Abstract A188: Harnessing synthetic lethality to predict the response to cancer treatments
Molecular Cancer Therapeutics(2018)
摘要
Synthetic lethality (SL) describes an interaction between a pair of genes whereby their double knockout is lethal, while their respective knockout is not. The identification of SL interactions (SLi) via large-scale genomic screens offers promising opportunities for developing selective therapies in cancer. However, our analysis of the TCGA cohort shows that many of the interactions do not carry predictive signal of patient survival or drug response. Here we present a data-driven approach termed ISLE (Identification of clinically relevant Synthetic LEthality) that mines the TCGA cohort to identify a subset of clinically relevant SL interactions (cSLi). ISLE consists of the following inference steps, analysis of tumor, cell line, and gene evolutionary data. We first create an initial pool of SL pairs identified through direct double knockout screens/isogenic cell line screens or inferred from large-scale shRNA/sgRNA single-gene knockout screens. Starting from this initial SL pool, ISLE first identifies putative SL gene pairs whose co-inactivation is under-represented in tumors, testifying that it is selected against. Second, it prioritizes candidate SL pairs whose co-inactivation is associated with improved patient’s prognosis, testifying that it may hamper tumor progression. Finally, it prioritizes SL-gene pairs with similar evolutionary phylogenetic profiles based on the notion that SL interactions are conserved across multiple species. We validate the identified SL pairs using an unseen large-scale in vitro drug response screen by showing the SL pairs marks a decent prediction accuracy (AUC~0.8). We compare ISLE’s performance to the standard supervised drug response prediction approaches in DREAM challenges, and our prediction based on generic pretreatment tumor samples (from TCGA) was within top 3 in prediction accuracy among the top predictors. ISLE-based approach also successfully distinguishes responders vs nonresponders to drug treatment (for u003e70% of drugs) in mouse xenografts using the activity profile of the drug target’s SL-partners. We then experimentally show the utility of SL in predicting synergistic drug combinations in patient-derived cell lines based on the notion that the two drugs whose targets have SL interactions are synergistic. Most importantly, we demonstrate for the first time that an SL network can successfully predict the treatment outcome in cancer patients in multiple large-scale patient datasets including TCGA, where cSLi are successfully predict patients’ response for more than 70% of cancer drugs. ISLE is predictive of patients’ response for the majority of current cancer drugs without any drug-specific training. Of paramount importance, the predictions of ISLE are based on SLi between (potentially) all genes in the cancer genome, thus prioritizing treatments for patients whose tumors do not bear specific actionable mutations in cancer driver genes, offering a novel approach to precision-based cancer therapy. Citation Format: Joo S. Lee, Avinash Das, Livnat Jerby-Arnon, Rand Arafeh, Matthew Davidson, Arnaud Amzallag, Seung Gu Park, Kuoyuan Cheng, Welles Robinson, Dikla Atias, Chani Stossel, Ella Buzhor, Gidi Stein, Joshua J. Waterfall, Paul S. Meltzer, Talia Golan, Sridhar Hannenhalli, Eyal Gottlieb, Cyril H. Benes, Yardena Samuels, Emma Shanks, Eytan Ruppin. Harnessing synthetic lethality to predict the response to cancer treatments [abstract]. In: Proceedings of the AACR-NCI-EORTC International Conference: Molecular Targets and Cancer Therapeutics; 2017 Oct 26-30; Philadelphia, PA. Philadelphia (PA): AACR; Mol Cancer Ther 2018;17(1 Suppl):Abstract nr A188.
更多查看译文
AI 理解论文
溯源树
样例
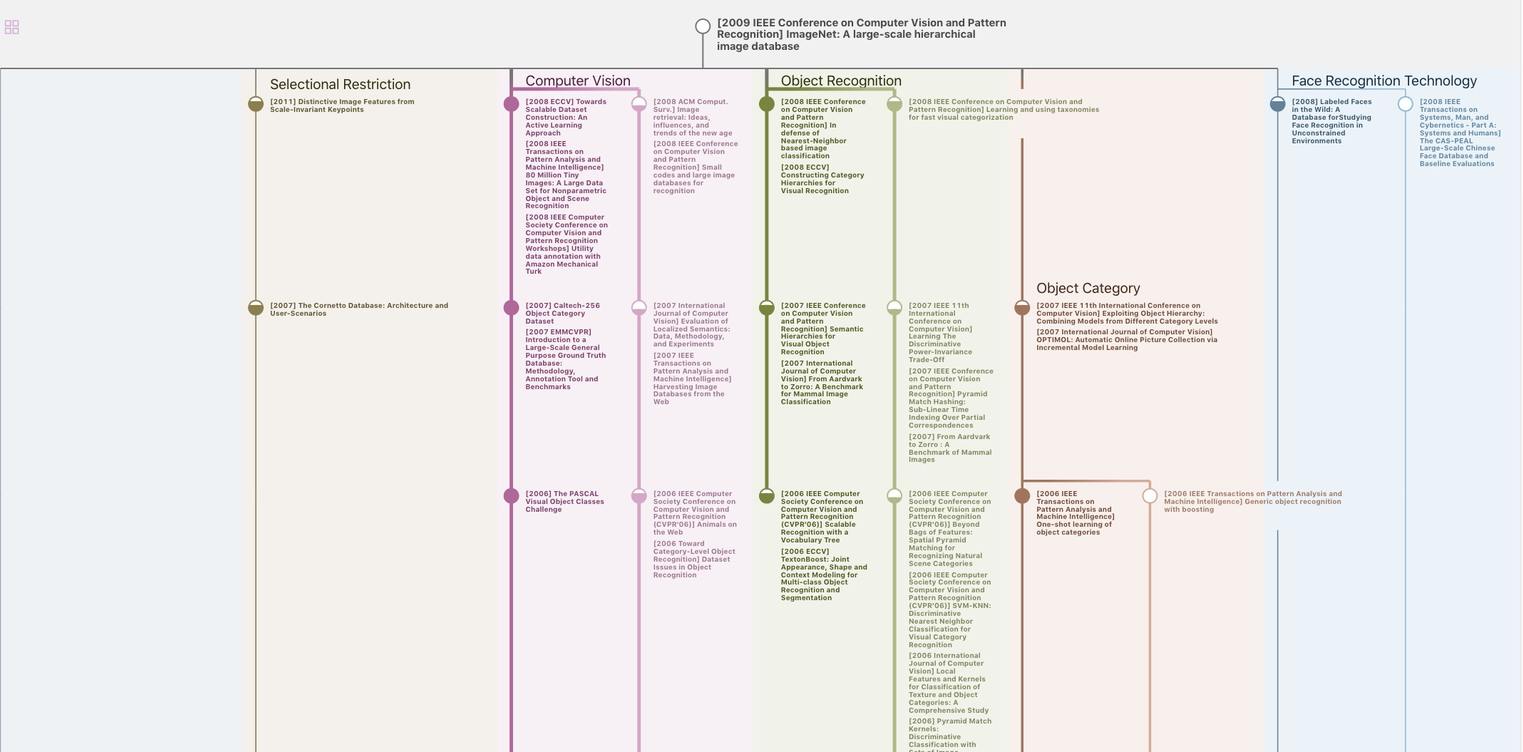
生成溯源树,研究论文发展脉络
Chat Paper
正在生成论文摘要