[P4–503]: IMPROVED PREDICTION OF PROGRESSION TO CLINICAL STAGES OF ALZHEIMER's DISEASE USING MULTIVARIATE SURFACE MORPHOMETRY OF MRI BIOMARKERS AND PATCH‐BASED SPARSE CODING
Alzheimers & Dementia(2017)
摘要
The best hope for slowing the progression of Alzheimer's disease (AD) will necessitate identifying sensitive biomarkers that precede and predict future symptomatic onset, i.e., amnestic Mild Cognitive Impairment (aMCI). We previously developed surface multivariate tensor-based morphometry (mTBM) and grey matter morphology signatures as potential structural MRI AD biomarkers to study hippocampal and lateral ventricular morphology. We subsequently developed patch-based sparse coding algorithms to successfully extract the most discriminating features from MR images for predicting cognitive decline. We now use these methods on a sample balanced for APOE gene dose. From a prospective cohort study, 18 initially cognitively unimpaired adults who subsequently progressed to the clinical diagnosis of aMCI or dementia due to AD within 1.8±0.8 years (progressors) were matched for age, sex, education, and APOE4 gene dose to 20 adults who remained cognitively unimpaired for at least 4 years after baseline visits (nonprogressors). Each baseline MRI scan was segmented with FSL (http://fsl.fmrib.ox.ac.uk/fsl/fslwiki), the hippocampal and ventricle surfaces parameterized, and the surface multivariate morphometry statistics (MMS) consisting of mTBM and radial distance (RD) generated. A collection of overlapping patches on the surface as the initial sparse coding dictionary was constructed. Stochastic Coordinate Coding was then applied to learn a dictionary and sparse codes. The max-pooling algorithm on the newly learned high-dimensional features was used to obtain a final set of low-dimensional features. Finally, an AdaBoost classifier was applied to categorize aMCI and cognitively unimpaired individuals with 5-fold cross validation adopted to evaluate classification accuracy, sensitivity, specificity, positive and negative predictive values. The prediction result of aMCI/AD was achieved with hippocampal surface MMS features, with 100% accuracy, 100% sensitivity, 100% specificity, 100% positive and 100% negative predictive values. This compares to 79% sensitivity and 78% specificity in the same sample based on standard automated brain mapping algorithmic programs to measure hippocampal volume, and assessed via binary logistic regression, and leave-one-out procedures. While our findings should be considered preliminary, sparse coding together with the surface multivariate morphometry may be applied to individual volumetric MRIs to predict imminent progression from the preclinical to clinical stages of AD with great accuracy.
更多查看译文
关键词
mri biomarkers,multivariate surface morphometry,alzheimers,sparse,patch-based
AI 理解论文
溯源树
样例
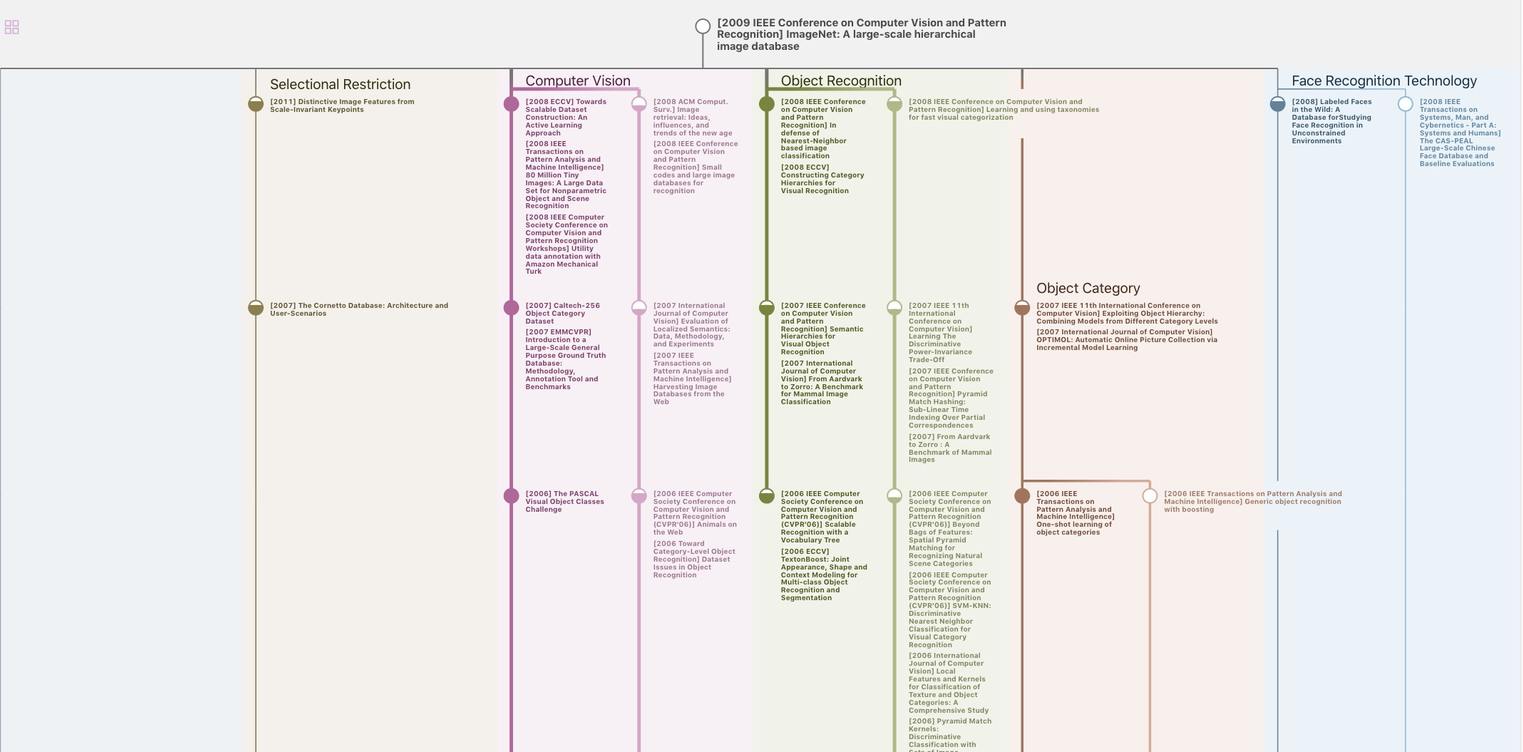
生成溯源树,研究论文发展脉络
Chat Paper
正在生成论文摘要