Forecasting Player Behavioral Data and Simulating In-Game Events
arXiv: Machine Learning(2018)
摘要
Understanding player behavior is fundamental in game data science. Video games evolve as players interact with the game, so being able to foresee player experience would help to ensure a successful game development. In particular, game developers need to evaluate beforehand the impact of in-game events. Simulation optimization of these events is crucial to increase player engagement and maximize monetization. We present an experimental analysis of several methods to forecast game-related variables, with two main aims: to obtain accurate predictions of in-app purchases and playtime in an operational production environment, and to perform simulations of in-game events in order to maximize sales and playtime. Our ultimate purpose is to take a step towards the data-driven development of games. The results suggest that even though the performance of traditional approaches, such as ARIMA is still better, the outcomes of state-of-the-art techniques like deep learning are promising. Deep learning comes up as a well-suited general model that could be used to forecast a variety of time series with different dynamic behaviors.
更多查看译文
关键词
Social games, Time series, Forecasting, Sequential analysis, Deep learning, ARIMA models, Gradient boosting
AI 理解论文
溯源树
样例
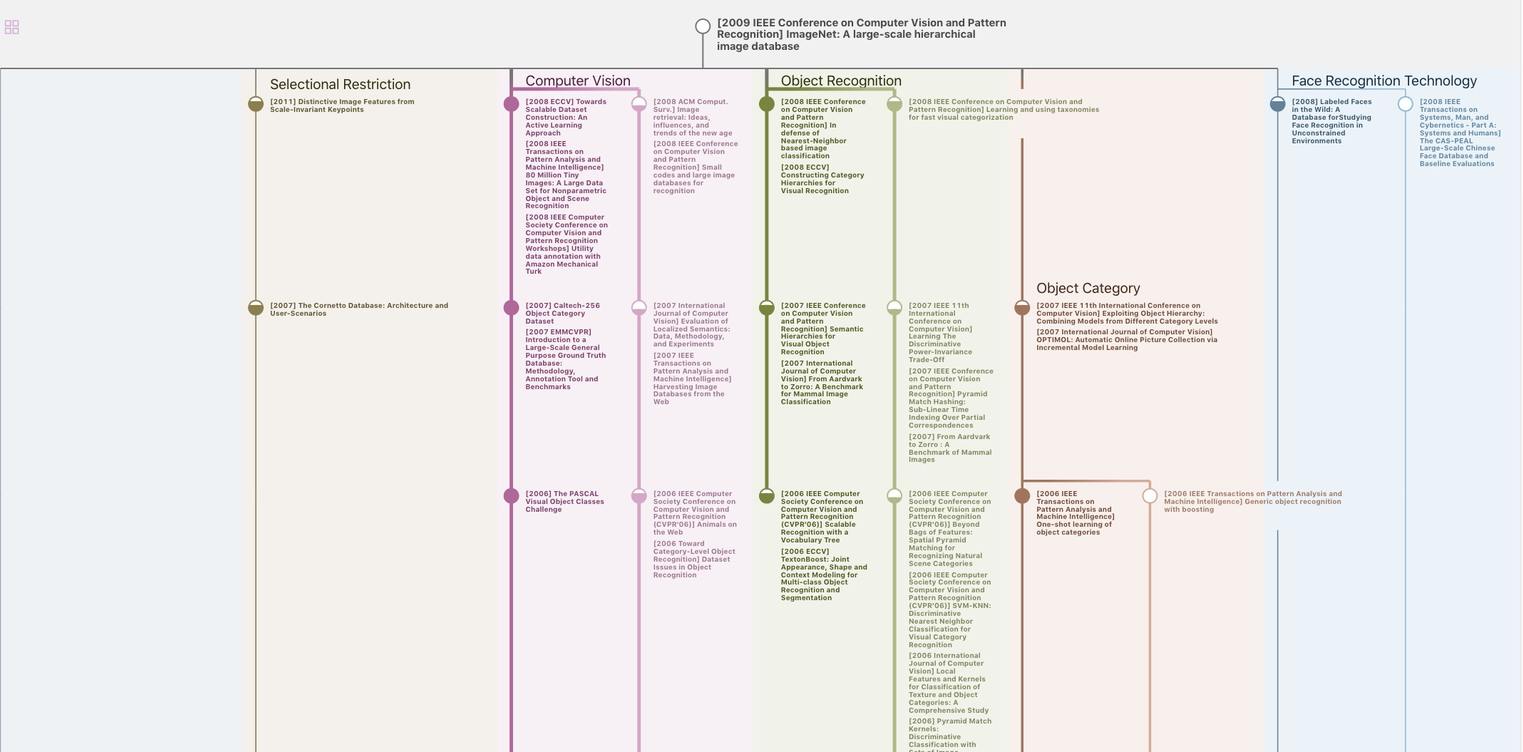
生成溯源树,研究论文发展脉络
Chat Paper
正在生成论文摘要