Classification of Mild Cognitive Impairment Stages Using Machine Learning Methods
2018 IEEE 22nd International Conference on Intelligent Engineering Systems (INES)(2018)
摘要
The elderly population in developed countries has augmented in the last decades. This has also entailed an increased prevalence of aging diseases. Mild Cognitive Impairment (MCI) is considered a prodromal stage of Alzheimer's Disease (AD), which is the most common dementia. We present a benchmarking of Machine Learning (ML) methods for MCI staging and its early detection via the multiclass classification of Cognitively Normal (CN), Early Mild Cognitive Impairment (EMCI) and Late Mild Cognitive Impairment (LMCI) subjects by means of neuropsychological scales. Data were obtained from Alzheimer's Disease Neuroimaging Initiative (ADNI). Our study has been aimed towards hybrid neural architectures, such as the Counter-propagation Network (CPN), for their input space quantification capability, and non-neural methods including ensembles. We also analyzed how class balancing affected them. Non-neural ensembles of learners obtained Area Under the Curve (AUC) values in the range 0.721-0.775, whereas 0.650-0.657 with the monolithic architecture CPN. This suggests that there is a weak difference among these cognitive stages, that the used scales do not offer high enough discriminant power, and that neural ensembles can be a more appropriate solution.
更多查看译文
关键词
aging diseases,prodromal stage,MCI staging,multiclass classification,nonneural methods,nonneural ensembles,machine learning methods,ML methods,mild cognitive impairment stages,cognitively normal,Alzheimers disease neuroimaging initiative,late mild cognitive impairment,neuropsychological scales,hybrid neural architectures,counter-propagation network,CPN,input space quantification capability,area under the curve,AUC,monolithic architecture,CN,EMCI,LMCI
AI 理解论文
溯源树
样例
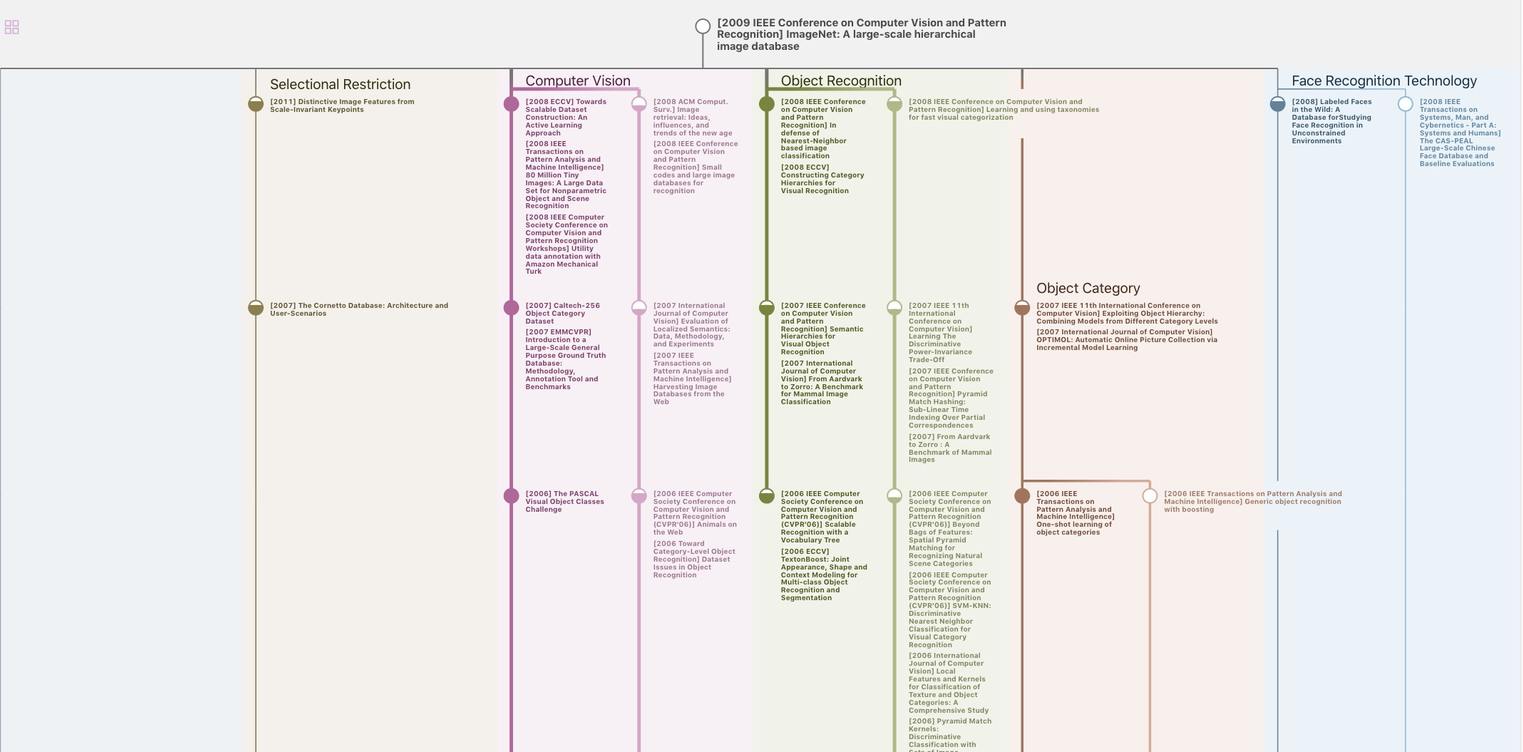
生成溯源树,研究论文发展脉络
Chat Paper
正在生成论文摘要