BUM: Bayesian User Model for Distributed Learning of User Characteristics from Heterogeneous Information
IEEE transactions on cognitive and developmental systems(2019)
摘要
This paper presents a Bayesian user model able to learn and estimate user characteristics in a distributed manner using heterogeneous information. A unified user representation is obtained from an inference process, receiving a set of independently estimated user characteristics from different sources. The independence of characteristic models enables the system to be modular, with each module estimating one characteristic. The proposed model is iterative, fusing new observations, and measurements with previous information in a process regulated entropy. The system allows diverse implementations, such as the combination of multiple robots with a cloud infrastructure or distributed ambient sensors. This paper aims to enable the system to perform online learning while interacting with users. The system is also able to obtain a correct user representation from heterogeneous information, even when some user characteristics cannot be computed. To demonstrate its functionality, the system is tested on two experimental datasets, obtained from simulated experiments and with real users. This technique advances the state of the art in the areas of AAL and user-adaptive systems, and in cloud-connected robots and Internet of Things, allowing for these heterogeneous and naturally distributed teams of devices to better model their users, potentially achieving higher interaction autonomy.
更多查看译文
关键词
Internet of Things (IoT),multimodal human-robot interaction,robot perception,social robots,user modeling,user profiling,user-adaptive interaction
AI 理解论文
溯源树
样例
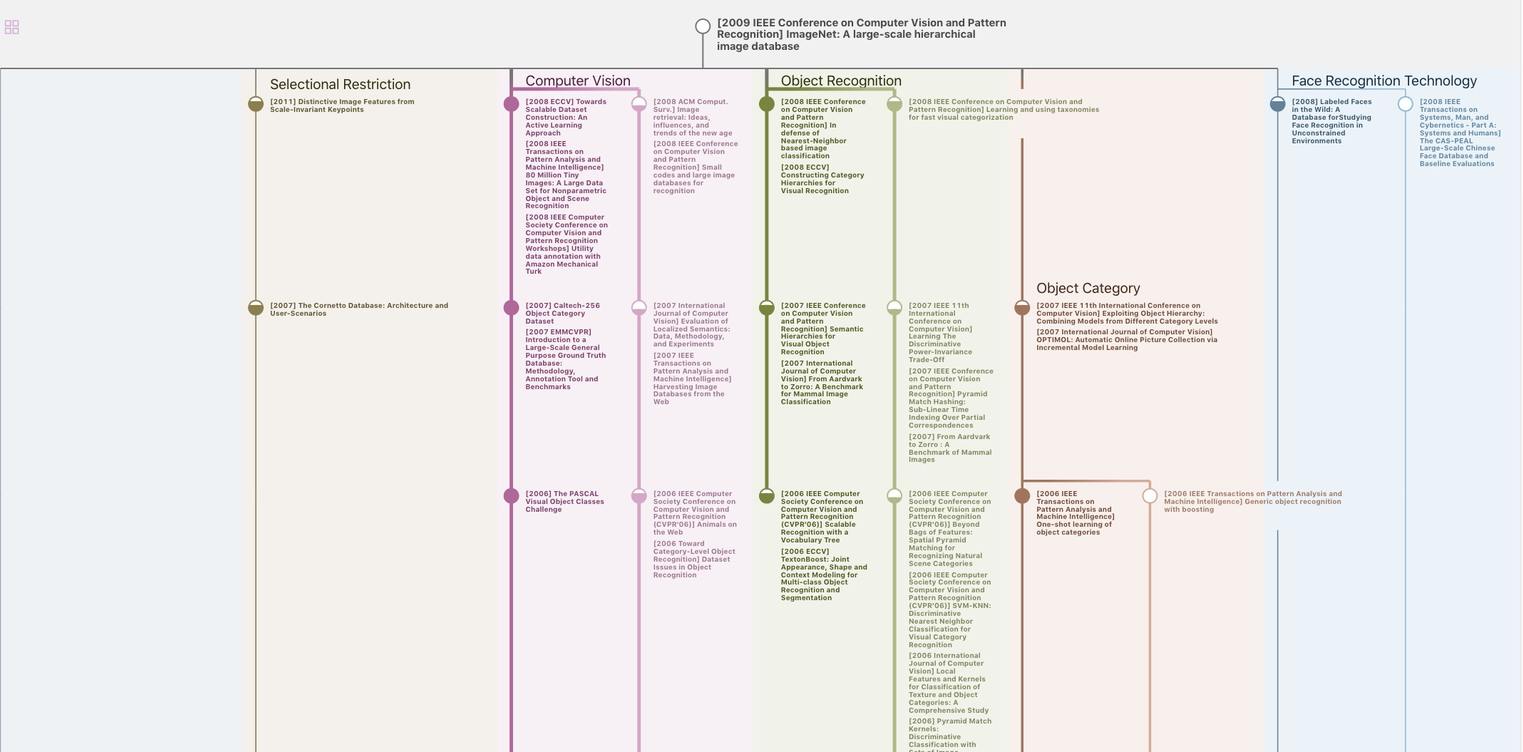
生成溯源树,研究论文发展脉络
Chat Paper
正在生成论文摘要