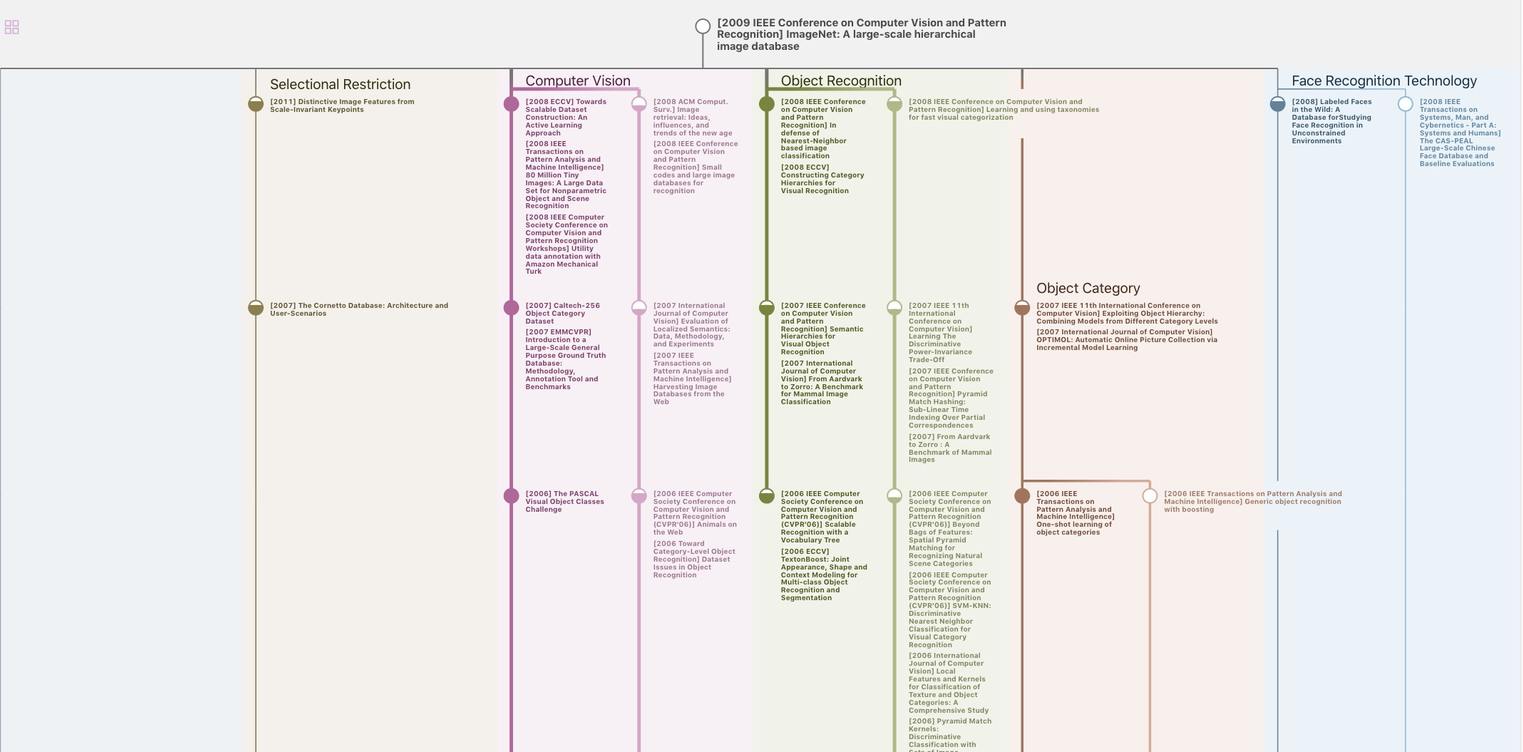
生成溯源树,研究论文发展脉络
Chat Paper
正在生成论文摘要