Gradient Direction Accumulation-Based Heat Kernel Signature Descriptor For Nonrigid 3d Model Retrieval
INTERNATIONAL JOURNAL OF PATTERN RECOGNITION AND ARTIFICIAL INTELLIGENCE(2019)
摘要
This paper presents an effective 3D local feature descriptor, which is called Gradient Direction Accumulation-based Heat Kernel Signature (GDA-HKS) descriptor, and its application in nonrigid 3D model retrieval. The GDA-HKS descriptor is based on the heat kernel signature, and it is scale invariant and robust to the nonrigid deformation of the 3D model. Compared with the SI-HKS descriptor, the GDA-HKS descriptor is constructed directly in the time domain, and it can effectively avoid the loss of high frequency information. The absolute gradient difference is used to encode the GDA-HKS descriptor, which can describe the changing trend of the one-dimensional signal more effectively. Extensive experimental results have validated the effectiveness of the designed GDA-HKS descriptor.
更多查看译文
关键词
Nonrigid 3D model retrieval, HKS, SI-HKS, GDA-HKS
AI 理解论文
溯源树
样例
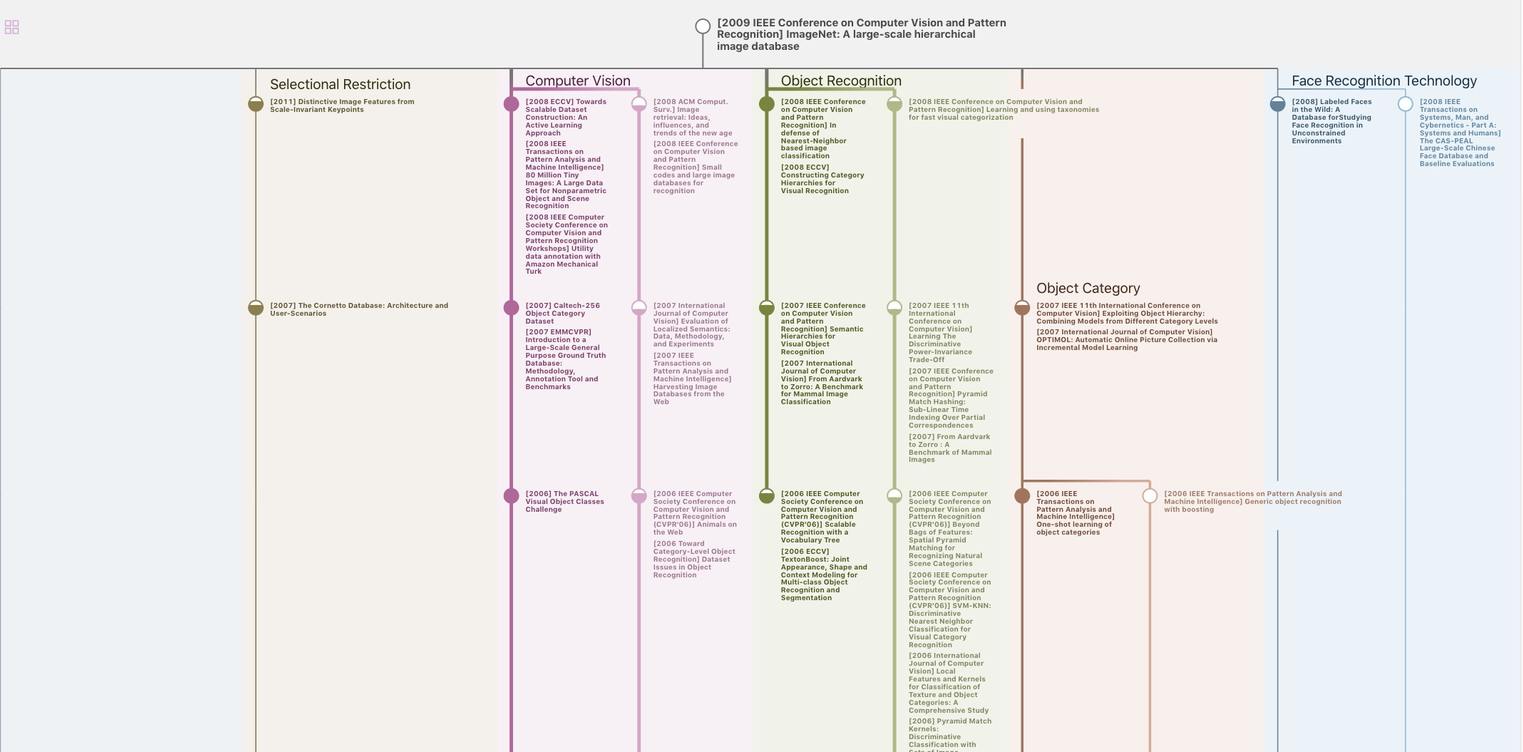
生成溯源树,研究论文发展脉络
Chat Paper
正在生成论文摘要