Deep-learning based hologram classification for molecular diagnostics
bioRxiv(2018)
摘要
Lens-free digital in-line holography (LDIH) is a promising microscopic tool that can overcome the limitations (e.g., field of view) of traditional lens-based microcopy. Images produced by LDIH, however, require extensive computation time to reconstruct objet images from complex diffraction patterns, which limits LDIH utility for point-of-care applications, particularly in resource limited settings. Here, we describe a new deep-learning (DL) based approach to process LDIH images in the context of cellular analyses. Specifically, we captured hologram images of cells surface-labeled with molecular-specific microbeads, and trained neural networks to classify these holograms without reconstruction. Using raw holograms as input, the trained networks could group individual cells according to the number of beads attached. Particularly, VGG-19 pretrained networks showed robust performance even with noisy experimental data. Combined with the developed DL approach, LDIH could be realized as low-cost, portable tool for point-of-care diagnostics.
更多查看译文
AI 理解论文
溯源树
样例
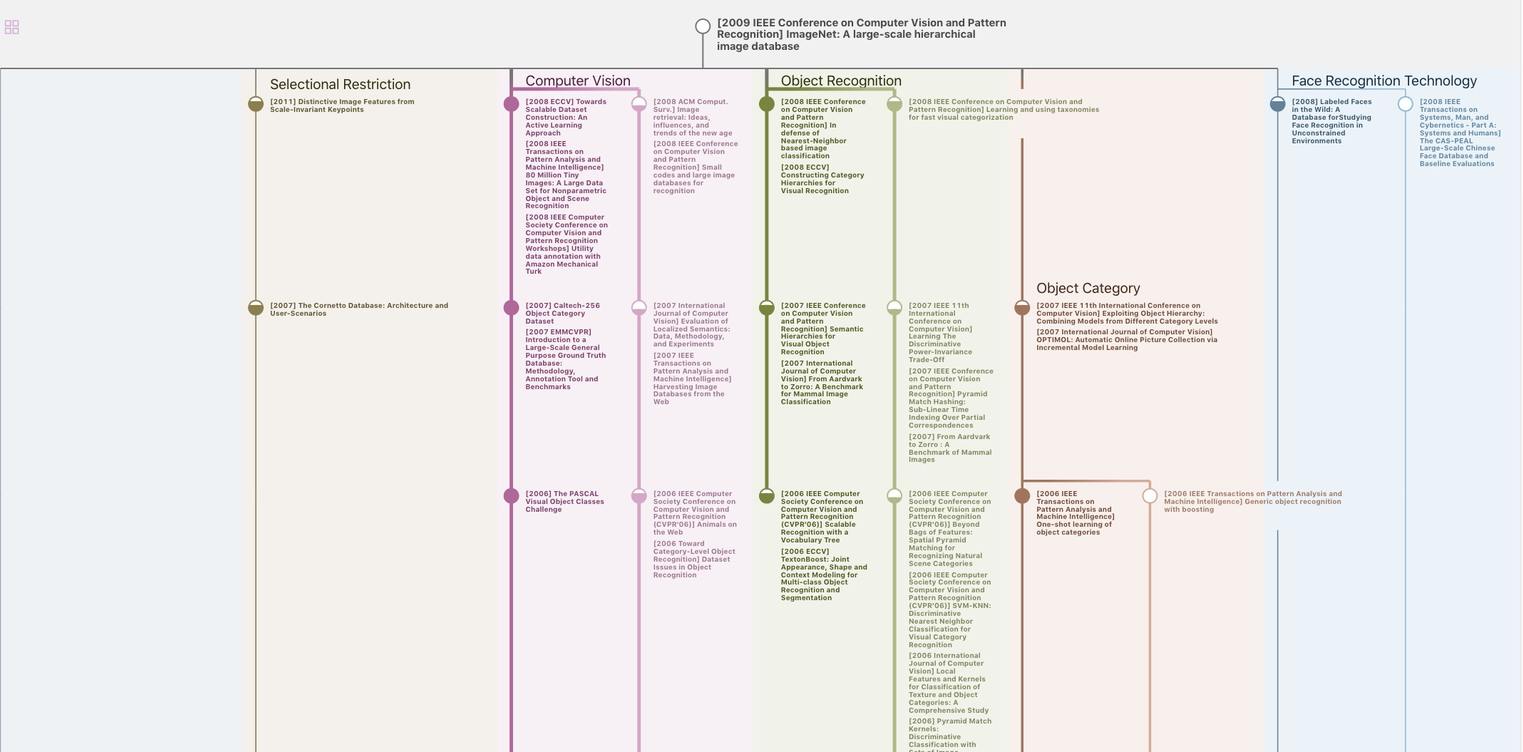
生成溯源树,研究论文发展脉络
Chat Paper
正在生成论文摘要