Improved Short-Term Point And Interval Forecasts Of The Daily Maximum Tropospheric Ozone Levels Via Singular Spectrum Analysis
ENVIRONMETRICS(2017)
摘要
We propose a general method for producing reliable short-term point and interval forecasts of daily maximum tropospheric ozone concentrations, a time series with a significant seasonal component and correlated errors in both mean and volatility. Our method combines symmetrizing data transformation and time series modeling techniques called the singular spectrum analysis and autoregressive models. Specifically, we transform the underlying distribution of the data to a symmetric distribution by applying the log and Yeo-Johnson transformation for accurate positive point forecasts. Moreover, we consider seasonality in both the mean and volatility of the time series, as well as empirical quantile estimation, for better interval forecasts. The accuracy of the proposed method is verified rigorously at selected sites in the United States with differing latitudes, geography, and degrees of anthropogenic activities. The results indicate that the proposed method seems to outperform the standard method that does not consider data transformation and seasonality in volatility.
更多查看译文
关键词
data transformation, seasonality, time series analysis, volatility
AI 理解论文
溯源树
样例
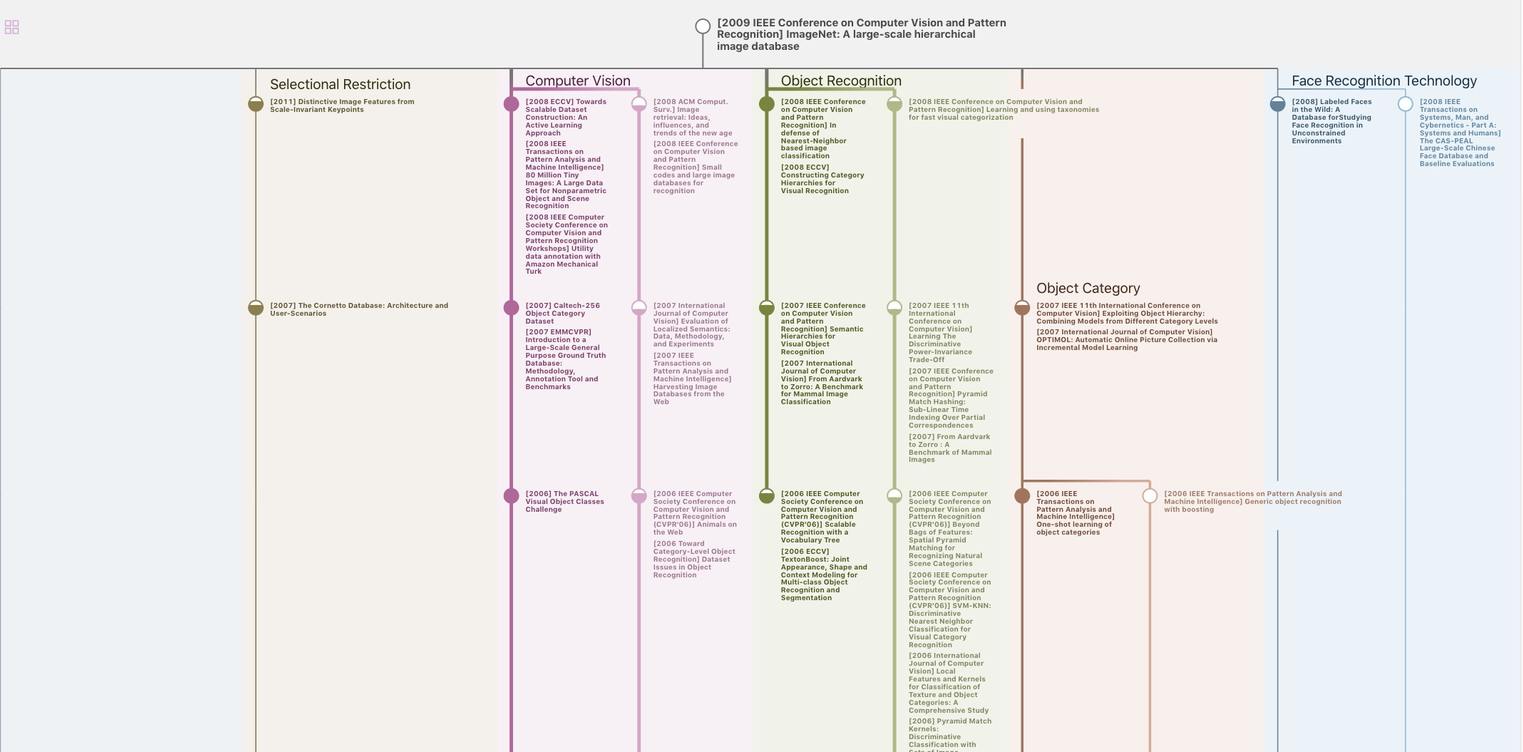
生成溯源树,研究论文发展脉络
Chat Paper
正在生成论文摘要